A segmentation method combining probability map and boundary based on multiple fully convolution network and repetitive training.
PHYSICS IN MEDICINE AND BIOLOGY(2019)
摘要
Cell nuclei image segmentation technology can help researchers observe each cell's stress response to drug treatment. However, it is still a challenge to accurately segment the adherent cell nuclei. At present, image segmentation based on a fully convolutional network (FCN) is attracting researchers' attention. We propose a multiple FCN architecture and repetitive training (M-FCN-RT) method to learn features of cell nucleus images. In M-FCN-RT, the multiple FCN (M-FCN) architecture is composed of several single FCNs (S-FCNs) with the same structure, and each FCN is used to learn the specific features of image datasets. In this paper, the M-FCN contains three FCNs; FCN1-2, FCN3 and FCNB. FCN1-2 and FCN3 are respectively used to learn the spatial features of cell nuclei for generating probability maps to indicate nucleus regions of an image; FCNB (boundary FCN) is used to learn the edge features of cell nuclei for generating the nucleus boundary. For the training of each FCN, we propose a repetitive training (RT) method to improve the classification accuracy of the model. To segment cell nuclei, we finally propose an algorithm combining the probability map and boundary (PMB) to segment the adherent nuclei. This paper uses a public opening nucleus image dataset to train, verify and evaluate the proposed M-FCN-RT and PMB methods. Our M-FCN-RT method achieves a high Dice similarity coefficient (DSC) of 92.11%, 95.64% and 87.99% on the three types of sub-datasets respectively for probability maps. In addition, segmentation experimental results show the PMB method is more effective and efficient compared with other methods.
更多查看译文
关键词
cell nuclei segmentation,fully convolutional network,multiple architecture,repetitive training
AI 理解论文
溯源树
样例
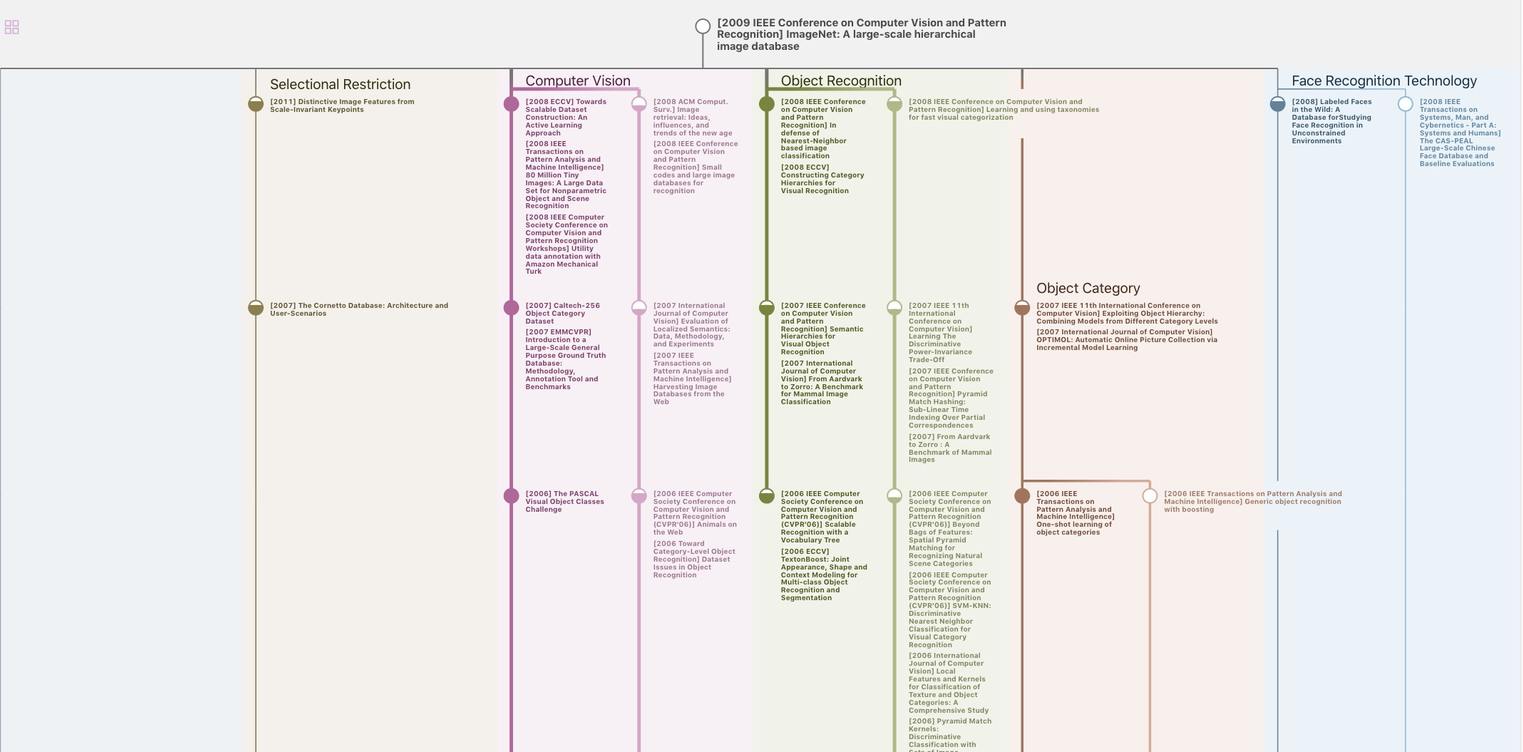
生成溯源树,研究论文发展脉络
Chat Paper
正在生成论文摘要