An empirical analysis of the optimization of deep network loss surfaces
arXiv: Learning(2016)
摘要
The success of deep neural networks hinges on our ability to accurately and efficiently optimize high-dimensional, non-convex loss functions. In this paper, we empirically investigate the geometry of the loss functions of state-of-the-art networks, and how commonly-used stochastic gradient descent variants optimize these loss functions. To do this, we visualize the loss function by projecting it down to low-dimensional spaces chosen based on the convergence points of different optimization algorithms. Our observations suggest that optimization algorithms encounter and choose different descent directions at many saddle points to find different final weights. Based on consistency we observe across re-runs of the same stochastic optimization algorithm, we hypothesize that each optimization algorithm makes characteristic choices at these saddle points.
更多查看译文
AI 理解论文
溯源树
样例
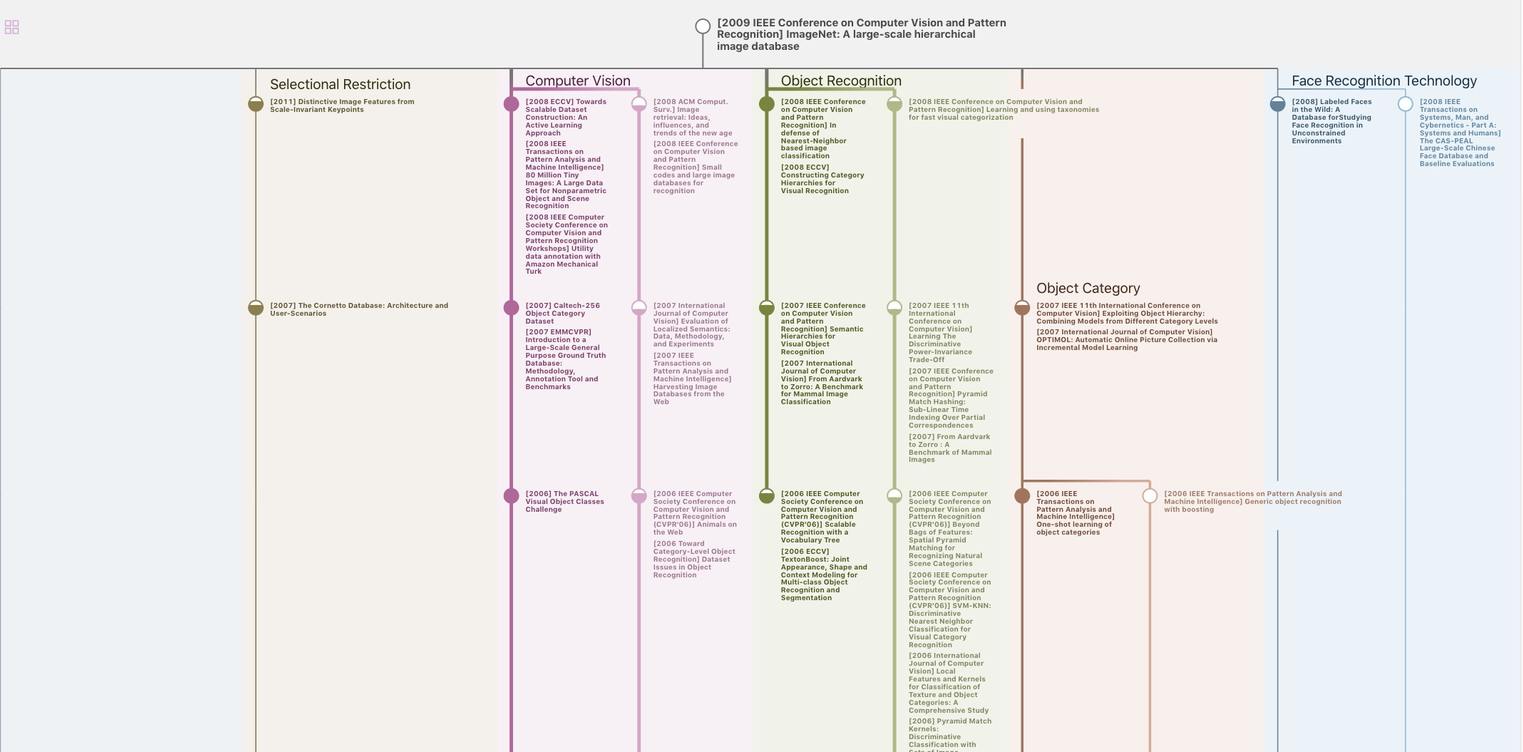
生成溯源树,研究论文发展脉络
Chat Paper
正在生成论文摘要