When Deep Meets Shallow: Subspace-Based Multi-View Fusion for Instance-Level Image Retrieval
2018 IEEE International Conference on Robotics and Biomimetics (ROBIO)(2018)
摘要
Instance-level image retrieval, which aims to find all the images correctly matching the query instance, has attracted extensive attention in the community of computer vision and multimedia. The success of recent retrieval systems largely depends on an image signature with sufficient discriminative power for visual representation. For the past few years, massive feature learning algorithms have sprung up, and they have significantly advanced the development of instance retrieval. In particular, CNN features derived from a deep neural network allows encoding high-level image contents and demonstrates unrivaled superiority to the hand-crafted shallow image signatures. However, the shallow features still play a beneficial role in visual matching particularly when dramatic variances in viewpoint and scale are present, since they inherit certain invariance from the local robust descriptor, e.g. SIFT. Thus, it is important to leverage the complementarity between these two heterogeneous signatures for multi-view fusion in instance retrieval. Since it is still an open problem, in this paper, we propose a subspace-based multi-view fusion strategy where a shared subspace is uncovered from the original high-dimensional features yielding a compact latent representation. Since our method works in an unsupervised fashion without any training process involved, it lends itself to various real-time robotic vision tasks, e.g. place recognition and scene description. Experiments on public benchmark datasets reveal the proposed method works better than other classic fusion approaches and achieves the state-of-the-art performance.
更多查看译文
关键词
Visualization,Task analysis,Robots,Image retrieval,Real-time systems,Image representation,Image reconstruction
AI 理解论文
溯源树
样例
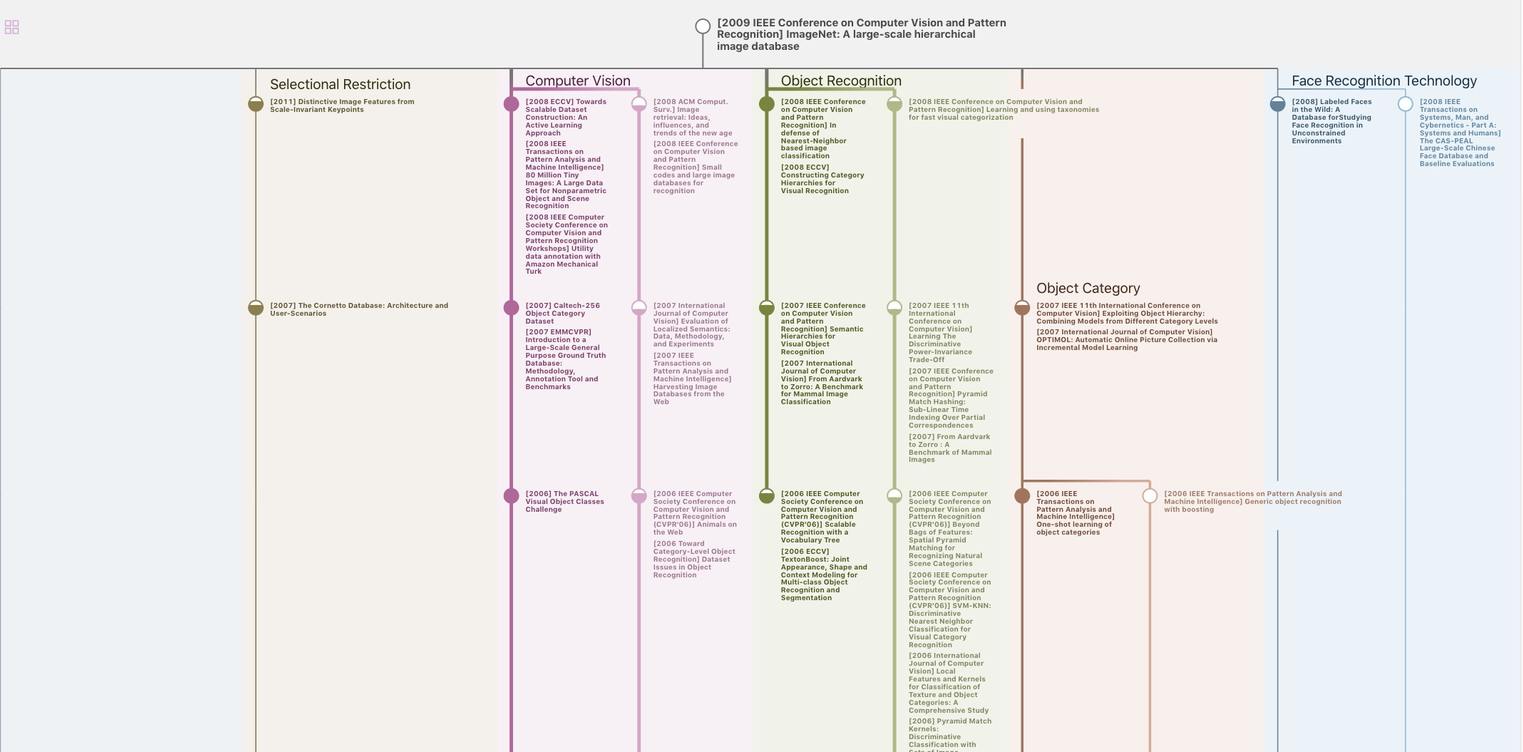
生成溯源树,研究论文发展脉络
Chat Paper
正在生成论文摘要