A New Model in Arabic Text Classification Using BPSO/REP-Tree
Journal of Engineering Research and Technology(2017)
摘要
Specifying an address or placing a specific classification to a page of text is an easy process somewhat, but what if there were many of these pages needed to reach a huge amount of documents. The process becomes difficult and debilitating to the human mind. Automatic text classification is the perfect solution to this problem by identifying a category for each document automatically. This can be achieved by machine learning; by building a model contains all possible attributes features of the text. But with the increase of attributes features, we had to pick the distinguishing features where a model is created to simulate the large amount of attributes (thousands of attributes). To deal with the high dimension of the original dataset, we use features selection process to reduce it by deleting the irrelevant attributes, words, where the rest of features still contain relevant information needed in the process of classification. In this research, a new approach which is Binary Particle Swarm Optimization (BPSO) with Reduced Error Pruning Tree (REP-Tree) is proposed to select the subset of features for Arabic classification process. We compare the proposed approach with two existing approaches; Binary Particle Swarm Optimization BPSO with K-Nearest Neighbor (KNN) and Binary Particle Swarm Optimization BPSO with Support Vector Machine (SVM). After we get the subset of attributes that result from features selection process, we use three common classifiers which are Decision Trees J 48, SVM and the prepared algorithm REP-Tree (as a classifier) to build the classification model. We created our own Arabic dataset; the BBC Arabic News dataset that are collected from the BBC Arabic website and another one existing is used datasets in our experiments, Alkhaleej News Dataset. Finally, we present the experimental results and showed that the proposed algorithm is missionary in this area of research.
更多查看译文
AI 理解论文
溯源树
样例
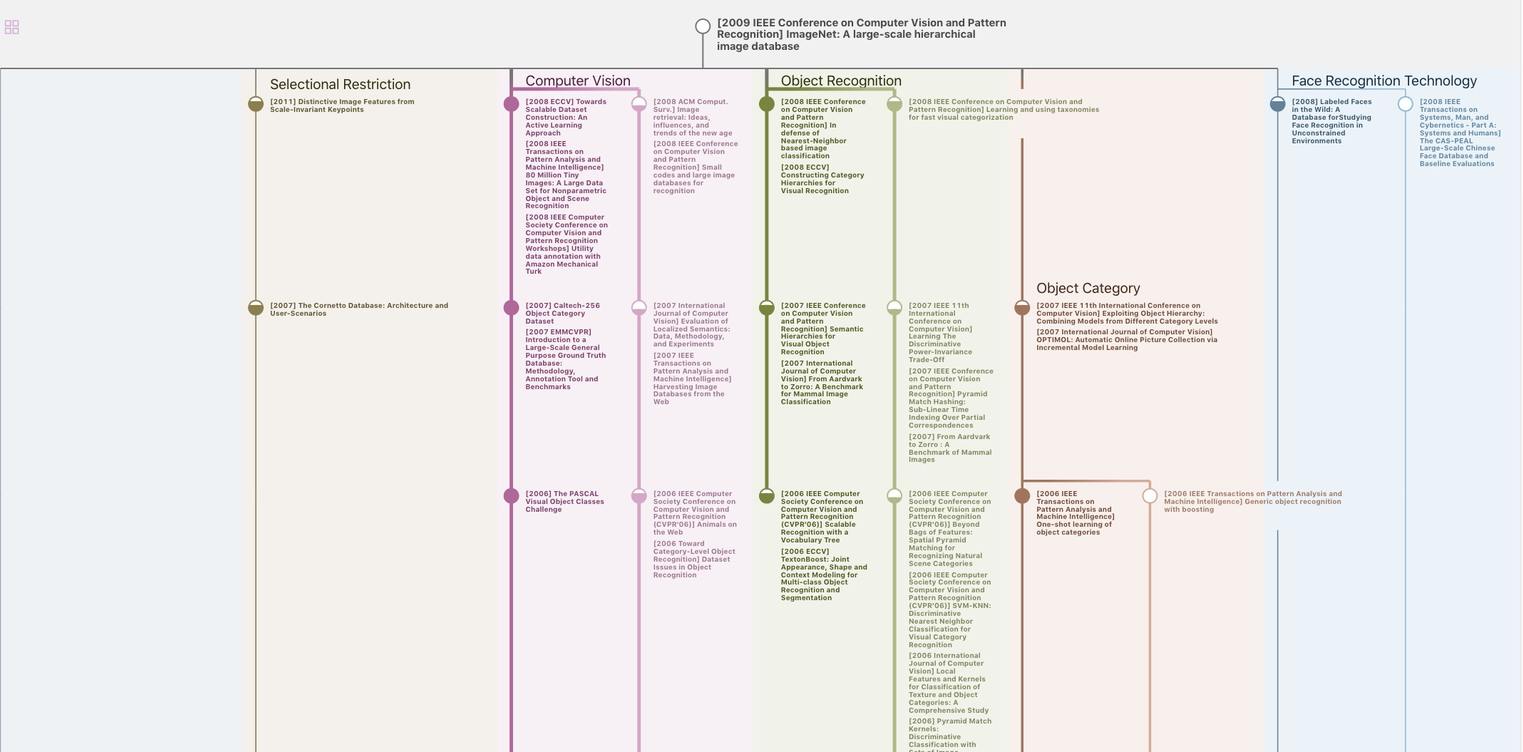
生成溯源树,研究论文发展脉络
Chat Paper
正在生成论文摘要