Fine-Grained Abnormal Driving Behaviors Detection and Identification with Smartphones.
IEEE Trans. Mob. Comput.(2017)
摘要
Real-time abnormal driving behaviors monitoring is a corner stone to improving driving safety. Existing works on driving behaviors monitoring using smartphones only provide a coarse-grained result, i.e., distinguishing abnormal driving behaviors from normal ones. To improve drivers' awareness of their driving habits so as to prevent potential car accidents, we need to consider a fine-grained monitoring approach, which not only detects abnormal driving behaviors but also identifies specific types of abnormal driving behaviors, i.e., Weaving, Swerving, Sideslipping, Fast U-turn, Turning with a wide radius, and Sudden braking. Through empirical studies of the 6-month driving traces collected from real driving environments, we find that all of the six types of driving behaviors have their unique patterns on acceleration and orientation. Recognizing this observation, we further propose a fine-grained abnormal Driving behavior Detection and iDentification system, D 3 , to perform real-time high-accurate abnormal driving behaviors monitoring using smartphone sensors. We extract effective features to capture the patterns of abnormal driving behaviors. After that, two machine learning methods, Support Vector Machine (SVM) and Neuron Networks (NN), are employed, respectively, to train the features and output a classifier model which conducts fine-grained abnormal driving behaviors detection and identification. From results of extensive experiments with 20 volunteers driving for another four months in real driving environments, we show that D 3 achieves an average total accuracy of 95.36 percent with SVM classifier model, and 96.88 percent with NN classifier model.
更多查看译文
关键词
Sensors,Smart phones,Feature extraction,Accelerometers,Monitoring,Automobiles
AI 理解论文
溯源树
样例
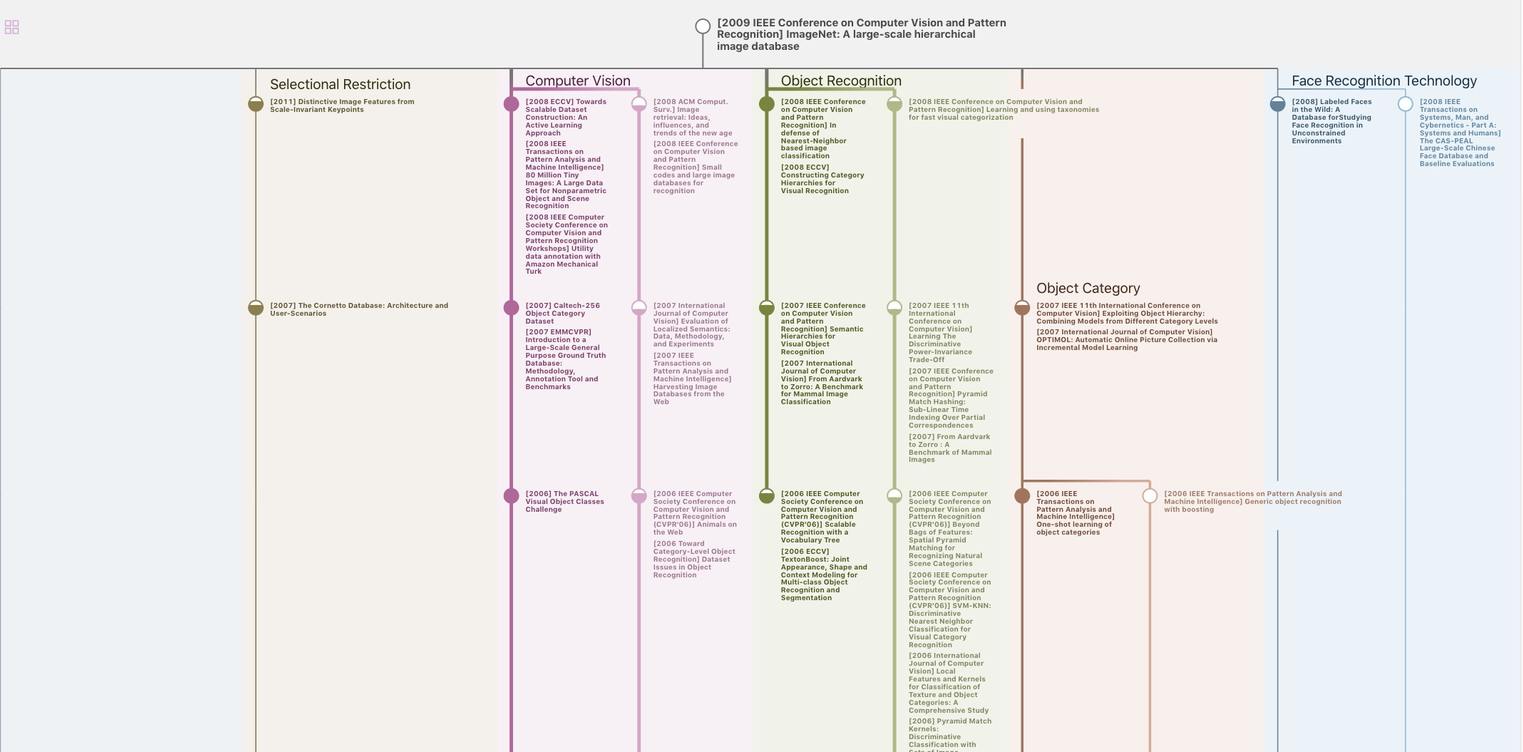
生成溯源树,研究论文发展脉络
Chat Paper
正在生成论文摘要