Classification and Re-Identification of Fruit Fly Individuals Across Days With Convolutional Neural Networks
2019 IEEE Winter Conference on Applications of Computer Vision (WACV)(2019)
摘要
Fruit flies have commonly been used as model organisms to understand various biological processes from genetics and development to social behavior. When studying multiple flies in an experiment, maintaining the identities of individual flies is often essential. The field of "computational ethology" has provided several methods that help biologists in this regard. Unmarked fly identities can be maintained by tracking each fly throughout the experiment, either manually correcting errors or computing and storing the statistics at each frame, but this becomes infeasible for experiments spanning several days. A popular alternative is to mark insects (with tags, paint or wing-clipping), but this has shown to have adverse effects on many of the same behaviors under study. To solve the above problems, we developed a method that can augment existing tracking software with a deep learning-based approach to recognize and re-identify individual fruit flies across days. This method should prove invaluable to biologists as it promises to revolutionize our ability to perform long-term experiments. To the best of our knowledge, this is the first work to investigate and successfully re-identify unmarked fruit flies across multiple days. We acquired our own dataset, which has around 3M images of 60 different fruit fly individuals, acquired in independent sets of 20. A vast majority (95%) of the flies have greater than 90% accuracy on a per-frame basis, and a majority of those (74%) have over 98% accuracy. With our method, less than 1 fly in 20 (on average) should be difficult to identify without resorting to parsimonious methods (i.e. examining which ID has not yet been assigned). This high classification accuracy demonstrates that we are able to successfully re-identify unmarked flies across days.
更多查看译文
关键词
Insects,Biological information theory,Visualization,Software,Task analysis,Training
AI 理解论文
溯源树
样例
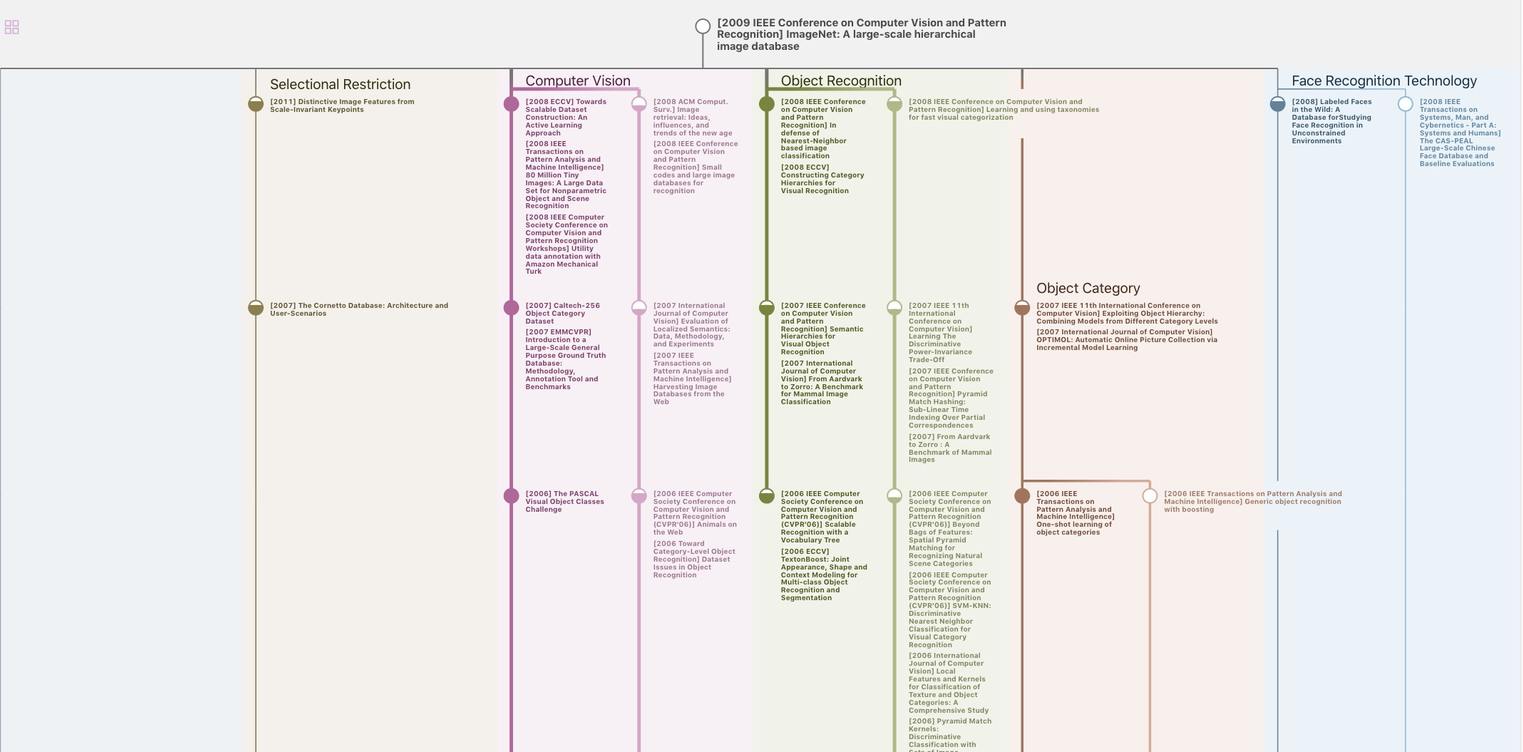
生成溯源树,研究论文发展脉络
Chat Paper
正在生成论文摘要