Incorporating satellite-derived data with annual and monthly land use regression models for estimating spatial distribution of air pollution.
Environmental Modelling & Software(2019)
摘要
The purpose of this study was to assess the performance of annual and monthly land use regression (LUR) models for estimating the spatial distribution of NO2 and PM2.5 in Taiwan. Samples were collected at 73 air quality monitoring sites in 2015. Data transformation coupled with extracting principle components and satellite-derived data were integrated with LUR modeling and applied to increase PM2.5 model performance. Results indicated that NO2 exhibited more robust model performance compared with PM2.5. Leave-one-out cross validation (LOOCV) R2 of NO2 annual model was 0.76 and ranged from 0.56 to 0.81 for monthly models. The LOOCV R2 of PM2.5 annual model was improved from 0.13 to 0.56 by applying principle component analysis and adding satellite data (i.e., percentage of sunshine coverage and aerosol optical depth). These approaches also improved the performance of PM2.5 monthly models. The median LOOCV R2 increased from 0.12 to 0.49.
更多查看译文
关键词
Land use,Fine particulate matter,Nitrogen dioxide,Principle component analysis,Aerosol optical depth
AI 理解论文
溯源树
样例
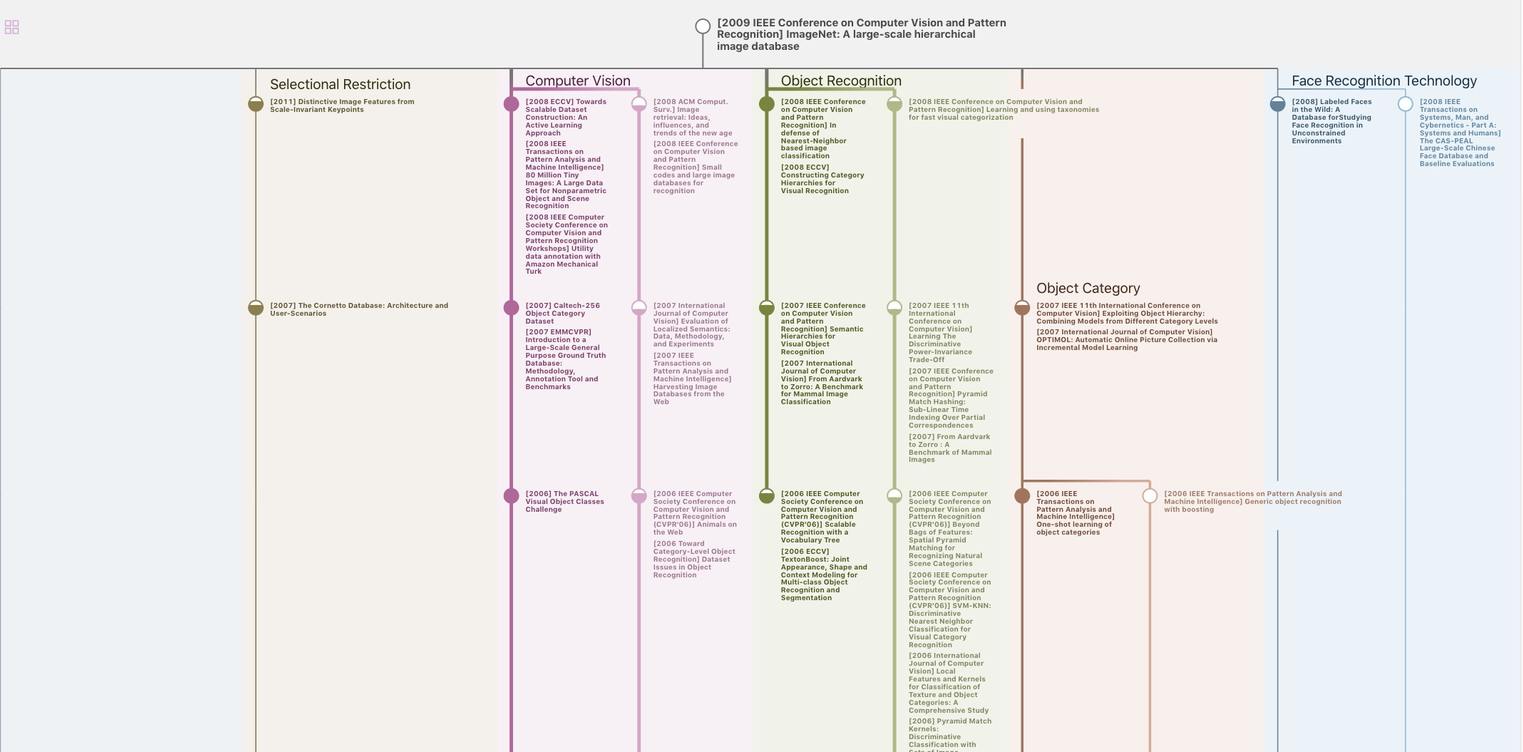
生成溯源树,研究论文发展脉络
Chat Paper
正在生成论文摘要