Real-Time Vehicle Make and Model Recognition with the Residual SqueezeNet Architecture.
SENSORS(2019)
摘要
Make and model recognition (MMR) of vehicles plays an important role in automatic vision-based systems. This paper proposes a novel deep learning approach for MMR using the SqueezeNet architecture. The frontal views of vehicle images are first extracted and fed into a deep network for training and testing. The SqueezeNet architecture with bypass connections between the Fire modules, a variant of the vanilla SqueezeNet, is employed for this study, which makes our MMR system more efficient. The experimental results on our collected large-scale vehicle datasets indicate that the proposed model achieves 96.3% recognition rate at the rank-1 level with an economical time slice of 108.8 ms. For inference tasks, the deployed deep model requires less than 5 MB of space and thus has a great viability in real-time applications.
更多查看译文
关键词
vehicle make recognition,deep learning,residual SqueezeNet
AI 理解论文
溯源树
样例
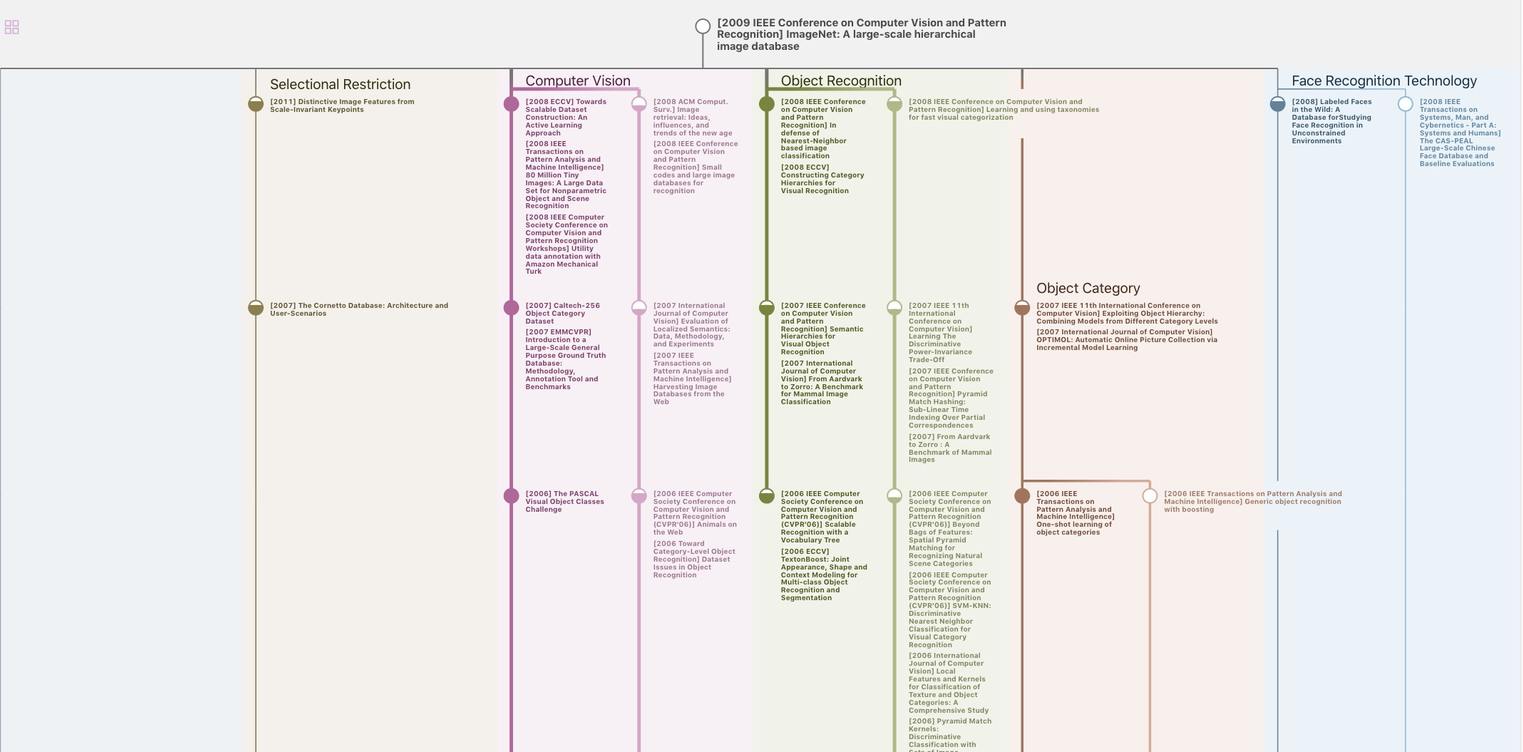
生成溯源树,研究论文发展脉络
Chat Paper
正在生成论文摘要