Restoring the missing high-frequency fluctuations in a wind power model based on reanalysis data
Renewable Energy(2016)
摘要
A previously developed model based on MERRA reanalysis data underestimates the high-frequency variability and step changes of hourly, aggregated wind power generation. The goal of this work is to restore these fluctuations. Since the volatility of the high-frequency signal varies in time, machine learning techniques were employed to predict the volatility. As predictors, derivatives of the output from the original “MERRA model” as well as empirical orthogonal functions of several meteorological variables were used. A FFT-IFFT approach, including a search algorithm for finding appropriate phase angles, was taken to generate a signal that was subsequently transformed to simulated high-frequency fluctuations using the predicted volatility. When comparing to the original MERRA model, the improved model output has a power spectral density and step change distribution in much better agreement with measurements. Moreover, the non-stationarity of the high-frequency fluctuations was captured to a large degree. The filtering and noise addition however resulted in a small increase in the RMS error.
更多查看译文
关键词
Wind power variability,Statistical modelling,Machine learning,Power spectral density,MERRA reanalysis dataset
AI 理解论文
溯源树
样例
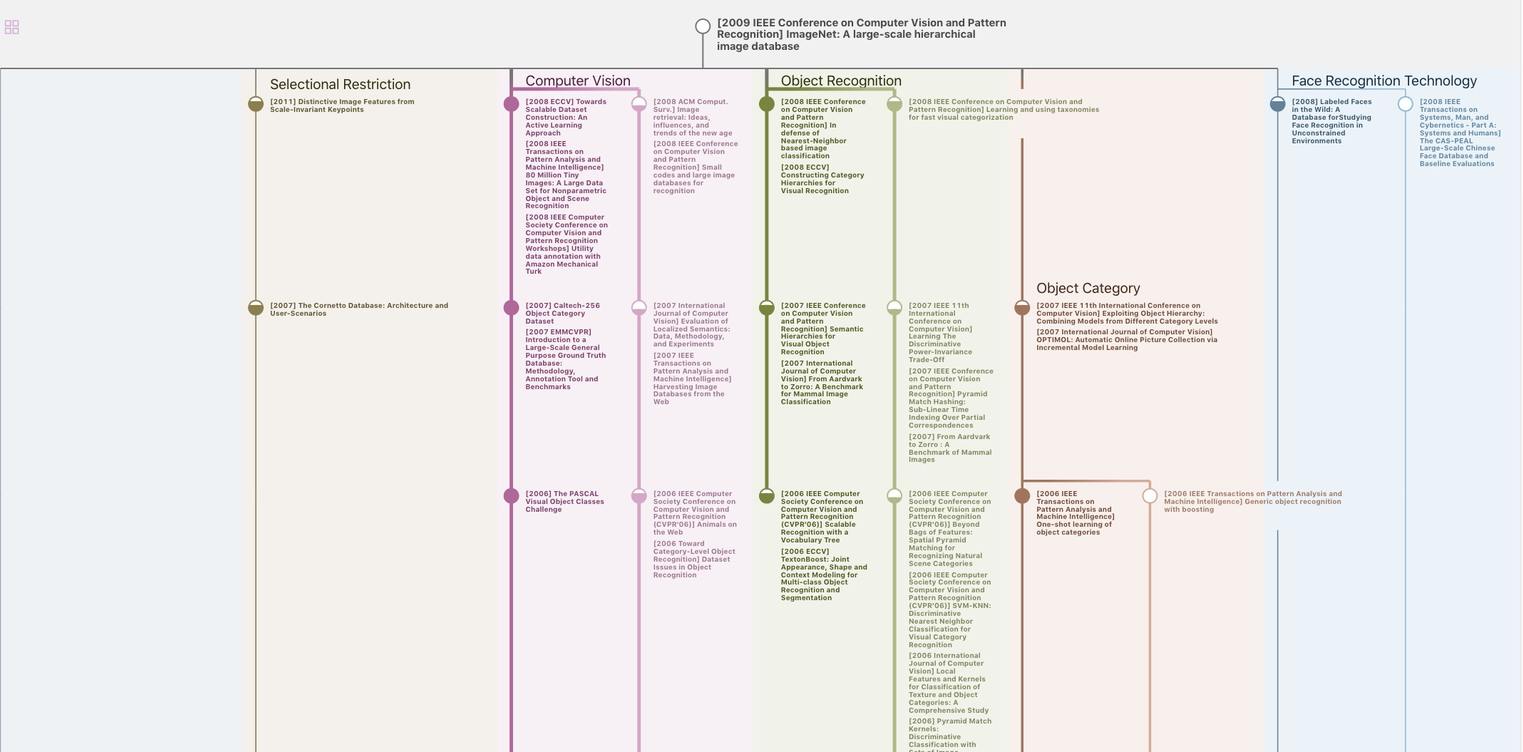
生成溯源树,研究论文发展脉络
Chat Paper
正在生成论文摘要