A Machine Learning Approach to Short-Term Traffic Flow Prediction: A Case Study of Interstate 64 in Missouri
2018 IEEE International Smart Cities Conference (ISC2)(2018)
摘要
Proactive traffic management is a subset of smart mobility applications in which traffic control strategies are implemented in advance to respond to anticipated roadway conditions. Predicted traffic flows are a key input to proactive traffic control systems, such as proactive freeway ramp metering, proactive variable speed limits, and proactive incident management systems. This paper proposes a machine learning approach for short-term traffic flow prediction where prevailing conditions, such as the traffic volume, speed, and occupancy of roadway segments, are used to predict traffic flow in short-term intervals. Four categories of predictive methods for traffic flow prediction were investigated: deep neural networks, a distributed random forest, a gradient boosting machine, and a generalized linear model Data from Interstate 64 in St. Louis, Missouri, in the United States were used to calibrate and evaluate the models. The results obtained by the four predictive methods were very similar, with the distributed random forest model slightly outperforming the models obtained by the other three methods. The case study showed that the inclusion of traffic flow, speed, occupancy, and time of day in the traffic prediction process reduces the traffic prediction error. However, the two-sample Kolmogorov-Smirnov test did not show a statistically significant benefit from the inclusion of upstream traffic data in the distributed random forest, and gradient boosting machine models.
更多查看译文
关键词
Predictive models,Data models,Traffic control,Transportation,Boosting,Decision trees
AI 理解论文
溯源树
样例
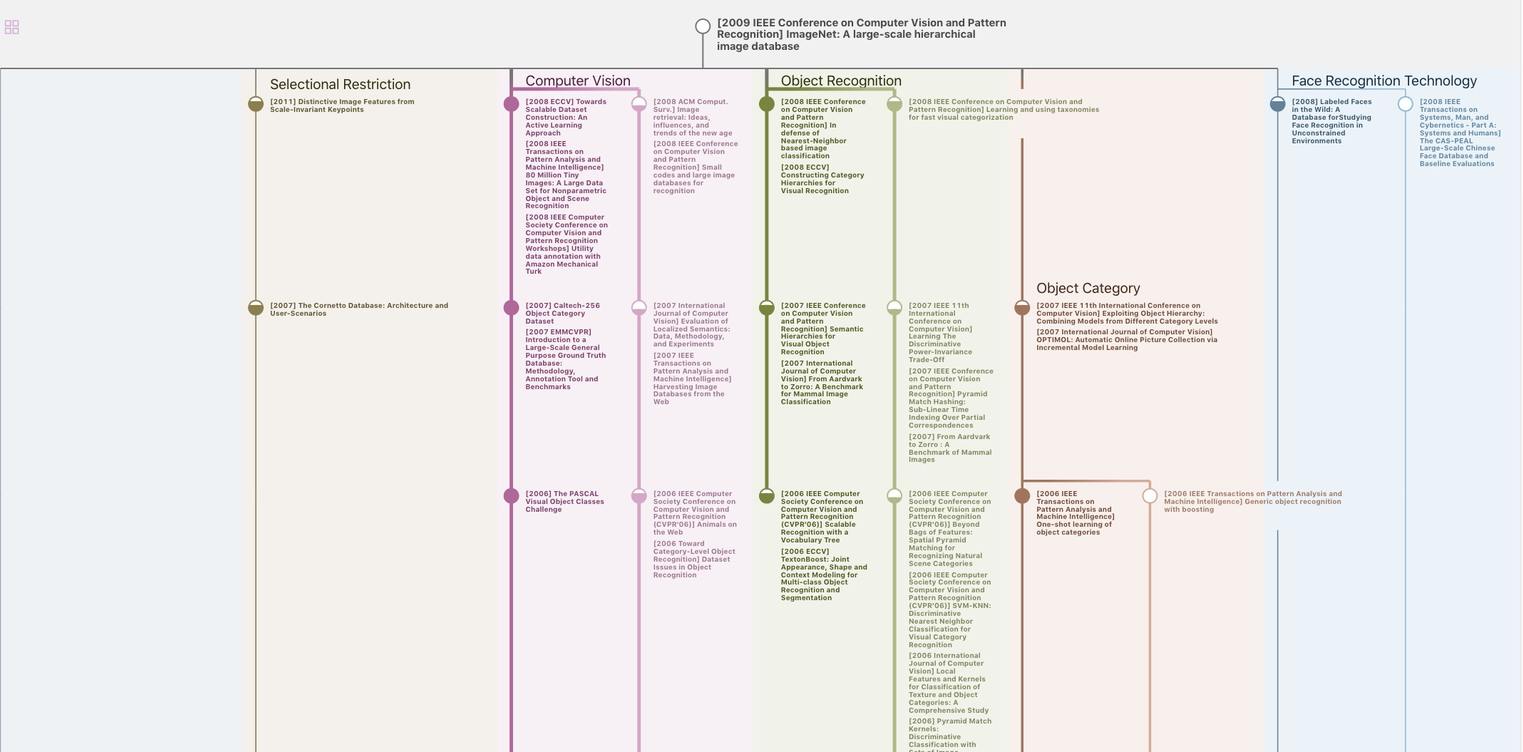
生成溯源树,研究论文发展脉络
Chat Paper
正在生成论文摘要