Nuclear detection using Higher-Order topic modeling
Homeland Security(2012)
摘要
In this paper, a novel approach to topic modeling based on the Higher Order Learning framework, Higher-Order Latent Dirichlet Allocation (HO-LDA), is applied to a critical issue in homeland security, nuclear detection. In addition, this research strives to improve topic models in the `real time' environment of online learning. In total, seventeen different nuclear radioisotopes are classified, and performance of Higher-Order versus traditional techniques is evaluated. This project employs LDA and HO-LDA on a nuclear detection numeric dataset to gain a topic decomposition of instances. These learned topics are then used as features in a traditional supervised classification algorithm. In essence, the LDA or HO-LDA topic assignments are used as features in supervised learning algorithms that predict the class (isotope), treating LDA or HO-LDA as a feature space transform. Using Topic Modeling on numeric nuclear detection data is cutting edge, as to our knowledge this has never been done before on a nuclear detection dataset. Two methods of feature transformation are evaluated, including Multinomial Feature Creation and Maximum Channel Value Feature Creation. Results demonstrate further evidence that Higher Order Learning techniques can be usefully applied in topic modeling applied to nuclear detection.
更多查看译文
关键词
feature extraction,learning (artificial intelligence),national security,pattern classification,public administration,radiation detection,radioisotopes,signal classification,spectral analysis,ho-lda,class prediction,feature space transform,feature transformation,higher order learning framework,higher-order latent dirichlet allocation,higher-order topic modeling,homeland security,isotope prediction,maximum channel value feature creation,multinomial feature creation,nuclear detection numeric dataset,nuclear radioisotope classification,online learning,supervised classification algorithm,supervised learning algorithm,topic assignment,topic decomposition,topic learning,lda,higher-order learning,latent dirichlet allocation,machine learning,nuclear detection,topic modeling,learning artificial intelligence
AI 理解论文
溯源树
样例
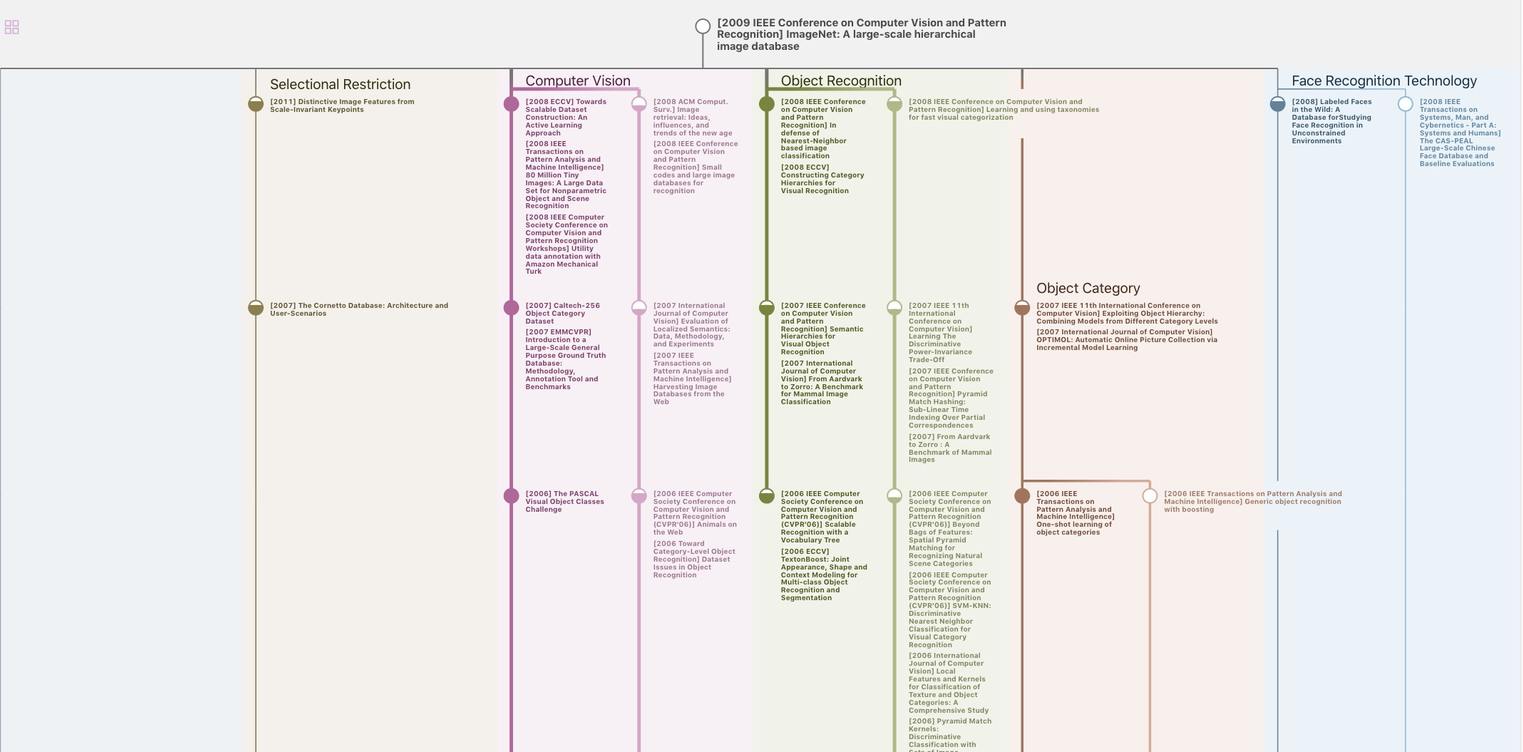
生成溯源树,研究论文发展脉络
Chat Paper
正在生成论文摘要