Image Completion With Discriminator Guided Context Encoder
2018 CONFERENCE RECORD OF 52ND ASILOMAR CONFERENCE ON SIGNALS, SYSTEMS, AND COMPUTERS(2018)
摘要
Image completion or inpainting is a technique that is used for reconstruction of damaged or distorted regions in an image. In this paper, a new convolutional neural network model is presented for image completion. The proposed method is based on an auto-encoder and a Generative Adversarial Network structure. To succeed at this task and produce a plausible output for the damaged or distorted region(s), the auto-encoder part of the network needs to understand the content of the entire image. On the other hand, discriminators that are used in the proposed network are responsible for deciding whether or not the inpainted output has the expected quality. The general discriminator looks at the entire image to evaluate if it is consistent as a whole, while the local discriminator looks only at the completed region to ensure the local consistency of the generated patches. The image completion network is then trained so that the discriminator networks conclude that the inpainted image is as real as the original. This approach is also aimed to reconstruct images regardless of where the damaged regions are. We compared our proposed method with two other approaches, and the results show that it performs better especially on images with low-texture.
更多查看译文
关键词
Image completion, inpainting, context encoder, discriminator
AI 理解论文
溯源树
样例
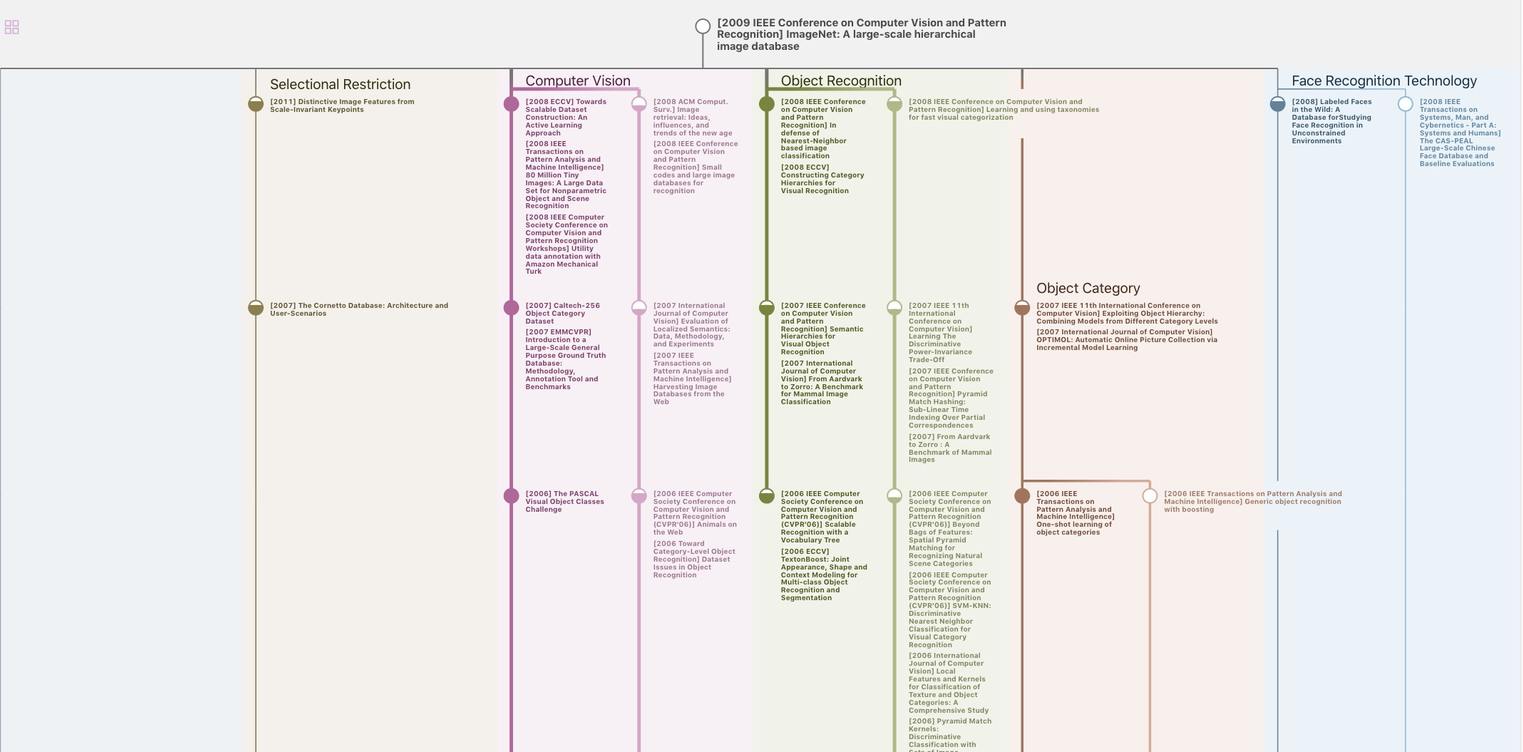
生成溯源树,研究论文发展脉络
Chat Paper
正在生成论文摘要