Unsupervised Learning-Based Long-Term Superpixel Tracking
IMAGE AND VISION COMPUTING(2019)
摘要
Finding correspondences between structural entities decomposing images is of high interest for computer vision applications. In particular, we analyze how to accurately track superpixels - visual primitives generated by aggregating adjacent pixels sharing similar characteristics - over extended time periods relying on unsupervised learning and temporal integration. A two-step video processing pipeline dedicated to long-term superpixel tracking is proposed. First, unsupervised learning-based superpixel matching provides correspondences between consecutive and distant frames using new context-rich features extended from greyscale to multi-channel and forward-backward consistency constraints. Resulting elementary matches are then combined along multi-step paths running through the whole sequence with various inter-frame distances. This produces a large set of candidate long-term superpixel pairings upon which majority voting is performed. Video object tracking experiments demonstrate the accuracy of our elementary estimator against state-of-the-art methods and proves the ability of multi-step integration to provide accurate long-term superpixel matches compared to usual direct and sequential integration. (C) 2019 Elsevier B.V. All rights reserved.
更多查看译文
关键词
Superpixel matching,Unsupervised learning,Superpixel tracking,Multi-step integration,Random forests,Forward-backward consistency
AI 理解论文
溯源树
样例
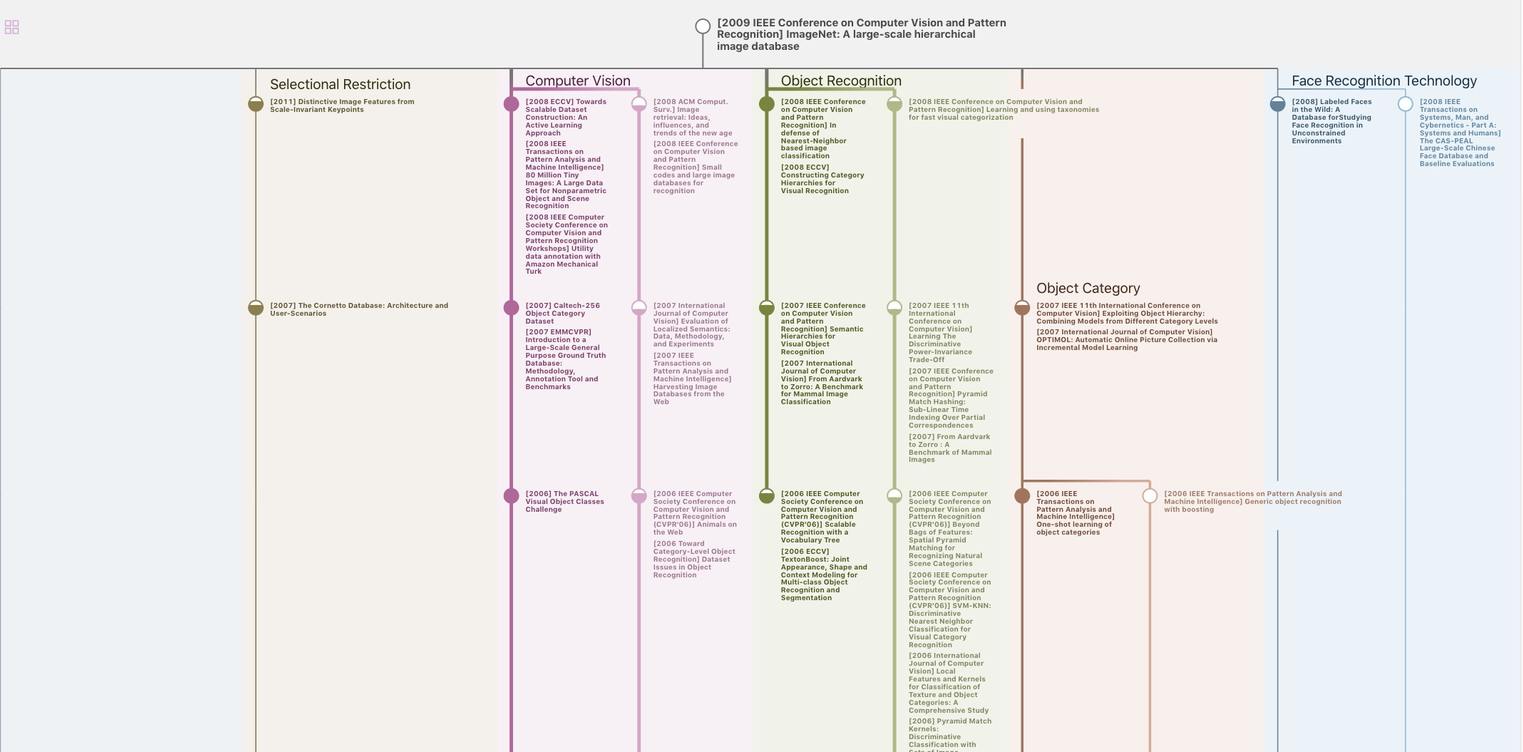
生成溯源树,研究论文发展脉络
Chat Paper
正在生成论文摘要