Deep Matching and Validation Network - An End-to-End Solution to Constrained Image Splicing Localization and Detection.
MM '17: ACM Multimedia Conference Mountain View California USA October, 2017(2017)
摘要
Image splicing is a very common image manipulation technique that is sometimes used for malicious purposes. A splicing detection and localization algorithm usually takes an input image and produces a binary decision indicating whether the input image has been manipulated, and also a segmentation mask that corresponds to the spliced region. Most existing splicing detection and localization pipelines suffer from two main shortcomings: 1) they use handcrafted features that are not robust against subsequent processing (e.g., compression), and 2) each stage of the pipeline is usually optimized independently. In this paper we extend the formulation of the underlying splicing problem to consider two input images, a query image and a potential donor image. Here the task is to estimate the probability that the donor image has been used to splice the query image, and obtain the splicing masks for both the query and donor images. We introduce a novel deep convolutional neural network architecture, called Deep Matching and Validation Network (DMVN), which simultaneously localizes and detects image splicing. The proposed approach does not depend on handcrafted features and uses raw input images to create deep learned representations. Furthermore, the DMVN is end-to-end optimized to produce the probability estimates and the segmentation masks. Our extensive experiments demonstrate that this approach outperforms state-of-the-art splicing detection methods by a large margin in terms of both AUC score and speed.
更多查看译文
关键词
image forensics, splicing detection and localization, deep learning
AI 理解论文
溯源树
样例
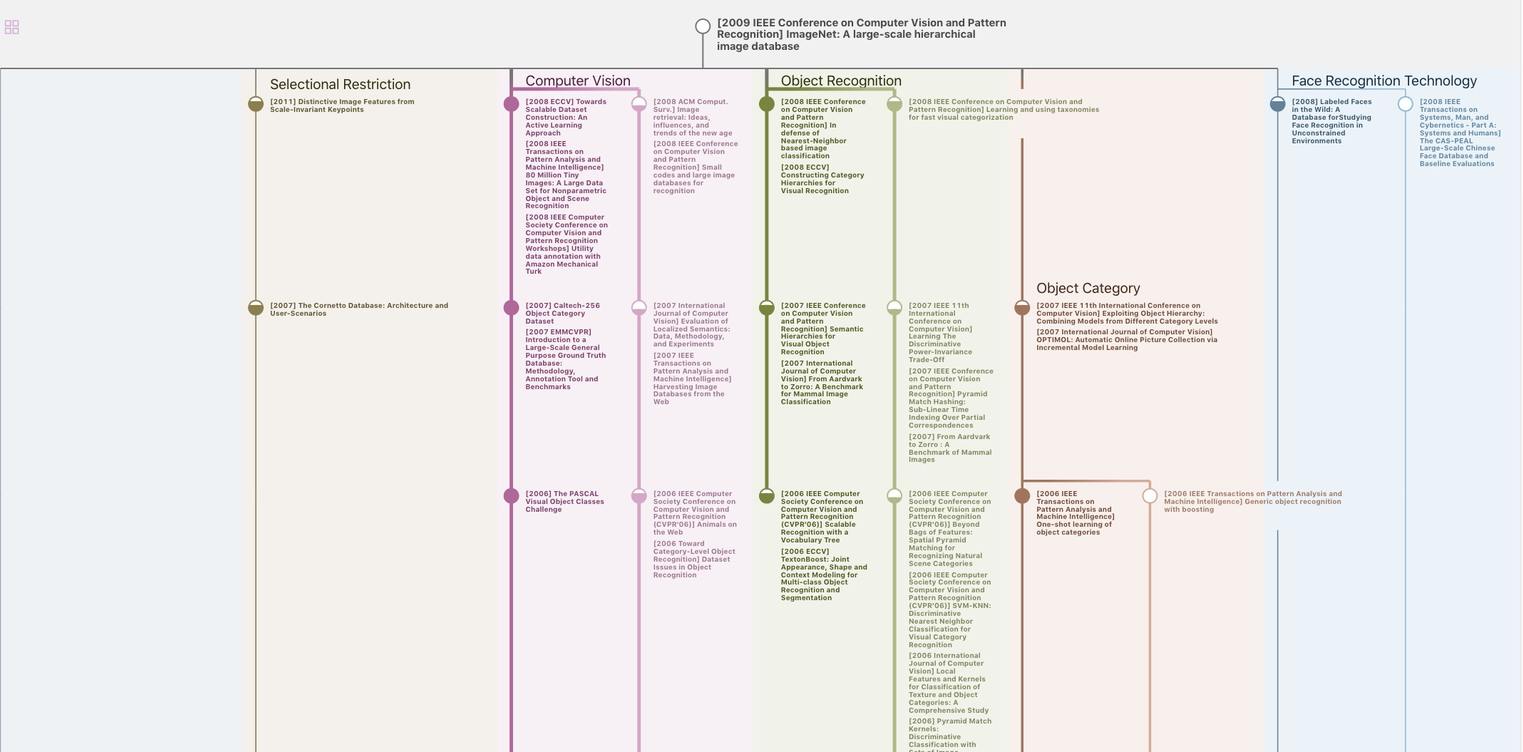
生成溯源树,研究论文发展脉络
Chat Paper
正在生成论文摘要