Bayesian Multichannel Speech Enhancement with a Deep Speech Prior.
Asia-Pacific Signal and Information Processing Association Annual Summit and Conference(2018)
摘要
This paper describes statistical multichannel speech enhancement based on a deep generative model of speech spectra. Recently, deep neural networks (DNNs) have widely been used for converting noisy speech spectra to clean speech spectra or estimating time-frequency masks. Such a supervised approach, however, requires a sufficient amount of training data (pairs of noisy speech data and clean speech data) and often fails in an unseen noisy environment. This calls for a blind source separation method called multichannel nonnegative matrix factorization (MNMF) that can jointly estimate low-rank source spectra and spatial covariances on the fly. However, the assumption of low-rankness does not hold true for speech spectra. To solve these problems, we propose a semi-supervised method based on an extension of MNMF that consists of a deep generative model for speech spectra and a standard low-rank model for noise spectra. The speech model can be trained in advance with auto-encoding variational Bayes (AEVB) by using only clean speech data and is used as a prior of clean speech spectra for speech enhancement. Given noisy speech spectrogram, we estimate the posterior of clean speech spectra while estimating the noise model on the fly. Such adaptive estimation is achieved by using Gibbs sampling in a unified Bayesian framework. The experimental results showed the potential of the proposed method.
更多查看译文
关键词
Bayesian multichannel speech enhancement,statistical multichannel speech enhancement,deep generative model,deep neural networks,noisy speech spectrogram model,clean speech data spectra,DNN,noisy speech spectra conversion,time-frequency mask estimation,supervised approach,blind source separation method,multichannel nonnegative matrix factorization,MNMF,low-rank source spectra estimation model,spatial covariance on the fly,semisupervised method,AEVB,autoencoding variational Bayes,adaptive estimation,Gibbs sampling,unified Bayesian framework
AI 理解论文
溯源树
样例
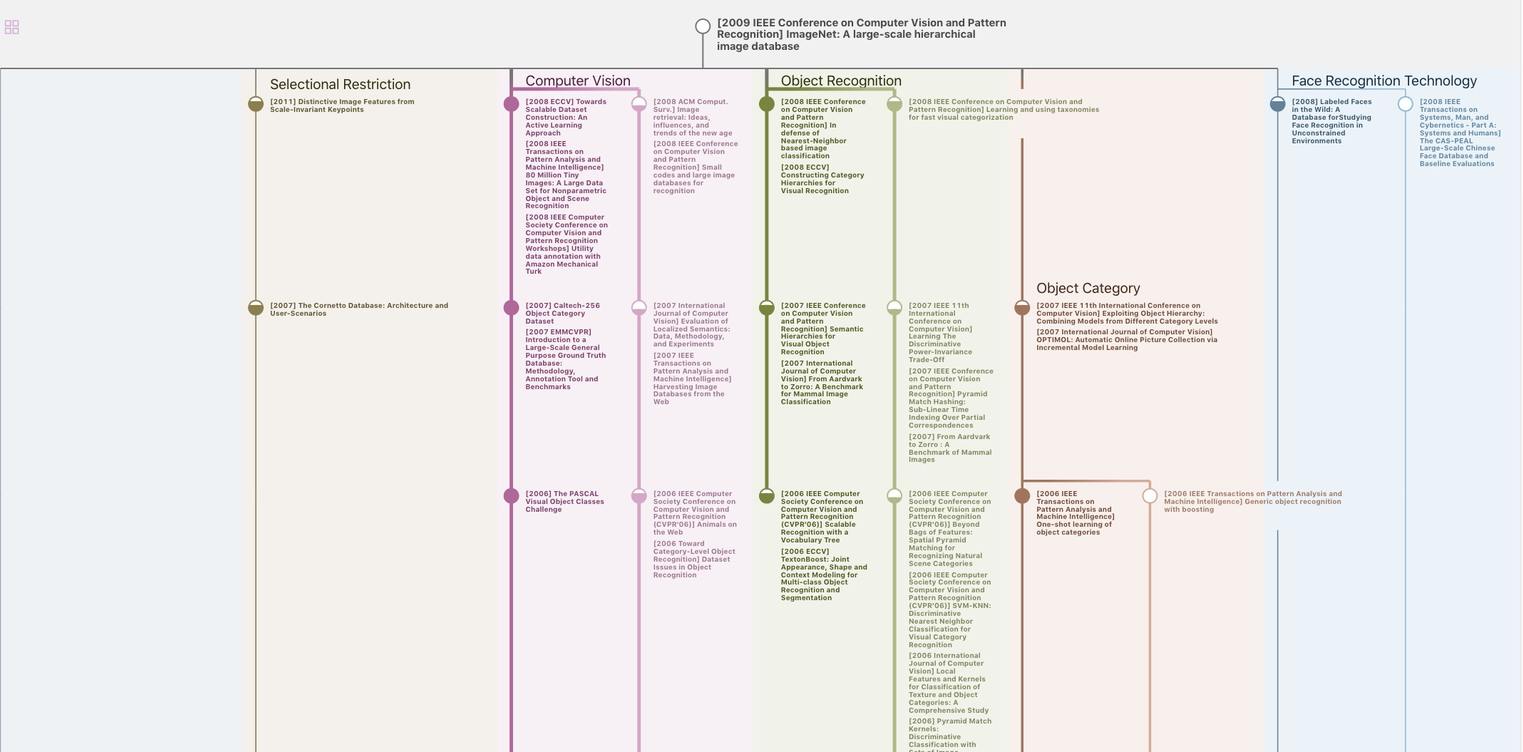
生成溯源树,研究论文发展脉络
Chat Paper
正在生成论文摘要