APPCLASSIFIER - Automated App Inference on Encrypted Traffic via Meta Data Analysis.
IEEE Global Communications Conference(2018)
摘要
As smart phones gradually become the dominant network traffic generators, app traffic analysis methods have gained great interests for network management and targeted advertisement. Specifically, previous works have shown that the scalability of app inference via traffic meta-data has the edge over traditional payload based analysis. However, such works mainly considered the ideal inference scenario where only one app is running on the client's device, without any background traffic noise interfered. In this paper, we extend the research to a more practical scenario, by assuming that multiple apps simultaneously run on a smart phone in the noisy background with complex traffic generated by the operating system. To that end, we propose APPCLASSIFIER, an Android app fingerprinting scheme for real-time app inference. We first leverage the observed differences in packet size distributions and traffic sequential behaviors to boost inference accuracy for noise-free traffic analysis. We then propose novel heuristic based methods to re-correct mislabeled traffic flows to realize real-time traffic inference. As a result, APPCLASSIFIER achieves inference accuracy of 823% for noise-free traffic analysis and reduces error rate from 66.7% to 36.4% for real-time traffic inference.
更多查看译文
关键词
re-correct mislabeled traffic,real-time traffic inference,APPCLASSIFIER,inference accuracy,noise-free traffic analysis,automated app inference,encrypted traffic,meta data analysis,smart phone,dominant network traffic generators,app traffic analysis methods,network management,traffic meta-data,ideal inference scenario,background traffic noise,complex traffic,Android app fingerprinting scheme,real-time app inference,traffic sequential behaviors,heuristic based methods
AI 理解论文
溯源树
样例
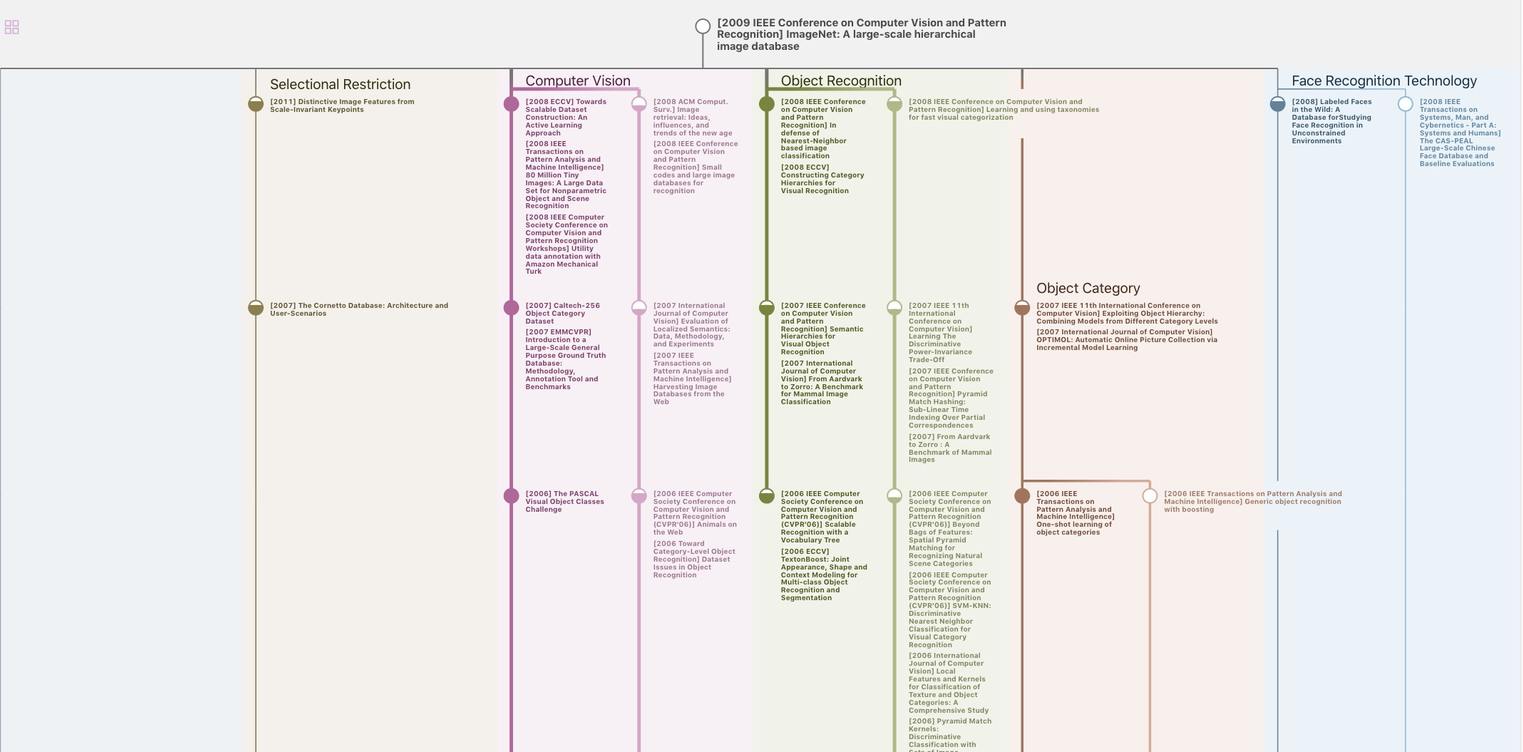
生成溯源树,研究论文发展脉络
Chat Paper
正在生成论文摘要