7.1 An 11.5TOPS/W 1024-MAC Butterfly Structure Dual-Core Sparsity-Aware Neural Processing Unit in 8nm Flagship Mobile SoC
2019 IEEE International Solid- State Circuits Conference - (ISSCC)(2019)
摘要
Deep learning has been widely applied for image and speech recognition. Response time, connectivity, privacy and security drive applications towards mobile platforms rather than cloud. For mobile systems-on-a-chip (SoCs), energy-efficient neural processing units (NPU) have been studied for performing the convolutional layers (CLs) and fully-connected layers (FCLs) [2–5] in deep neural networks. Moreover, considering that neural networks are getting deeper, the NPU needs to integrate 1K or even more multiply/accumulate (MAC) units. For energy efficiency, compression of neural networks has been studied by pruning neural connections and quantizing weights and features with 8b or even lower fixed-point precision without accuracy loss [1]. A hardware accelerator exploited network sparsity for high utilization of MAC units [3]. However, since it is challenging to predict where pruning is possible, the accelerator needed complex circuitry for selecting an array of features corresponding to an array of non-zero weights. For reducing the power of MAC operations, bit-serial multipliers have been applied [5]. Generally, extremely low- or variable-bit-precision neural networks need to be carefully trained.
更多查看译文
AI 理解论文
溯源树
样例
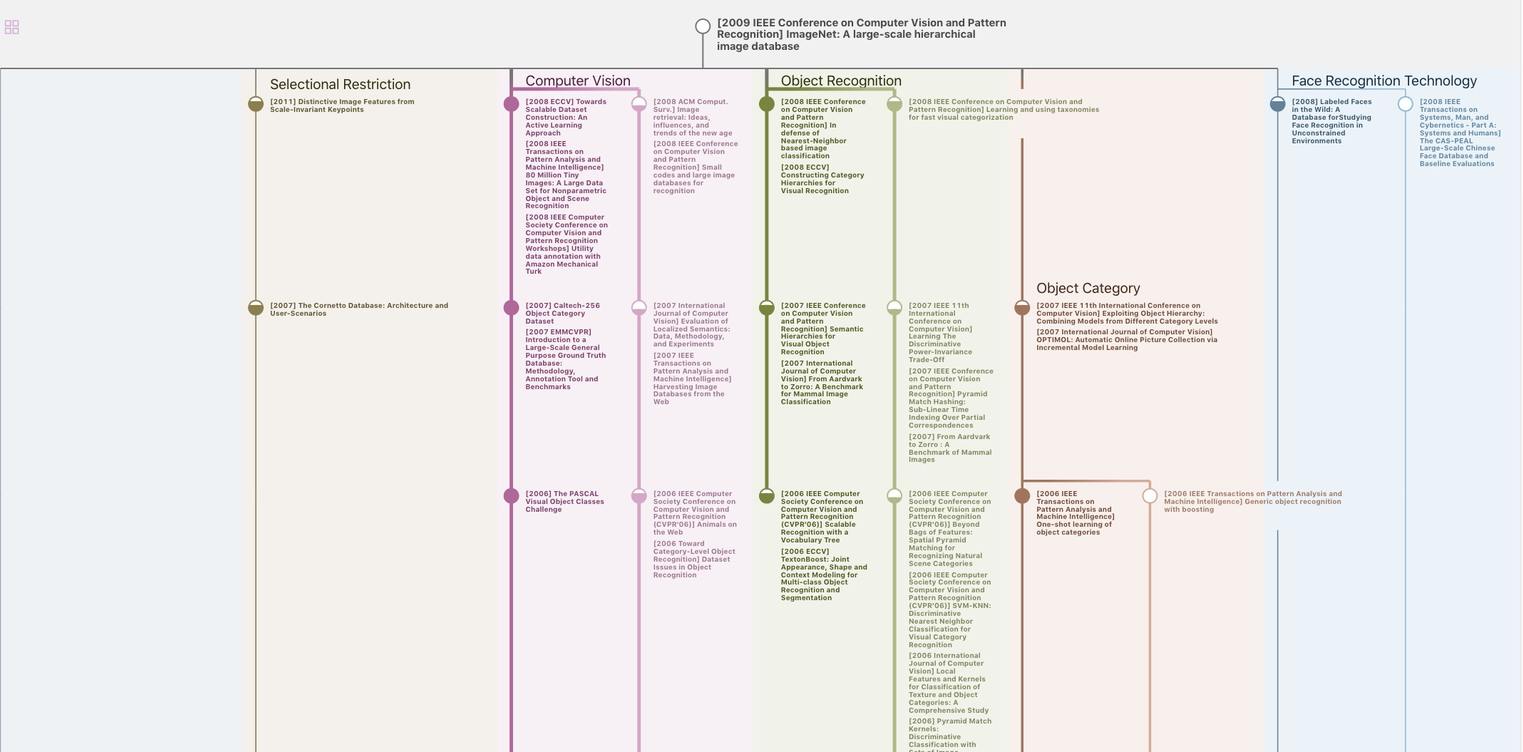
生成溯源树,研究论文发展脉络
Chat Paper
正在生成论文摘要