Inferring the brain's internal model from sensory responses in a probabilistic inference framework
bioRxiv(2020)
摘要
During perception, the brain combines information received from its senses with prior information about the outside world. The mathematical concept of probabilistic inference has previously been suggested as a framework for understanding both perception and cognition. Whether this framework can explain not only behavior but also the underlying neural computations has been an open question. We propose that sensory neuronsu0027 activity represents a central quantity of Bayesian computations: posterior beliefs about the outside world. As a result, sensory responses, just like the beliefs themselves, should depend both on sensory inputs and on prior information represented in other parts of the brain. We show that this dependence on internal variables induces variability in sensory responses that -- in the context of a psychophysical task -- is related both to the structure of that task and to the neuronsu0027 stimulus tuning. We derive analytical predictions for the correlation between different neuronsu0027 responses, and for their correlation with behavior. Furthermore, we show that key neurophysiological observations from much studied perceptual discrimination and detection experiments agree with those predictions. Our work thereby provides a normative explanation for those observations, requiring a reinterpretation of the role of correlated variability for sensory coding. Finally, the fact that sensory responses (which we observe) are a product both of external inputs (which we control) and of internal beliefs, allows us to reverse-engineer information about the subjectu0027s internal beliefs by observing sensory neuronsu0027 responses alone. Population recordings of sensory neurons in animals performing a task can therefore be used to track changes in the internal beliefs with learning and attention.
更多查看译文
AI 理解论文
溯源树
样例
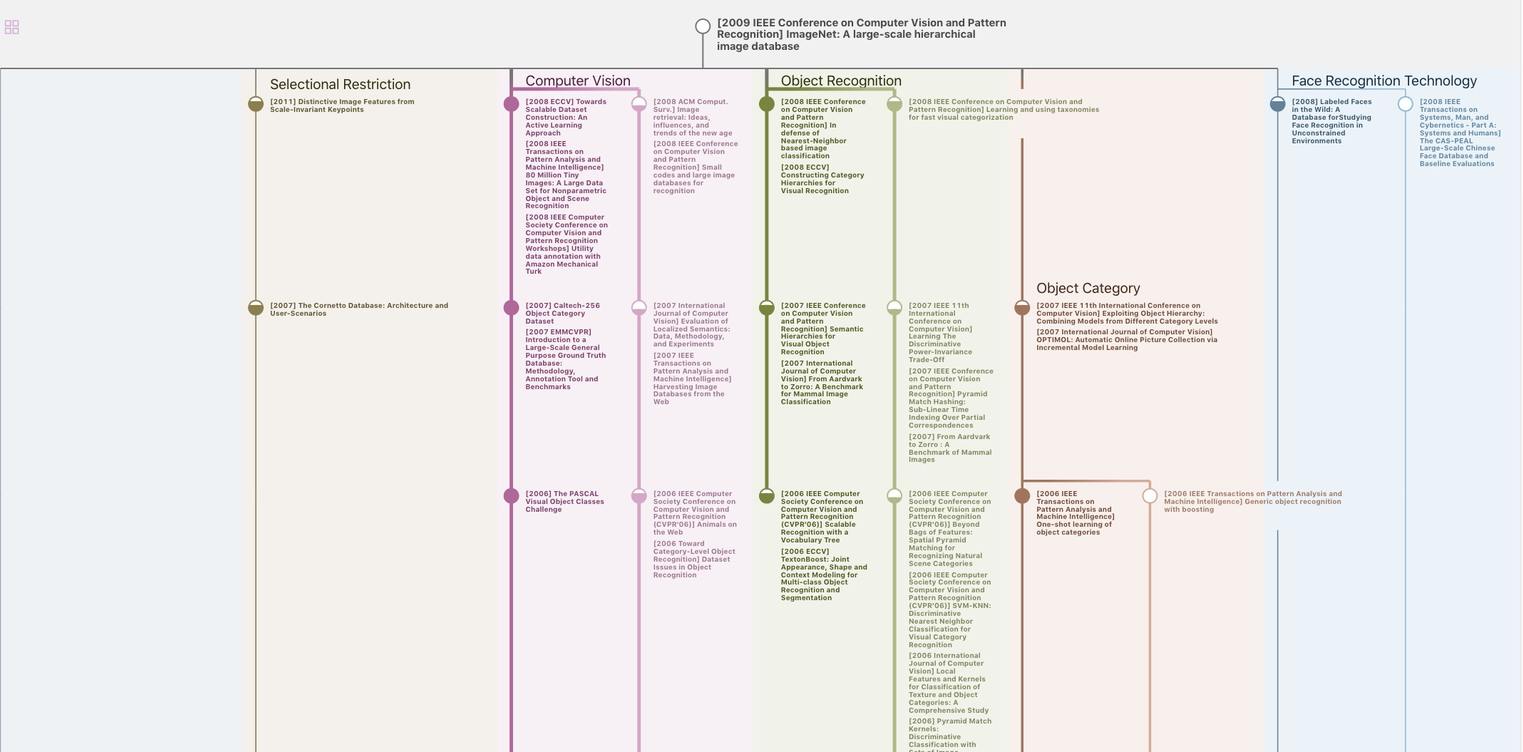
生成溯源树,研究论文发展脉络
Chat Paper
正在生成论文摘要