Correcting for Cryptic Relatedness in Genome-Wide Association Studies
arXiv: Quantitative Methods(2016)
摘要
While the individuals chosen for a genome-wide association study (GWAS) may not be closely related to each other, there can be distant (cryptic) relationships that confound the evidence of disease association. These cryptic relationships violate the GWAS assumption regarding the independence of the subjects' genomes, and failure to account for these relationships results in both false positives and false negatives. This paper presents a method to correct for these cryptic relationships. We accurately detect distant relationships using an expectation maximization (EM) algorithm for finding the identity coefficients from genotype data with know prior knowledge of relationships. From the identity coefficients we compute the kinship coefficients and employ a kinship-corrected association test. We assess the accuracy of our EM kinship estimation algorithm. We show that on genomes simulated from a Wright-Fisher pedigree, our method converges quickly and requires only a relatively small number of sites to be accurate. We also demonstrate that our kinship coefficient estimates outperform state-of-the-art covariance-based approaches and PLINK's kinship estimate. To assess the kinship-corrected association test, we simulated individuals from deep pedigrees and drew one site to recessively determine the disease status. We applied our EM algorithm to estimate the kinship coefficients and ran a kinship-adjusted association test. Our approach compares favorably with the state-of-the-art and far out-performs a na\"ive association test. We advocate use of our method to detect cryptic relationships and for correcting association tests. Not only is our model easy to interpret due to the use of identity states as latent variables, but also inference provides state-of-the-art accuracy.
更多查看译文
AI 理解论文
溯源树
样例
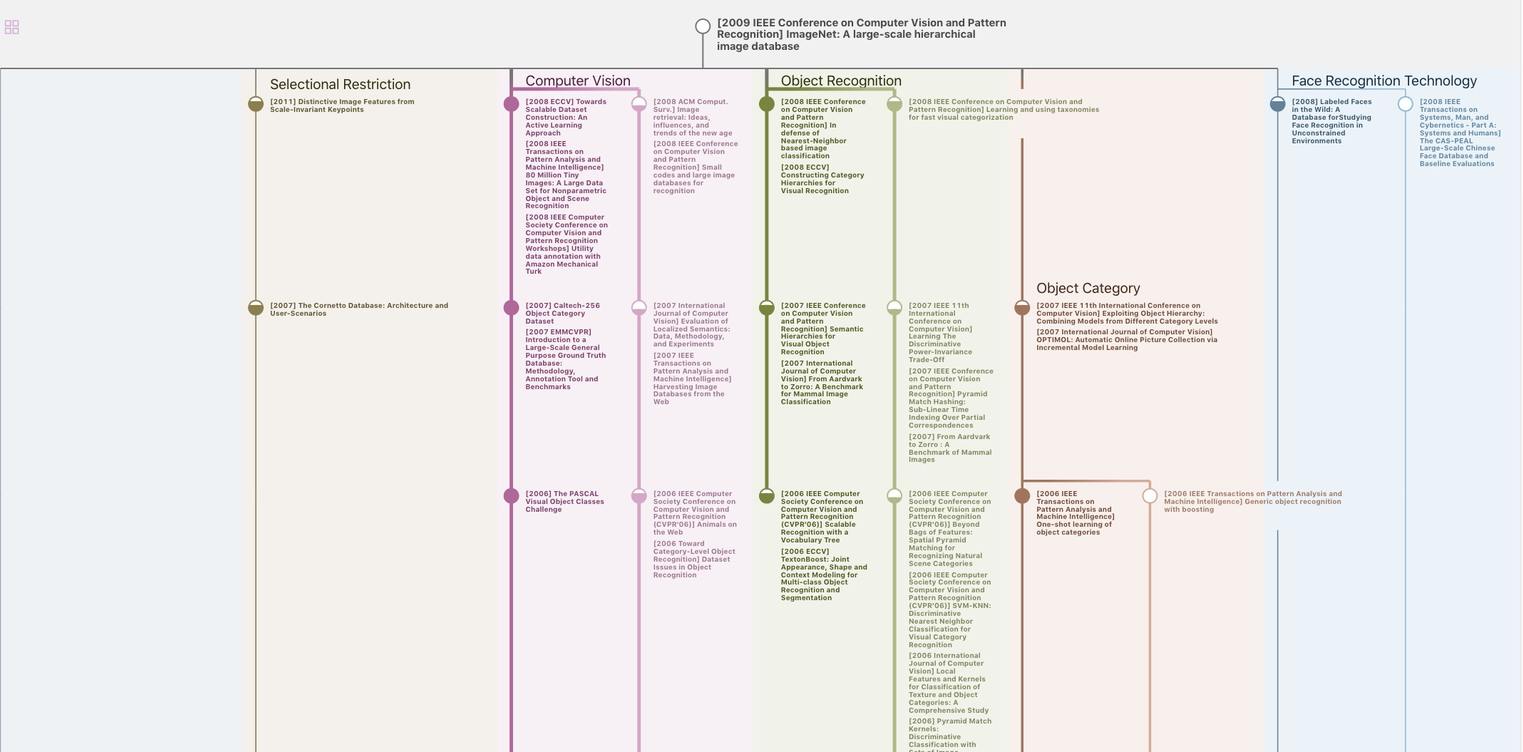
生成溯源树,研究论文发展脉络
Chat Paper
正在生成论文摘要