Human activity monitoring based on hidden Markov models using a smartphone.
IEEE INSTRUMENTATION & MEASUREMENT MAGAZINE(2016)
摘要
This paper presents an human sensing (HS) system based on Hidden Markov Models (HMMs) for classifying physical activities: walking, walking-upstairs, walking-downstairs, sitting, standing and lying down. The system includes a feature extractor (developed by the authors and presented in a previous work), an HMMs training module and an HAR module. All experiments have been done using a publicly available dataset named UCI Human Activity Recognition Using Smartphones. The final results using HMMs obtain comparable results to other recognition methods. Some improvements have been obtained when considering a discriminative HMM training procedure. The best result obtains an activity recognition error rate (ARER) of 2.5%. This work is focused on independent activity recognition and extends other works from the same authors focused on activity segmentation and feature extraction.
更多查看译文
关键词
Hidden Markov models,Training,Feature extraction,Error analysis,Computational modeling,Human factors
AI 理解论文
溯源树
样例
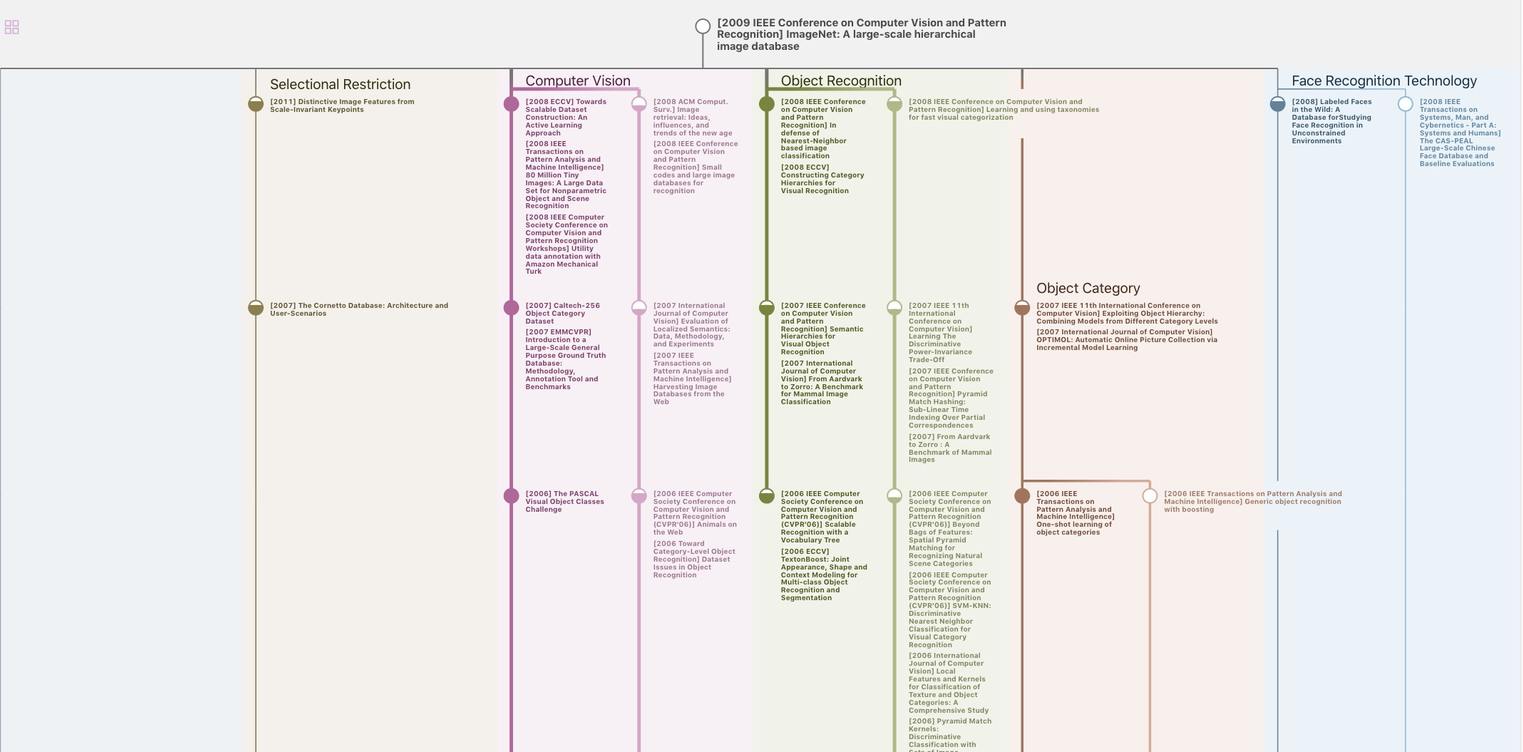
生成溯源树,研究论文发展脉络
Chat Paper
正在生成论文摘要