Probabilistic estimation of short sequence expression using RNA-Seq data and the positional bootstrap
bioRxiv(2016)
摘要
When estimating expression of a transcript or part of a transcript using RNA-Seq data, it is commonly assumed that reads are generated uniformly from positions within the transcript. While this assumption is acceptable for long transcript sequences, it frequently leads to large errors for short sequences, e.g., less than 100 bp.Analysis of short sequences, such as splice junctions adjacent to alternatively spliced axons and microRNAs, is increasingly importantand necessitates addressing errors in short-sequence expression estimation. Indeed, when we examined RNA-Seq data from diverse studies, we found that large errors are introduced by variations RNA-Seq coverage due to sequence content, experimental conditions and sample preparation.We developed a technique that we call the positional bootstrap, which quantifies the level of uncertainty in expression induced by non-uniform coverage. Unlike methods that attempt to correct for biases in coverage, but do so by making strong assumptions about the form of those biases, the positional bootstrap can quantify the noise induced by all types of bias, including unknown ones. Results obtained using independently generated RNA-Seq datasets show that the positional bootstrap increases the accuracy of estimates of alternative splicing levels, tissue-differential alternative splicing and tissue differentialexpression, by a factor of up to 10.An efficient Python implementation of the algorithm is freely available from github.com/PSI-Lab/BENTO-Seq.
更多查看译文
AI 理解论文
溯源树
样例
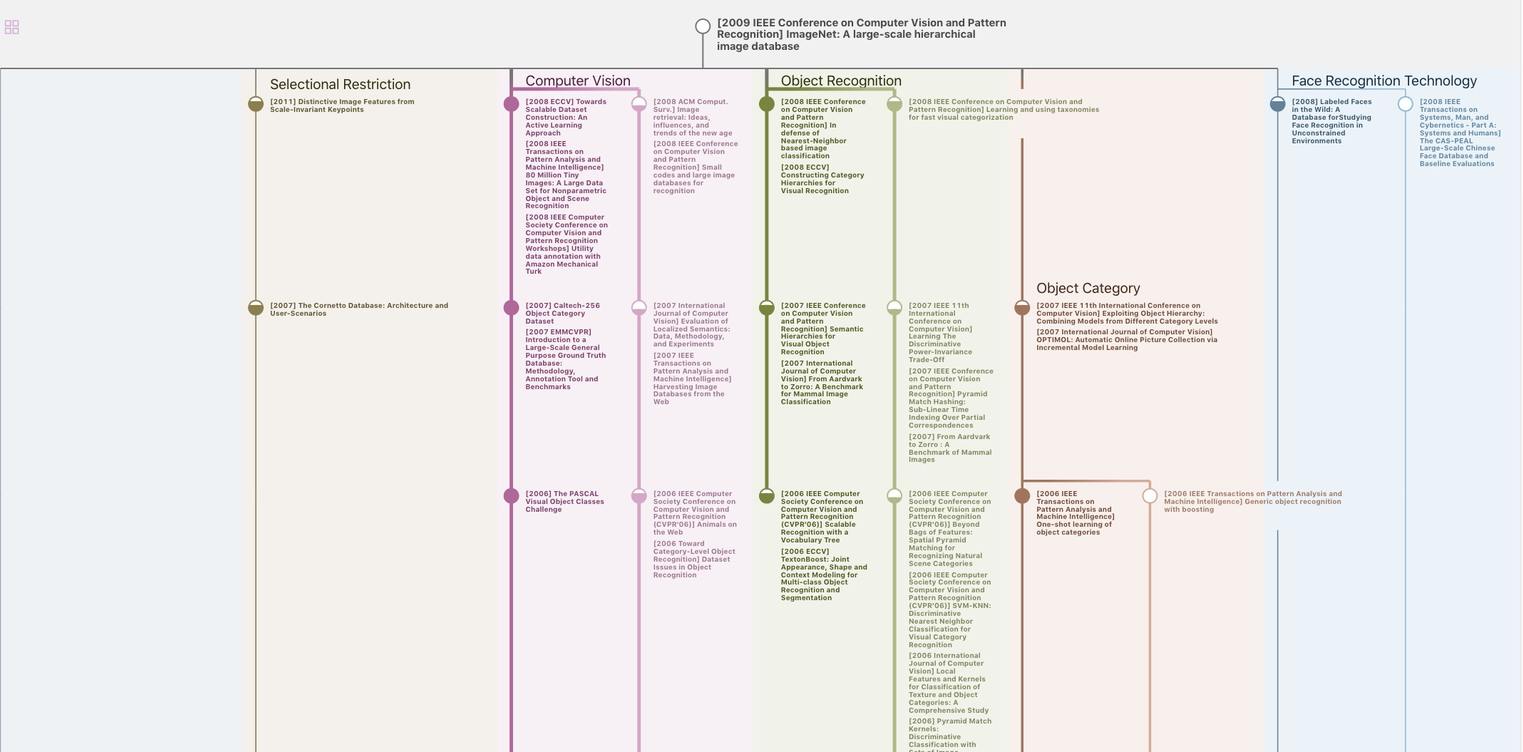
生成溯源树,研究论文发展脉络
Chat Paper
正在生成论文摘要