SD-HOC - Seasonal Decomposition Algorithm for Mining Lagged Time Series.
AusDM(2017)
摘要
Mining time series data is a difficult process due to the lag factor and different time of data arrival. In this paper, we present Seasonal Decomposition for Human Occupancy Counting (SD-HOC), a customised feature transformation decomposition, novel way to estimate the number of people within a closed space using only a single carbon dioxide sensor. SD-HOC integrates time lag and line of best fit model in the preprocessing algorithms. SD-HOC utilises seasonal-trend decomposition with moving average to transform the preprocessed data and for each trend, seasonal and irregular component, different regression algorithms are modelled to predict each respective human occupancy component value. Utilising M5 method linear regression for trend and irregular component and dynamic time warping for seasonal component, a set of the prediction value for each component was obtained. Zero pattern adjustment model is infused to increase the accuracy and finally, additive decomposition is used to reconstruct the prediction value. The accuracy results are compared with other data mining algorithms such as decision tree, multi-layer perceptron, Gaussian processes - radial basis function, support vector machine, random forest, naive Bayes and support vector regression in two different locations that have different contexts.
更多查看译文
关键词
Ambient sensing, Building occupancy, Presence detection, Number estimation, Cross-space modeling, Contextual information, Human occupancy detection, Carbon dioxide, Machine learning
AI 理解论文
溯源树
样例
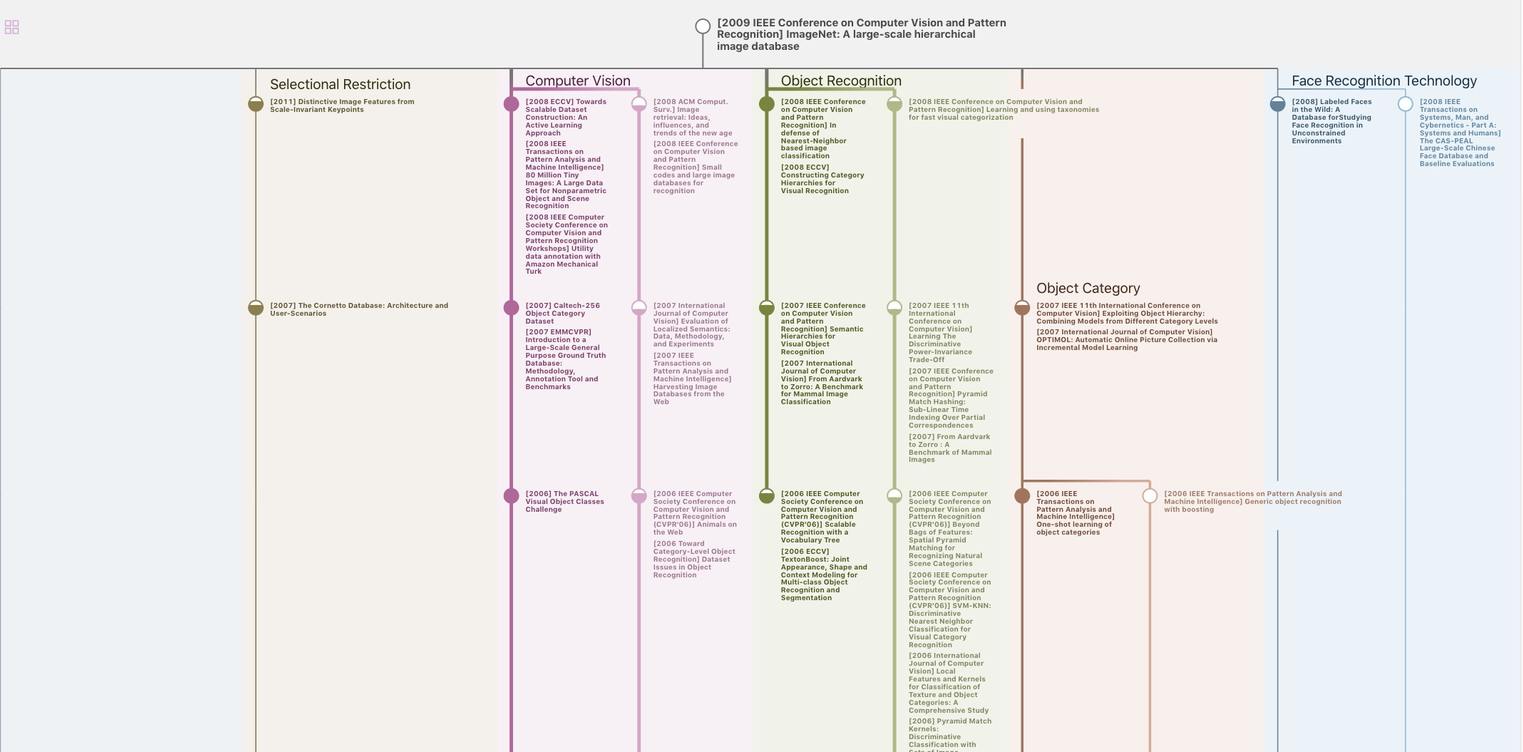
生成溯源树,研究论文发展脉络
Chat Paper
正在生成论文摘要