Impairment Mitigation In Non-Coherent Optical Transmission Enabled With Machine Learning For Intra-Datacenter Networks
Proceedings of SPIE(2017)
摘要
Ever-increasing intra-datacenter traffic will spur the introduction of high-baud rates and high- order modulation formats. Increasing symbol rates and modulation levels decreases tolerance against transmission impairment that includes chromatic dispersion. Transmission distance in warehouse-scale datacenterscan be several kilometers, and then management of chromatic dispersion is necessary. Dispersion-compensating fibers are widely deployed in backbone networks, however, applying them in datacenters is not cost-effective since wavelength channels are coarsely multiplexed. In digital coherent systems, signal distortion due to chromatic dispersion can be resolved in digital domain; however, it will take long time before coherent systems can be introduced in datacenter networks because of their high cost. In this paper, we propose a novel impairment mitigation method employing machine learning. The proposed method is effective even after non-coherent detection and hence it can be applied to cost-sensitive intra-datacenter networks. The machine learns optimum symbol-decision criteria from a sequence of dispersed training signals, and it discriminates payload signals in accordance with the established decision criteria. With the scheme, the received signals can be demodulated in the presence of large chromatic dispersion. The transmission distance thus can be extended without relying on costly optical dispersion compensation. Since information of transmission links is not a priori required, the proposed scheme can easily be applied to any datacenter network. We conduct transmission experiments using 400-Gbps channels each of which comprises 8-subcarrier 28-Gbaud 4-ary pulse-amplitude-modulation (PAM-4) signals, and confirm the effectiveness of the proposed scheme.
更多查看译文
关键词
Short-reach transmission, machine learning, PAM-4
AI 理解论文
溯源树
样例
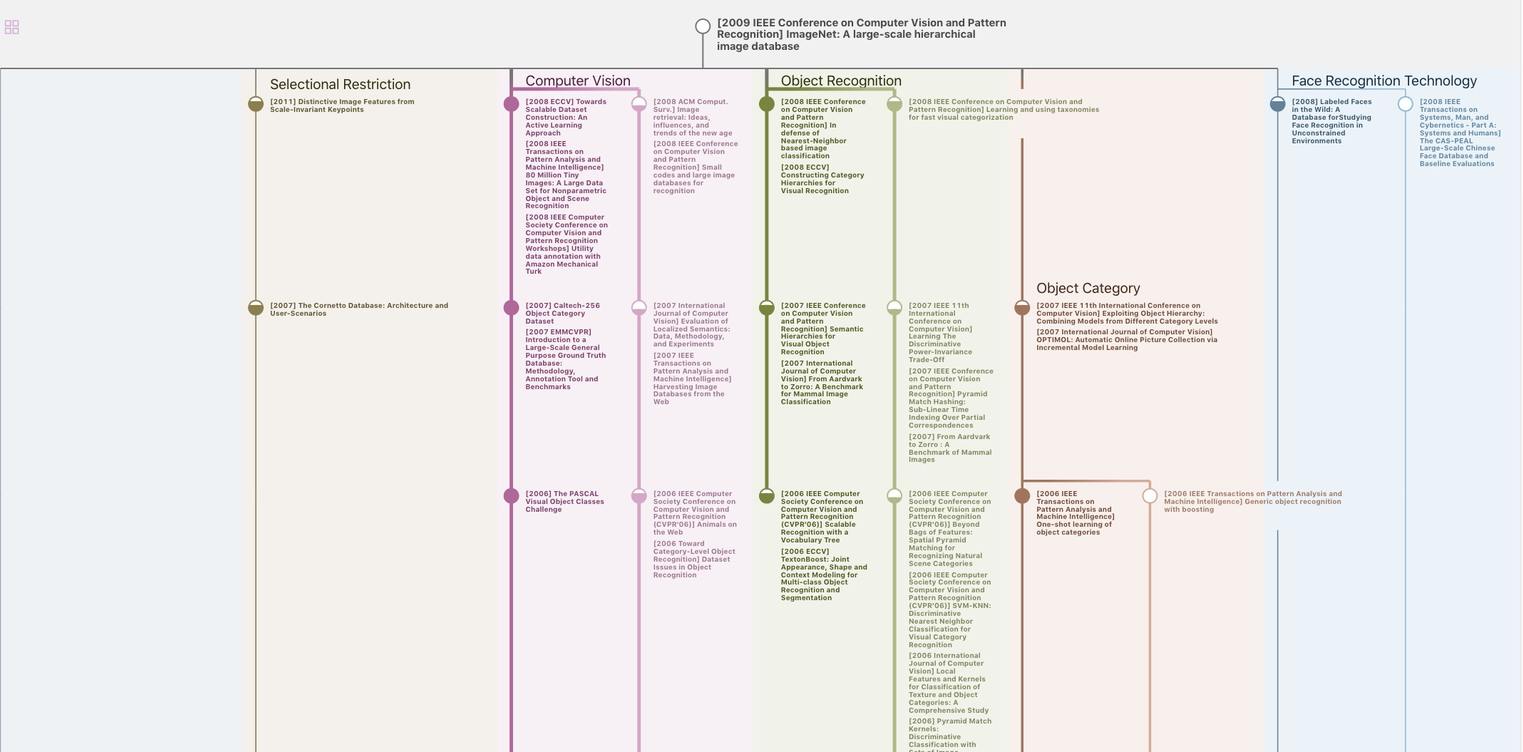
生成溯源树,研究论文发展脉络
Chat Paper
正在生成论文摘要