Evaluation of Seasonally Classified Inputs for the Prediction of Daily Groundwater Levels: NARX Networks Vs Support Vector Machines
Environmental Modeling & Assessment(2018)
摘要
Farmers and stakeholders who use groundwater for irrigation need efficient and cost-effective tools to support sustainable crop production. The variation of groundwater levels at local scale and its continuous use for agriculture intensifies the demand for reliable groundwater information. However, groundwater levels are very dynamic and difficult to predict under traditional modeling approaches, and manual monitoring of wells is costly and time-consuming. Simplified but powerful machine learning models represent a practical alternative to support groundwater management decisions at the farm scale. The predictive capacity of a nonlinear autoregressive with exogenous inputs (NARX) artificial neural network (ANN) and a support vector regression (SVR) trained with a radial basis function (RBF) algorithm were evaluated for an irrigation well located in a highly productive agricultural region in the southeastern USA. We used separately multiple years of daily historical time series classified by summer (withdrawal) and winter (recharge) seasons and evaluated the impacts of this division in the models’ predictive capability. Results showed that SVR had a better modeling performance based on the mean squared error (MSE) and prediction trend for both seasons. In addition, our study suggests that the prediction of daily levels with input time series classified by seasons provides higher accuracy than using the entire withdrawal and recharge periods as a whole. Results also indicate that the recharge season becomes a linear problem, which substantially reduces the SVR modeling computational requirements. The application of our proposed modeling approach in the management of groundwater sources for irrigation provides important information, at short time scale, for the estimation of groundwater variability at local scale.
更多查看译文
关键词
Groundwater levels, Artificial neural networks (ANN), Support vector machine (SVM), Water management, Groundwater irrigation
AI 理解论文
溯源树
样例
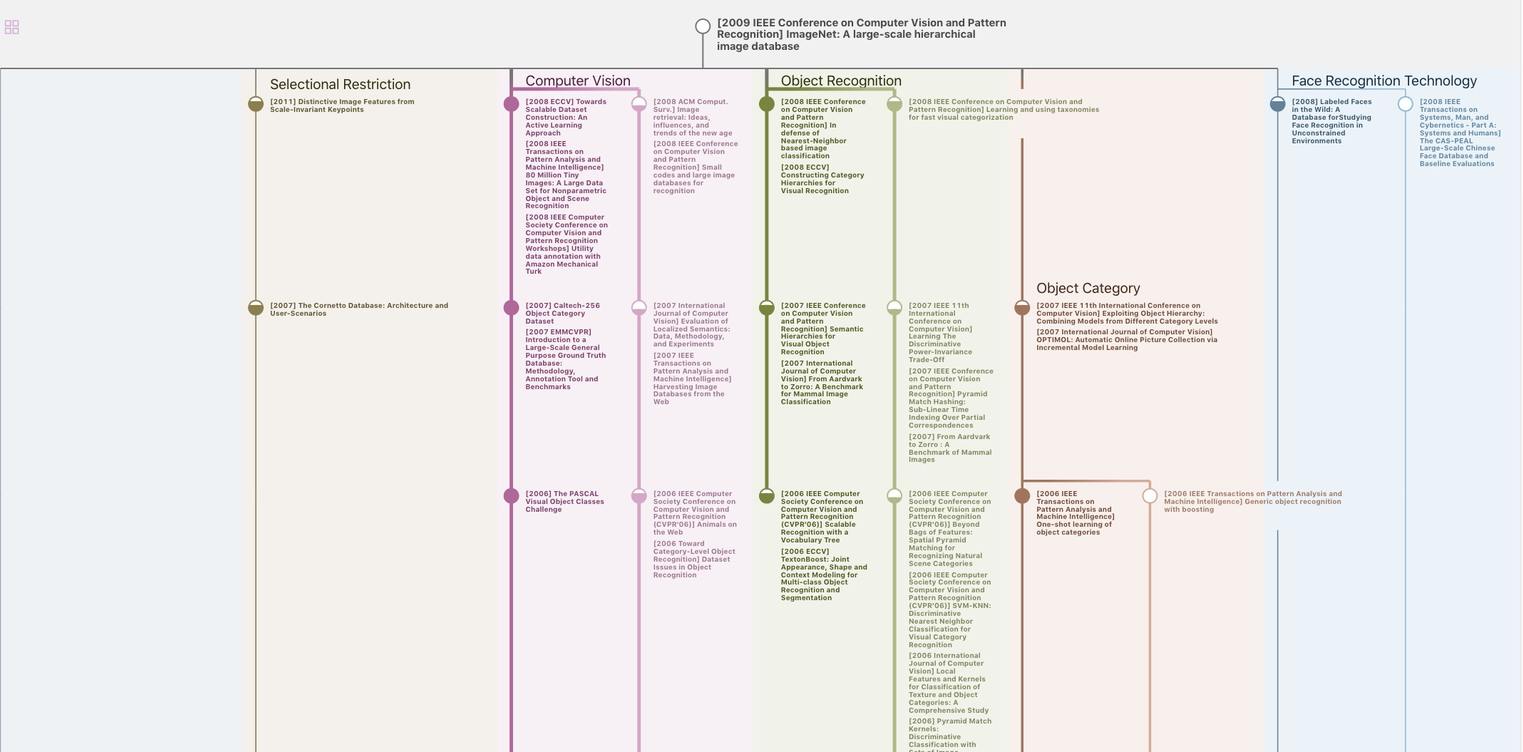
生成溯源树,研究论文发展脉络
Chat Paper
正在生成论文摘要