Joint Entity Recognition and Linking in Technical Domains Using Undirected Probabilistic Graphical Models.
Lecture Notes in Artificial Intelligence(2017)
摘要
The problems of recognizing mentions of entities in texts and linking them to unique knowledge base identifiers have received considerable attention in recent years. In this paper we present a probabilistic system based on undirected graphical models that jointly addresses both the entity recognition and the linking task. Our framework considers the span of mentions of entities as well as the corresponding knowledge base identifier as random variables and models the joint assignment using a factorized distribution. We show that our approach can be easily applied to different technical domains by merely exchanging the underlying ontology. On the task of recognizing and linking disease names, we show that our approach outperforms the state-of-the-art systems DNorm and TaggerOne, as well as two strong lexicon-based baselines. On the task of recognizing and linking chemical names, our system achieves comparable performance to the state-of-the-art.
更多查看译文
关键词
Joint entity recognition and linking,Undirected probabilistic graphical models,Diseases,Chemicals
AI 理解论文
溯源树
样例
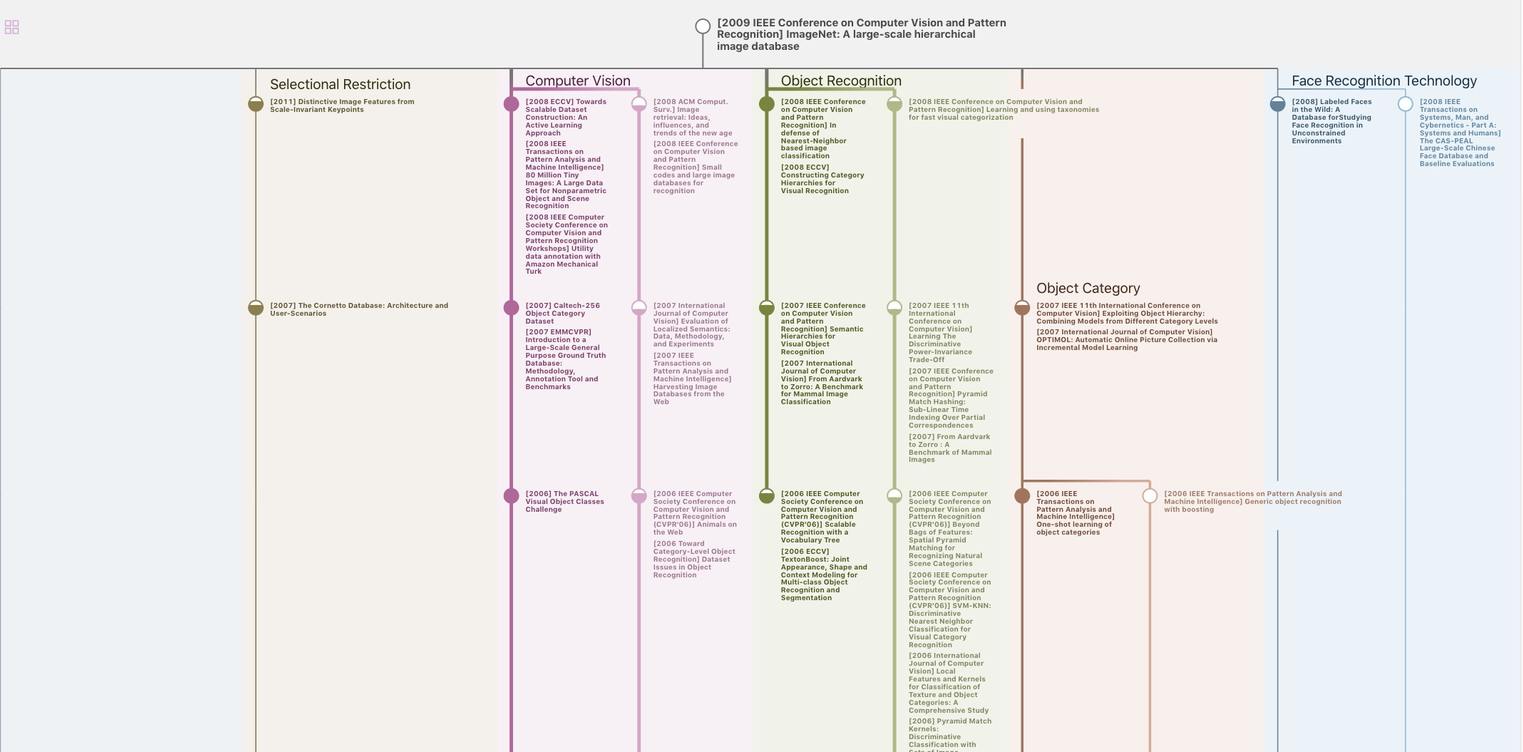
生成溯源树,研究论文发展脉络
Chat Paper
正在生成论文摘要