Rao-Blackwellized Particle Filters for Recognizing Activities and Spatial Context from Wearable Sensors
ISER(2008)
摘要
The focus of our work is on providing accurate information about a per-son's activities and environmental context in everyday environments based on wearable sensors and GPS devices. More specifically, we wish to estimate a person's motion type (such as walking, running, going upstairs/downstairs, or driving a vehicle) and whether a person is outdoors, inside a building, or in a vehicle. These activity estimates are combined with GPS information so as to estimate the trajectory of the person along with information about which buildings the person enters. To do this, our approach assumes that the bounding boxes of buildings are known (extracted from satellite images). Another emphasis of our work is on performing activity recognition based on a minimum number of sensor devices. There are in fact a variety of systems that utilize multiple sensors and measurements taken all over the body [5, 9]. Our approach, by contrast, attempts to produce as accurate as possible activity recognition requiring only one sensing device mounted only at one location on the body. Our reasoning for reducing the total number of sensors is threefold: 1) it can be unwieldy for the person wearing the sensors to have many such sensors and battery packs mounted all over the body, 2) we wish to minimize overall system cost, and 3) we wish to extend operational time between battery replacement/recharge. In this paper, we show how Rao-Blackwellized particle filters can be ap-plied to e ciently estimate joint posteriors over a person's activity and spatial context. Extensive experiments demonstrate that, by performing such joint inference, our system is able to generate more consistent estimates for a per-
更多查看译文
关键词
activity recognition,consistent estimator,spatial context
AI 理解论文
溯源树
样例
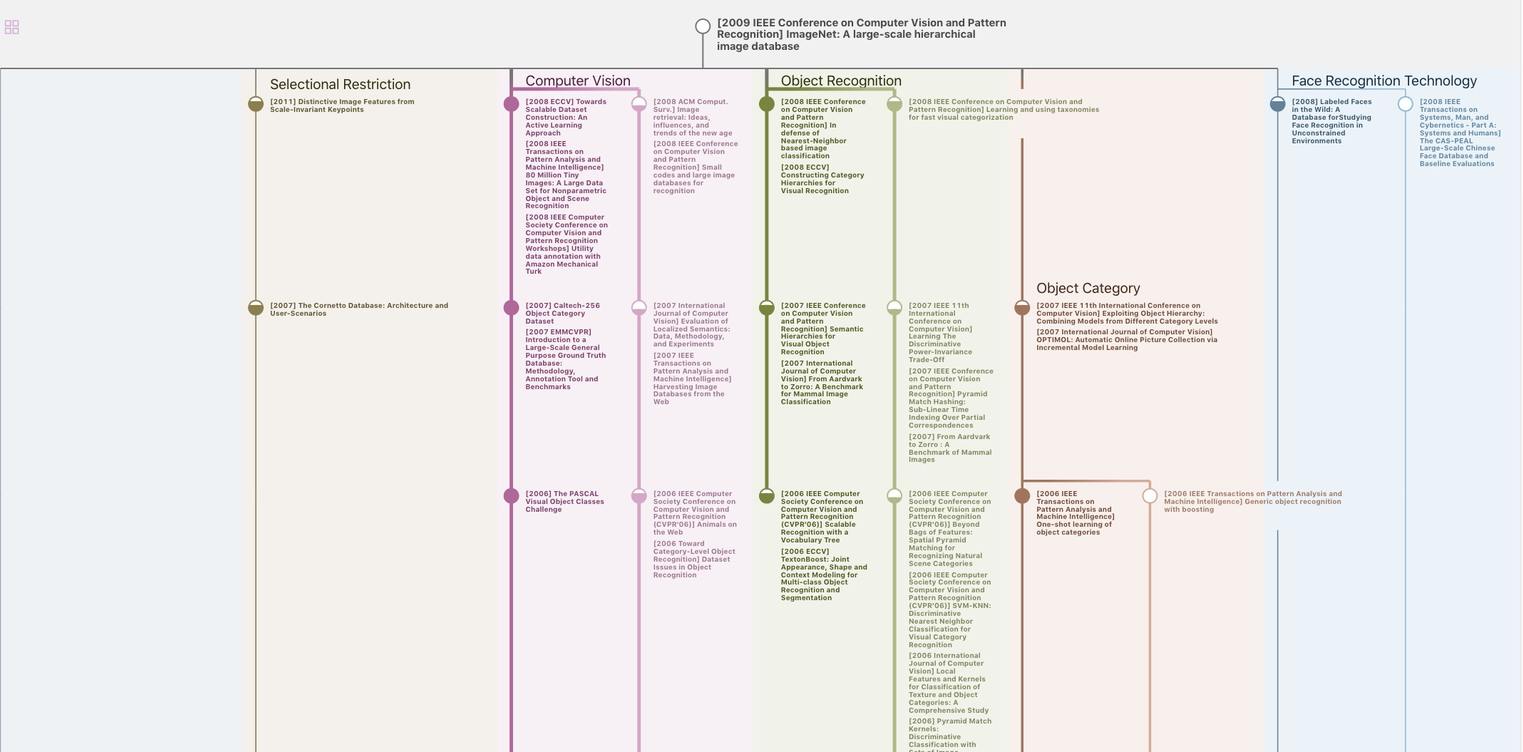
生成溯源树,研究论文发展脉络
Chat Paper
正在生成论文摘要