Determinants of In-Hospital Mortality After Percutaneous Coronary Intervention: A Machine Learning Approach.
JOURNAL OF THE AMERICAN HEART ASSOCIATION(2019)
摘要
Background-The ability to accurately predict the occurrence of in-hospital death after percutaneous coronary intervention is important for clinical decision-making. We sought to utilize the New York Percutaneous Coronary Intervention Reporting System in order to elucidate the determinants of in-hospital mortality in patients undergoing percutaneous coronary intervention across New York State. Methods and Results-We examined 479 804 patients undergoing percutaneous coronary intervention between 2004 and 2012, utilizing traditional and advanced machine learning algorithms to determine the most significant predictors of in-hospital mortality. The entire data were randomly split into a training (80%) and a testing set (20%). Tuned hyperparameters were used to generate a trained model while the performance of the model was independently evaluated on the testing set after plotting a receiver-operator characteristic curve and using the output measure of the area under the curve (AUC) and the associated 95% CIs. Mean age was 65.2 +/- 11.9 years and 68.5% were women. There were 2549 in-hospital deaths within the patient population. A boosted ensemble algorithm (AdaBoost) had optimal discrimination with AUC of 0.927 (95% CI 0.923-0.929) compared with AUC of 0.913 for XGBoost (95% CI 0.906-0.919, P=0.02), AUC of 0.892 for Random Forest (95% CI 0.889-0.896, P<0.01), and AUC of 0.908 for logistic regression (95% CI 0.907-0.910, P<0.01). The 2 most significant predictors were age and ejection fraction. Conclusions-A big data approach that utilizes advanced machine learning algorithms identifies new associations among risk factors and provides high accuracy for the prediction of in-hospital mortality in patients undergoing percutaneous coronary intervention.
更多查看译文
关键词
big data analytics,in-hospital mortality,machine learning,percutaneous coronary intervention
AI 理解论文
溯源树
样例
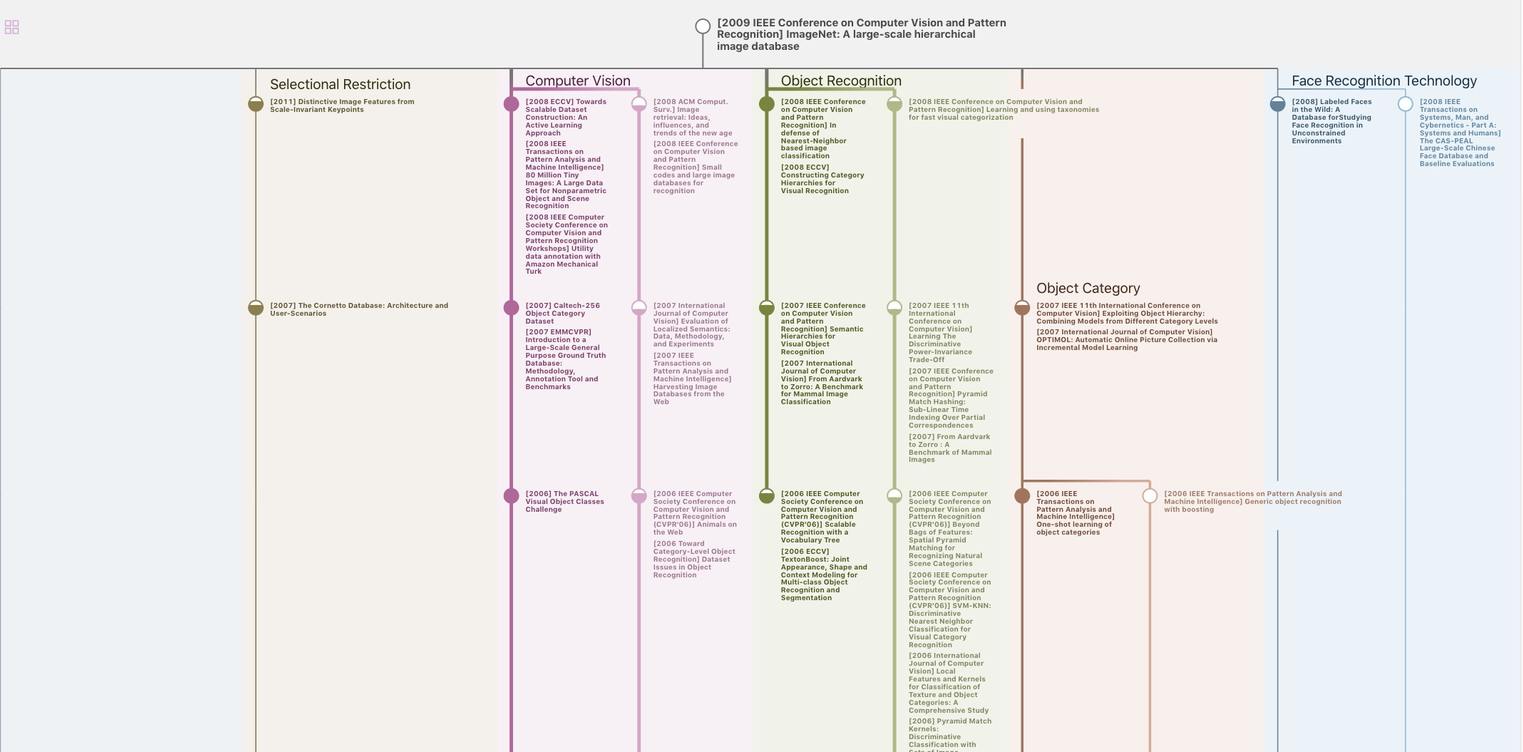
生成溯源树,研究论文发展脉络
Chat Paper
正在生成论文摘要