An Active Learning Approach to Build Adaptive Cost Models for Web Services
Data & knowledge engineering(2019)
摘要
Delivering accurate estimates of query costs in web services is important in different contexts, e.g., to measure their Quality of Service. However, building a reliable cost model is difficult as (i) a web service is a black box often hiding a complex computation, (ii) a call to the same service can yield completely different costs by simply changing a parameter value, and (iii) execution costs can drift with time. In this paper we propose Tiresias, an approach that, given a web service exposing an interface with a fixed number of parameters, initializes and actively adapts a model to accurately predict query costs. The cost model is represented by a regression tree trained through two interleaved querying cycles: a passive one, where the costs measured for user-generated queries are used to update the tree, and an active one, where the service is probed through system-generated queries to cope with drifts in the cost function. Tiresias is finally evaluated in terms of effectiveness and efficiency through a set of experimental tests performed on both real and synthetic datasets.
更多查看译文
关键词
Cost models,Web services,Active learning,Regression trees
AI 理解论文
溯源树
样例
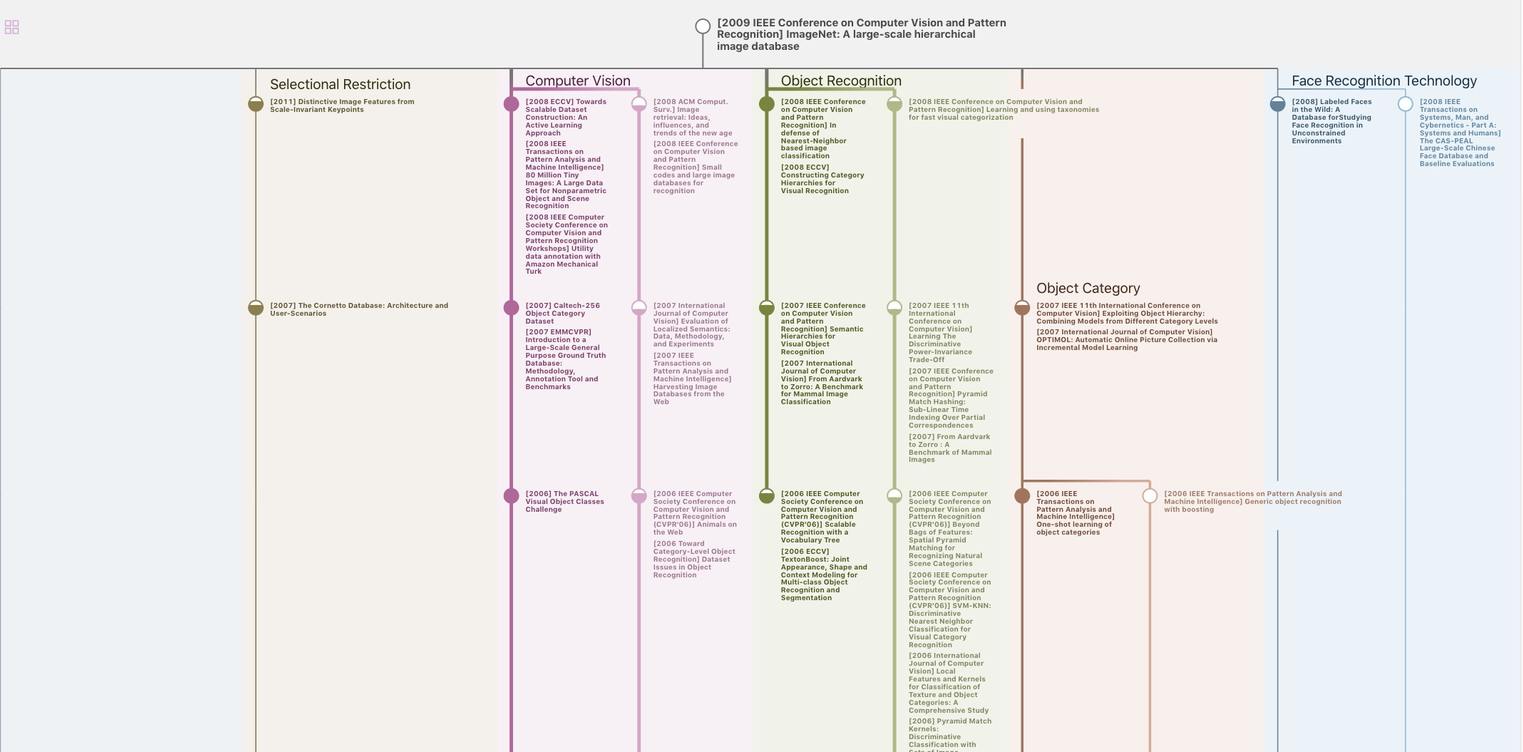
生成溯源树,研究论文发展脉络
Chat Paper
正在生成论文摘要