Online LSTM-based Iterative Mask Estimation for Multi-Channel Speech Enhancement and ASR
2018 Asia-Pacific Signal and Information Processing Association Annual Summit and Conference (APSIPA ASC)(2018)
摘要
Accurate steering vector estimation is the key point for a beamformer which suppresses the background noise to improve the noisy speech quality and intelligibility. Recently, time-frequency masking approach, which estimates the steering vectors that are utilized for a beamformer, is popular in this field. In particular, we have proposed an iterative mask estimation (IME) approach to improve the complex Gaussian mixture model (CGMM) based beamforming and yield the best system for multi-channel ASR in CHiME-4 challenge [1]. And in [2], we also demonstrated that our algorithm could improve the speech quality (PESQ) and intelligibility (STOI) for multi-channel speech enhancement. In this study, we focus on the online processing of our IME algorithm for multi-channel speech enhancement and ASR, which achieves comparable performance to the offline version. In addition, a regression long short-term memory recurrent neural network (LSTM-RNN) for a multiple-target joint learning is utilized, denoted as LSTM-MT, to replace two separate models in [2]. Experiments on the CHiME-4 simulation data show that the online IME algorithm can improve the enhancement performance, e.g., PESQ from 2.18 to 2.58 and STOI from 86.85 to 94.51, which is comparable to those obtained by offline IME. Furthermore, the LSTM-MT based post-processing can achieve an additional PESQ improvement from 2.58 to 2.71. And experiments on the CHiME-4 real data show that the online IME approach outperforms the online CGMM-based approach, with a relative word error reduction (WER) of 14.49%.
更多查看译文
关键词
Speech enhancement,Array signal processing,Estimation,Noise measurement,Signal to noise ratio,Time-frequency analysis
AI 理解论文
溯源树
样例
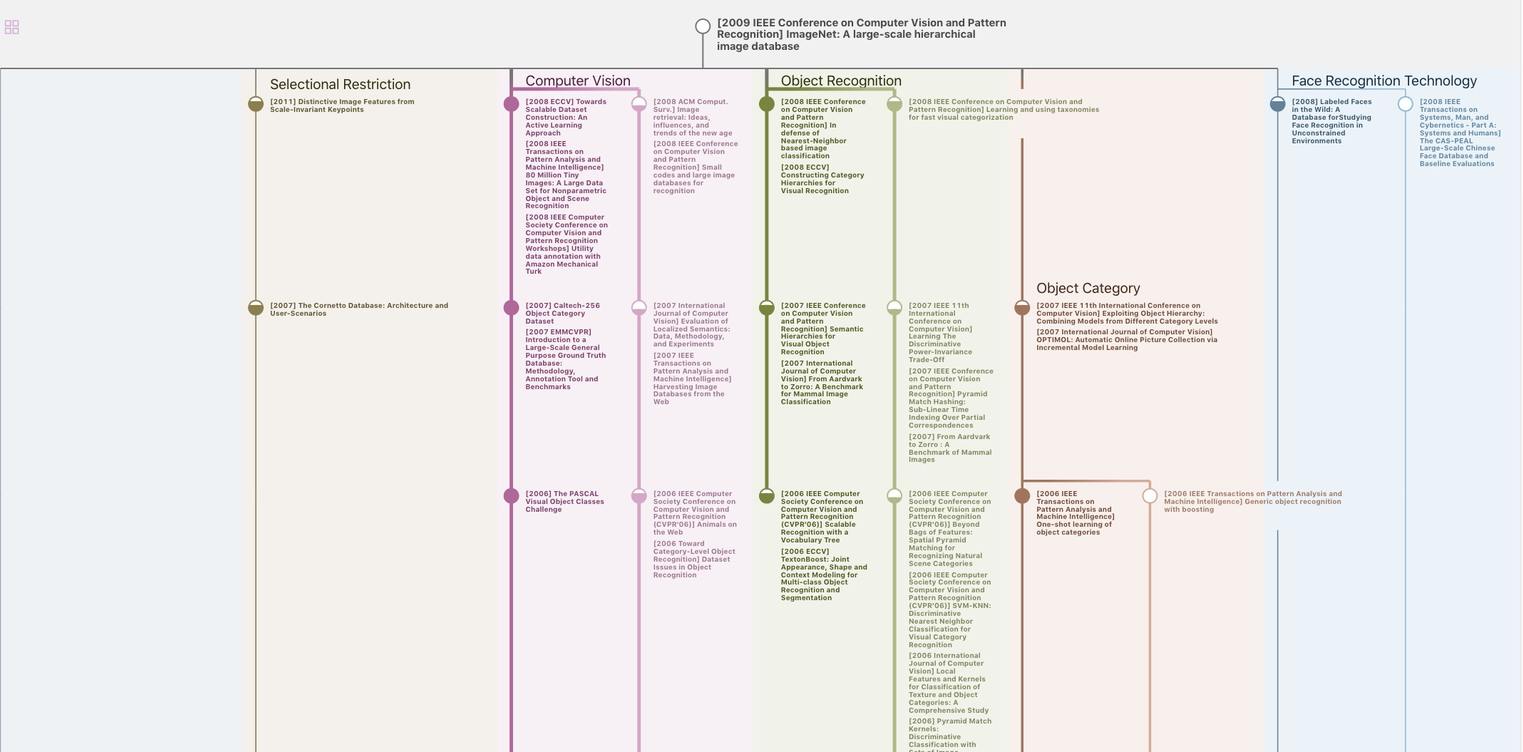
生成溯源树,研究论文发展脉络
Chat Paper
正在生成论文摘要