Enhancing The Dissfcm Algorithm For Data Stream Classification
FUZZY LOGIC AND APPLICATIONS, WILF 2018(2019)
摘要
Analyzing data streams has become a new challenge to meet the demands of real time analytics. Conventional mining techniques are proving inefficient to cope with challenges associated with data streams, including resources constraints like memory and running time along with single scan of the data. Most existing data stream classification methods require labeled samples that are more difficult and expensive to obtain than unlabeled ones. Semi-supervised learning algorithms can solve this problem by using unlabeled samples together with a few labeled ones to build classification models. Recently we proposed DISSFCM, an algorithm for data stream classification based on incremental semi-supervised fuzzy clustering. To cope with the evolution of data, DISSFCM adapts dynamically the number of clusters by splitting large-scale clusters. While splitting is effective in improving the quality of clusters, a repeated application without counter-balance may induce many small-scale clusters. To solve this problem, in this paper we enhance DISSFCM by introducing a procedure that merges small-scale clusters. Preliminary experimental results on a real-world benchmark dataset show the effectiveness of the method.
更多查看译文
关键词
Data stream classification, Semi-supervised fuzzy clustering, Incremental adaptive clustering
AI 理解论文
溯源树
样例
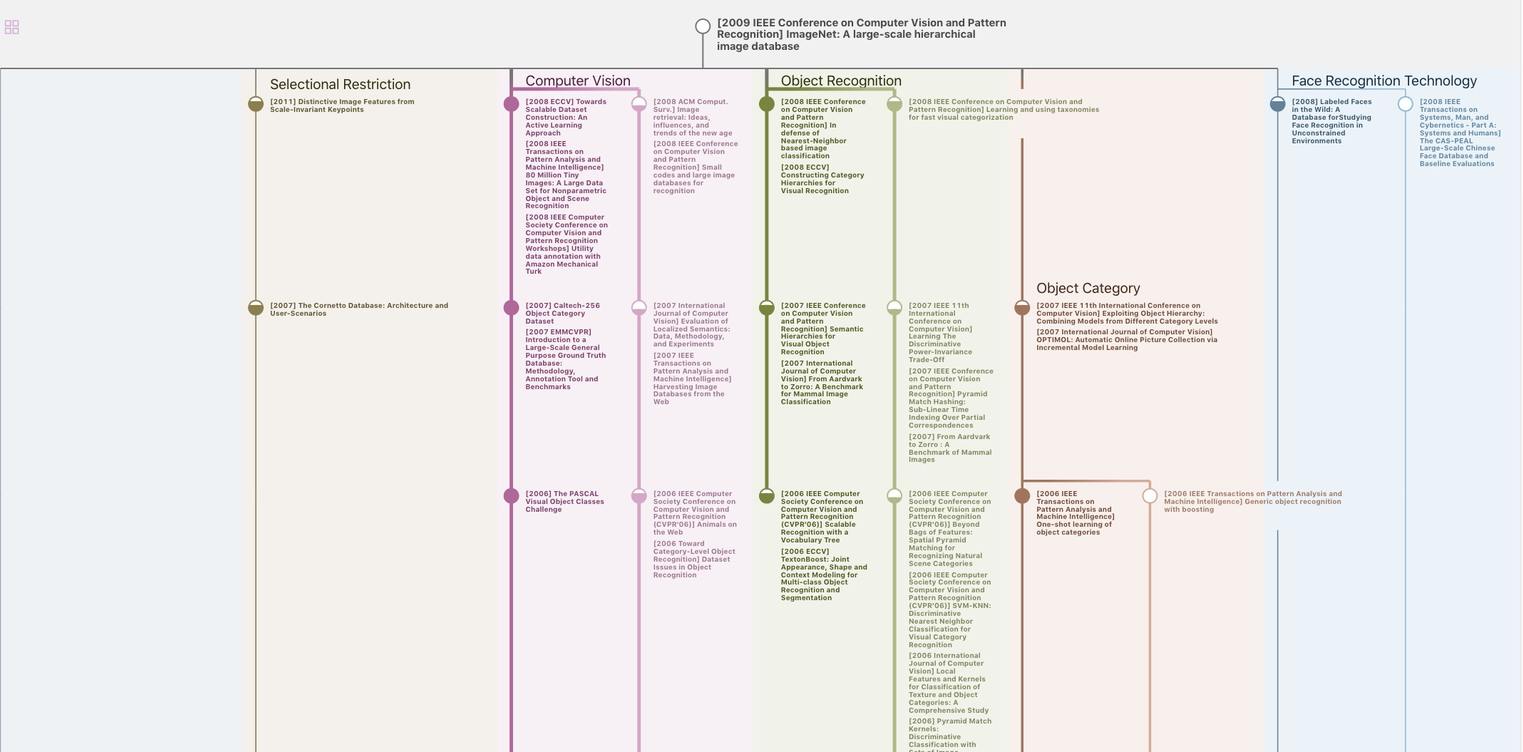
生成溯源树,研究论文发展脉络
Chat Paper
正在生成论文摘要