Dimensionality Reduction and Variable Selection in Multivariate Varying-Coefficient Models With a Large Number of Covariates
JOURNAL OF THE AMERICAN STATISTICAL ASSOCIATION(2018)
摘要
Motivated by the study of gene and environment interactions, we consider a multivariate response varying-coefficient model with a large number of covariates. The need of nonparametrically estimating a large number of coefficient functions given relatively limited data poses a big challenge for fitting such a model. To overcome the challenge, we develop a method that incorporates three ideas: (i) reduce the number of unknown functions to be estimated by using (noncentered) principal components; (ii) approximate the unknown functions by polynomial splines; (iii) apply sparsity-inducing penalization to select relevant covariates. The three ideas are integrated into a penalized least-square framework. Our asymptotic theory shows that the proposed method can consistently identify relevant covariates and can estimate the corresponding coefficient functions with the same convergence rate as when only the relevant variables are included in the model. We also develop a novel computational algorithm to solve the penalized least-square problem by combining proximal algorithms and optimization over Stiefel manifolds. Our method is illustrated using data from Framingham Heart Study.
更多查看译文
关键词
Multivariate regression,Oracle property,Polynomial splines
AI 理解论文
溯源树
样例
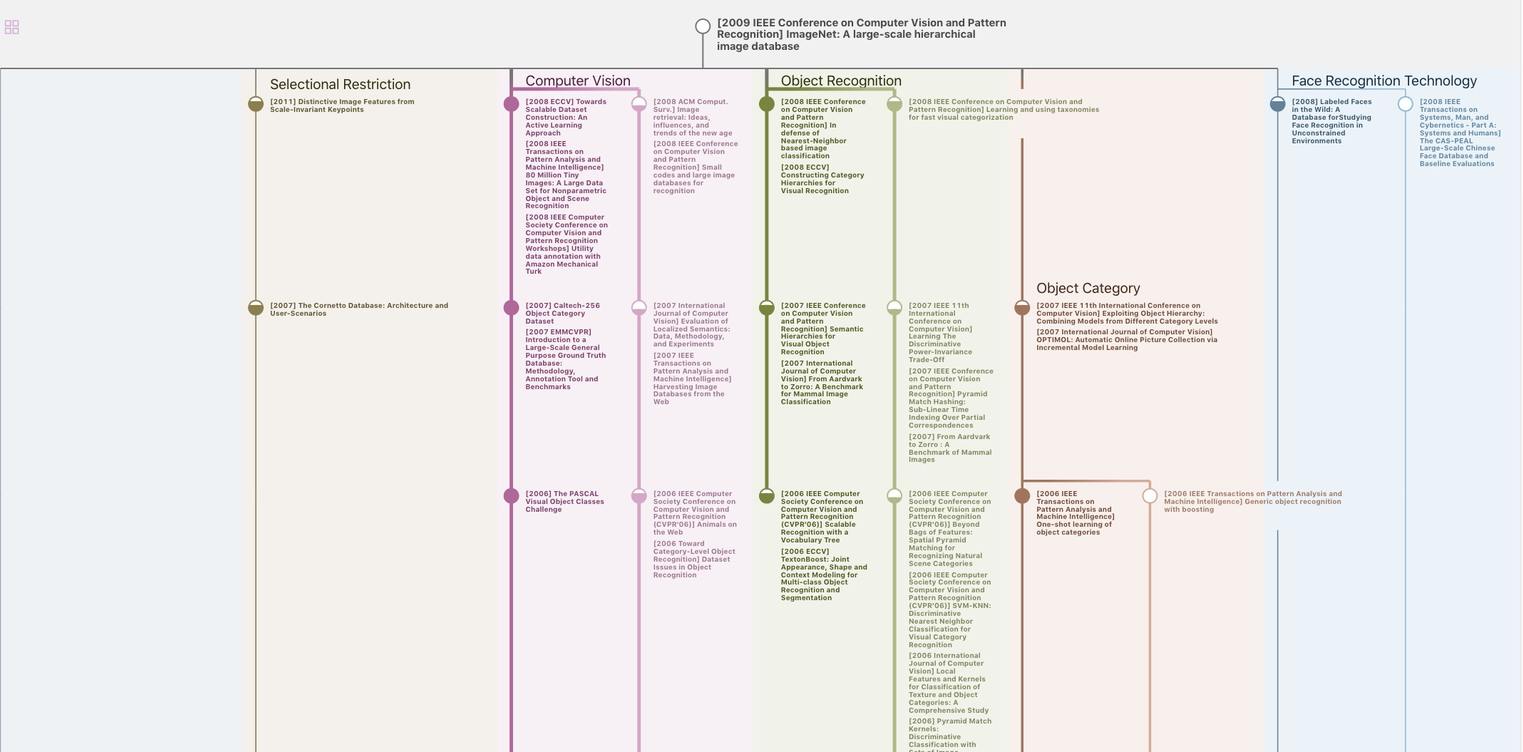
生成溯源树,研究论文发展脉络
Chat Paper
正在生成论文摘要