Tensor Sparsity For Classifying Low-Frequency Ultra-Wideband (Uwb) Sar Imagery
2017 IEEE Radar Conference (RadarConf)(2017)
摘要
Although a lot of progress has been made over the years, one critical challenge still facing low-frequency (UHF to L-band) ultra-wideband (UWB) synthetic aperture radar (SAR) technology is the discrimination of buried and obscured targets of interest from other natural and manmade clutter objects in the scene. The key issues are i) low-resolution SAR imagery for this frequency band, ii) targets of interests being typically small compared to the radar signal wavelengths, iii) targets having low radar cross sections (RCS), and iv) very noisy SAR imagery (e.g. target responses buried in responses from cluttered environment). In this paper, we consider the problem of discriminating and classifying buried targets of interest (buried metal and plastic mines, 155-mm unexploded ordinance left perpendiclicularUXOright perpendiclicular, etc.) from other natural and manmade clutter objects (soda can, rocks, etc.) in the presence of noisy responses from the rough ground surfaces for low-frequency UWB 2-D SAR images. We generalize the traditional sparse representation-based classification (SRC) to a model with capability of using the information of the shared class, and implement multichannel classification problems by exploiting structures of sparse coefficients using various techniques. Here, we employ an electromagnetic (EM) SAR database generated using the finite-difference, time-domain (FDTD) software, which is based on a full-wave computational EM method.
更多查看译文
关键词
SRC,sparse recovery,UWB,SAR,discriminator,classifier
AI 理解论文
溯源树
样例
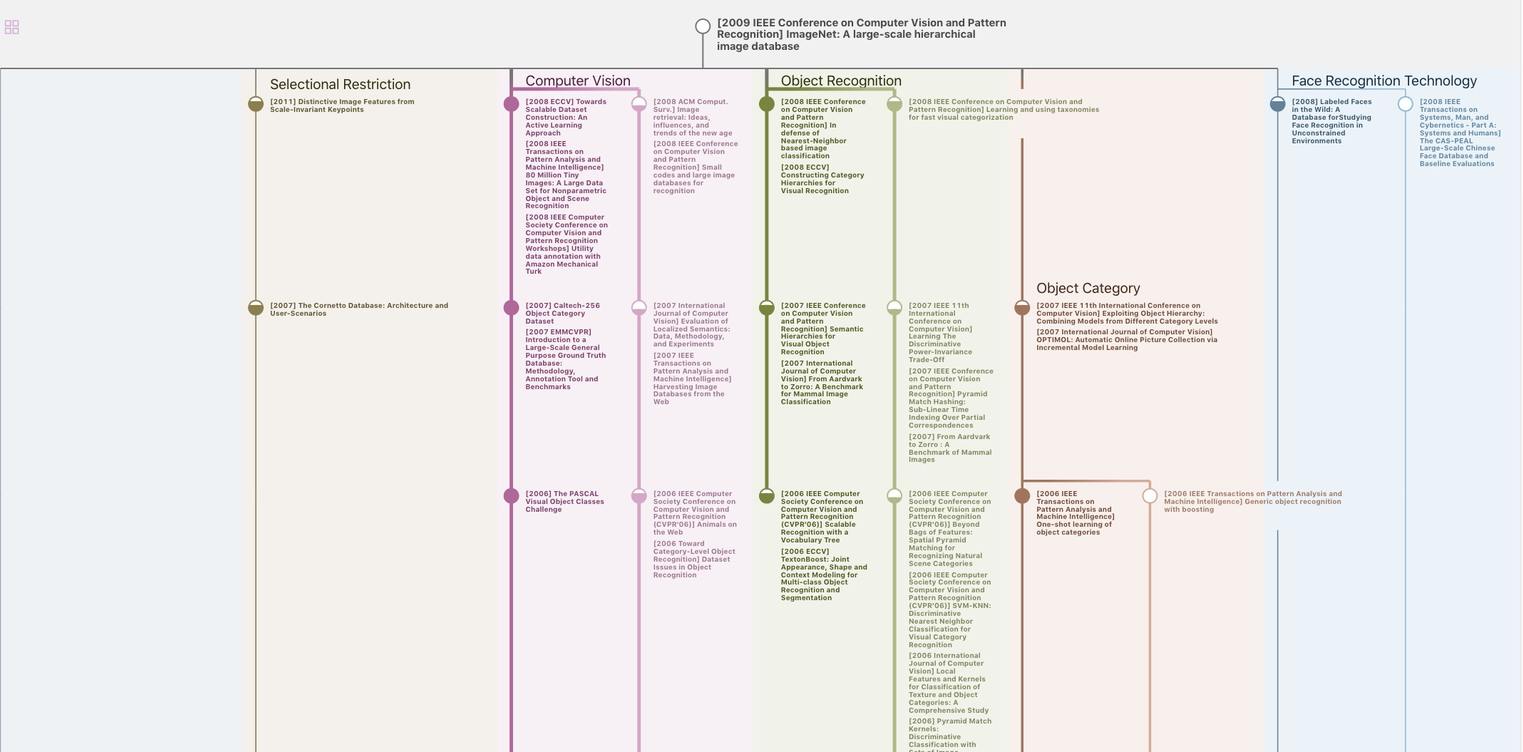
生成溯源树,研究论文发展脉络
Chat Paper
正在生成论文摘要