Improving Word Embedding Coverage in Less-Resourced Languages Through Multi-Linguality and Cross-Linguality: A Case Study with Aspect-Based Sentiment Analysis.
ACM Trans. Asian & Low-Resource Lang. Inf. Process.(2019)
摘要
In the era of deep learning-based systems, efficient input representation is one of the primary requisites in solving various problems related to Natural Language Processing (NLP), data mining, text mining, and the like. Absence of adequate representation for an input introduces the problem of data sparsity, and it poses a great challenge to solve the underlying problem. The problem is more intensified with resource-poor languages due to the absence of a sufficiently large corpus required to train a word embedding model. In this work, we propose an effective method to improve the word embedding coverage in less-resourced languages by leveraging bilingual word embeddings learned from different corpora. We train and evaluate deep Long Short Term Memory (LSTM)-based architecture and show the effectiveness of the proposed approach for two aspect-level sentiment analysis tasks (i.e., aspect term extraction and sentiment classification). The neural network architecture is further assisted by hand-crafted features for prediction. We apply the proposed model in two experimental setups: multi-lingual and cross-lingual. Experimental results show the effectiveness of the proposed approach against the state-of-the-art methods.
更多查看译文
关键词
Aspect-Based Sentiment Analysis (ABSA), Indian languages, Long Short Term Memory (LSTM), Sentiment analysis, bilingual word embeddings, cross-lingual sentiment analysis, data sparsity, deep learning, low-resourced languages
AI 理解论文
溯源树
样例
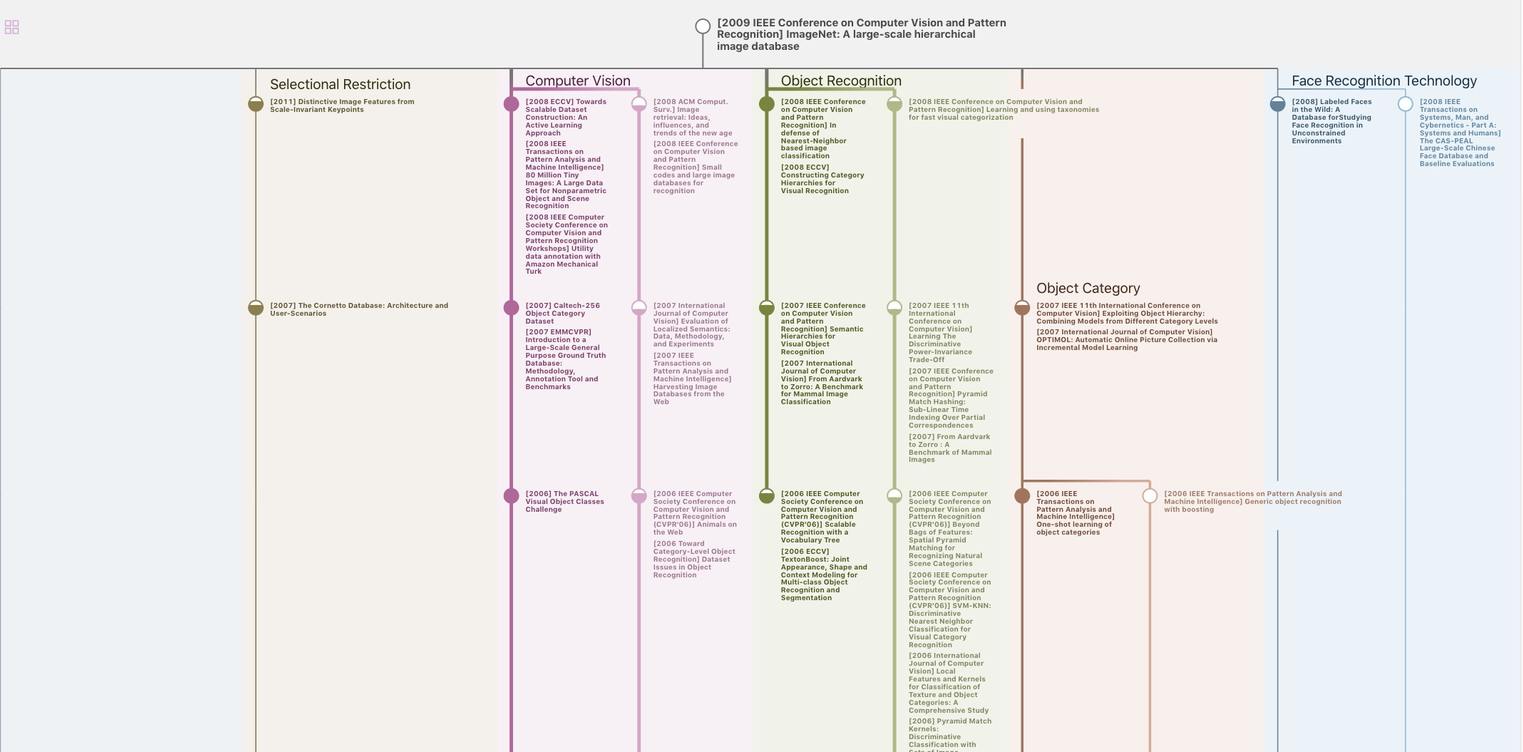
生成溯源树,研究论文发展脉络
Chat Paper
正在生成论文摘要