An iterative learning approach for anticipatory traffic signal control on urban networks
TRANSPORTMETRICA B-TRANSPORT DYNAMICS(2017)
摘要
Traffic signal control on urban road networks offers high opportunity to improve traffic operations. Among others, anticipatory control determines signal timings to optimize network performance, taking into account explicitly the route choice responses and resulting (equilibrium) network flow patterns. However, the optimal control decisions are usually calculated using equilibrium flow models that are in general only a coarse representation of reality. As a result, model-reality mismatch often leads to suboptimal conditions characterized by unexpected congestion effects. This paper presents a novel method to support control decisions for practical applications in real traffic systems that operate repeatedly, for instance from week to week, month to month, and in the presence of flow measurements. The proposed method generates a sequence of control settings to track the real flow response, by observing errors between modeled flows and the measurements. Improvement in the control performance is achieved by learning from this error information. A theoretical analysis on convergence of the control sequence is provided and the impact of different weighting parameters on the convergence performance is discussed. Numerical examples on a test network confirm the effectiveness of the proposed iterative learning approach in tackling modeling error, as well as a main role of model bias correction in tracking reality. The impact of weighting parameters on its convergence is also illustrated.
更多查看译文
关键词
Anticipatory traffic control,modeling error,iterative learning,reality-tracking,model bias correction
AI 理解论文
溯源树
样例
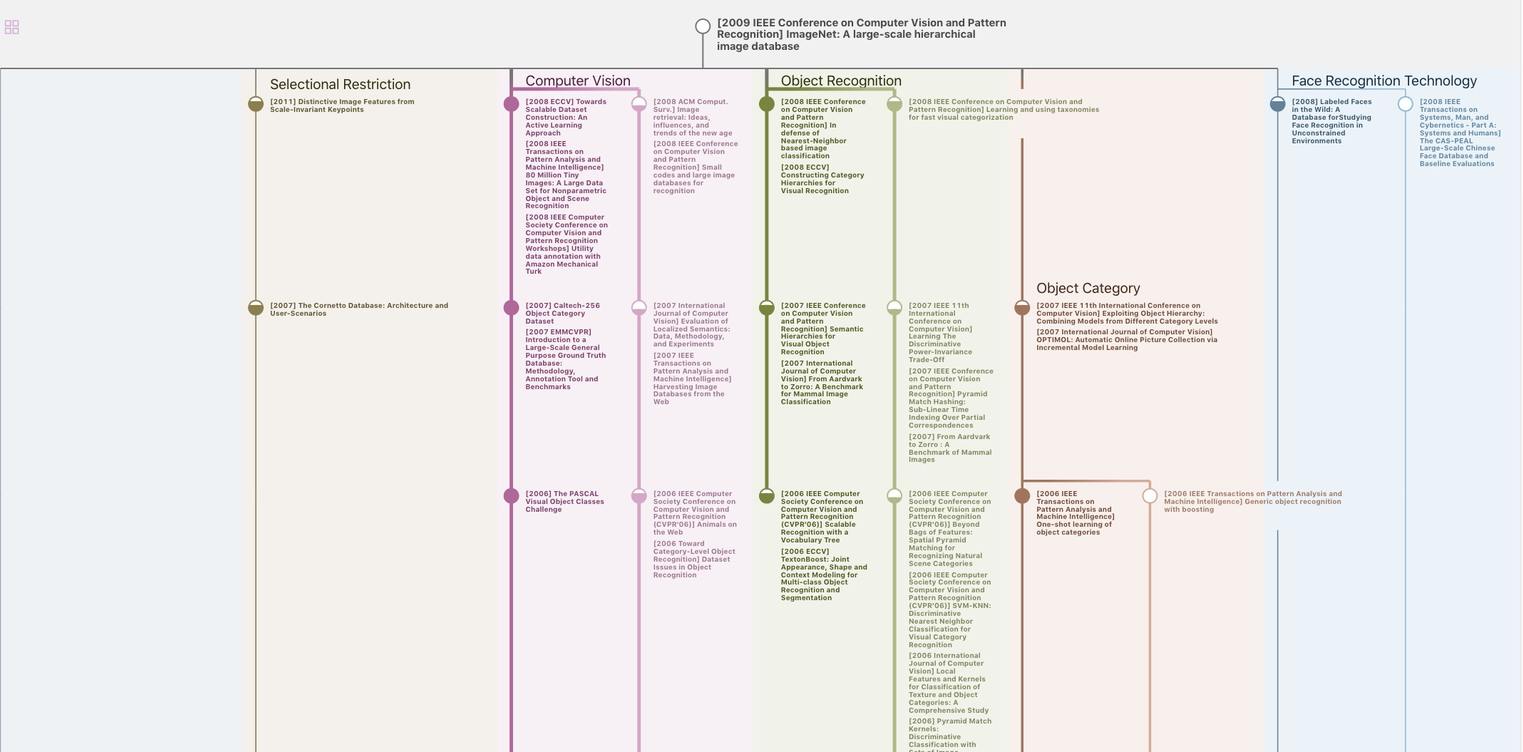
生成溯源树,研究论文发展脉络
Chat Paper
正在生成论文摘要