Detection of Left Ventricular Hypertrophy Using Bayesian Additive Regression Trees: The MESA.
JOURNAL OF THE AMERICAN HEART ASSOCIATION(2019)
摘要
Background-We developed a new left ventricular hypertrophy (LVH) criterion using a machine-learning technique called Bayesian Additive Regression Trees (BART). Methods and Results-This analysis included 4714 participants from MESA (Multi-Ethnic Study of Atherosclerosis) free of clinically apparent cardiovascular disease at enrollment. We used BART to predict LV mass from ECG and participant characteristics using cardiac magnetic resonance imaging as the standard. Participants were randomly divided into a training set (n=3774) and a validation set (n=940). We compared the diagnostic/prognostic performance of our new BART-LVH criteria with traditional ECG-LVH criteria and cardiac magnetic resonance imaging-LVH. In the validation set, BART-LVH showed the highest sensitivity (29.0%; 95% CI, 18.3%39.7%), followed by Sokolow-Lyon-LVH (21.7%; 95% CI, 12.0%-31.5%), Peguero-Lo Presti (14.5%; 95% CI, 6.2%-22.8%), Cornell voltage product (10.1%; 95% CI, 3.0%-17.3%), and Cornell voltage (5.8%; 95% CI, 0.3%-11.3%). The specificity was >93% for all criteria. During a median follow-up of 12.3 years, 591 deaths, 492 cardiovascular disease events, and 332 coronary heart disease events were observed. In adjusted Cox models, both BART-LVH and cardiac magnetic resonance imaging-LVH were associated with mortality (hazard ratio [95% CI], 1.88 [1.45-2.44] and 2.21 [1.74-2.81], respectively), cardiovascular disease events (hazard ratio [95% CI], 1.46 [1.08-1.98] and 1.91 [1.46-2.51], respectively), and coronary heart disease events (hazard ratio [95% CI], 1.72 [1.20-2.47] and 1.96 [1.41-2.73], respectively). These associations were stronger than associations observed with traditional ECG-LVH criteria. Conclusions-Our new BART-LVH criteria have superior diagnostic/prognostic ability to traditional ECG-LVH criteria and similar performance to cardiac magnetic resonance imaging-LVH for predicting events.
更多查看译文
关键词
ECG,ensemble predictive modeling,left ventricular hypertrophy,nonparametric machine learning
AI 理解论文
溯源树
样例
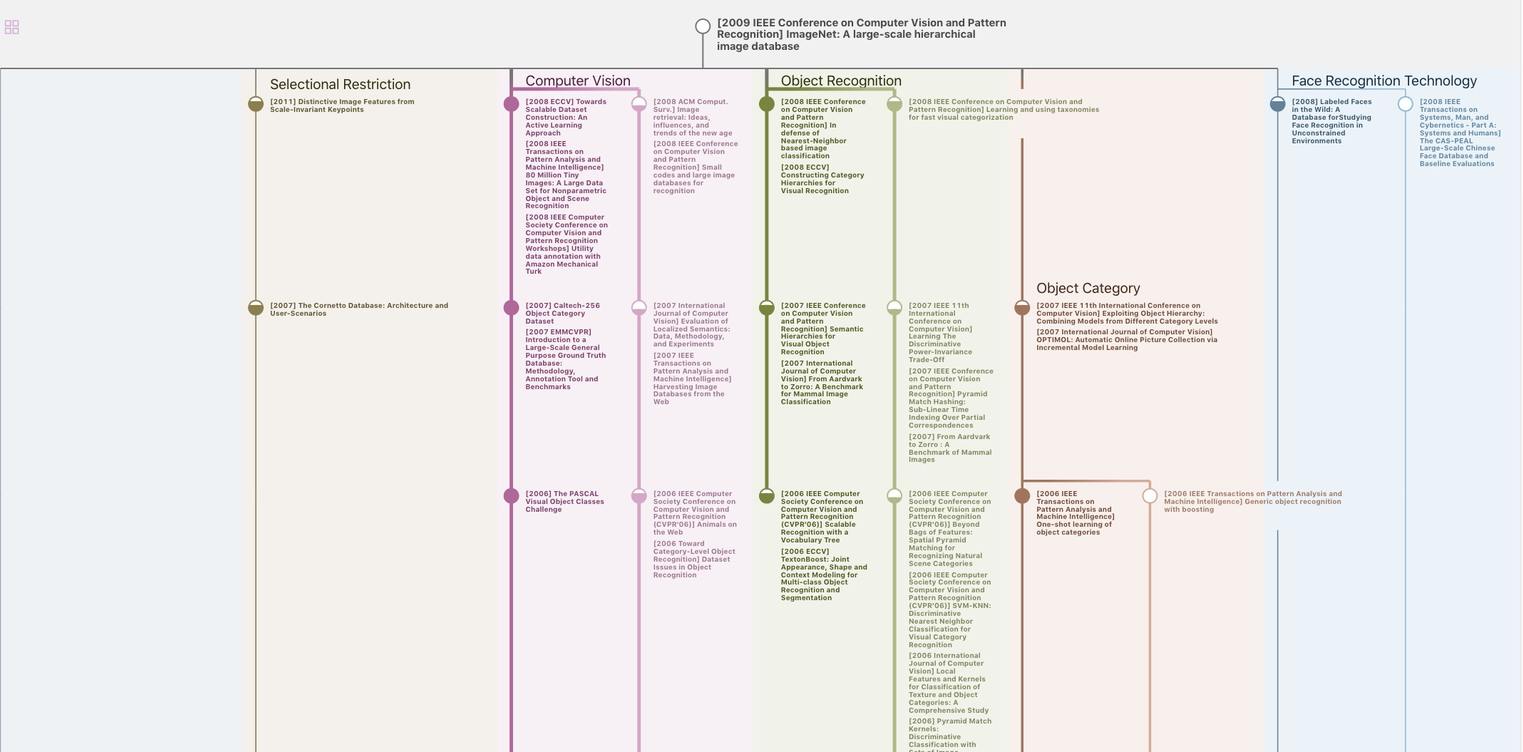
生成溯源树,研究论文发展脉络
Chat Paper
正在生成论文摘要