Radiation reduction of minimally invasive transforaminal lumbar interbody fusion with localisation system in overweight patients: practical technique.
BONE & JOINT JOURNAL(2017)
摘要
Aims Minimally invasive transforaminal lumbar interbody fusion (MITLIF) has been well validated in overweight and obese patients who are consequently subject to a higher radiation exposure. This prospective multicentre study aimed to investigate the efficacy of a novel lumbar localisation system for MITLIF in overweight patients. Patients and Methods The initial study group consisted of 175 patients. After excluding 49 patients for various reasons, 126 patients were divided into two groups. Those in Group A were treated using the localisation system while those in Group B were treated by conventional means. The primary outcomes were the effective radiation dosage to the surgeon and the exposure time. Results There were 62 patients in Group A and 64 in Group B. The mean effective dosage was 0.0217 mSv (standard deviation (SD) 0.0079) in Group A and 0.0383 mSv (SD 0.0104) in Group B (p < 0.001). The mean fluoroscopy exposure time was 26.42 seconds (SD 5.91) in Group A and 40.67 seconds (SD 8.18) in Group B (p < 0.001). The operating time was 175.56 minutes (SD 32.23) and 206.08 minutes (SD 30.15) (p < 0.001), respectively. The mean pre-operative localisation time was 4.73 minutes (SD 0.84) in Group A and 7.03 minutes (SD 1.51) in Group B (p < 0.001). The mean screw placement time was 47.37 minutes (SD 10.43) in Group A and 67.86 minutes (SD 14.15) in Group B (p < 0.001). The pedicle screw violation rate was 0.35% (one out of 283) in Group A and 2.79% (eight out of 287) in Group B (p = 0.020). Conclusion The study shows that the localisation system can effectively reduce radiation exposure, exposure time, operating time, pre-operative localisation time, and screw placement time in overweight patients undergoing MITLIF.
更多查看译文
关键词
Localisation system,Minimally invasive,Radiation reduction,Transforaminal lumbar interbody fusion
AI 理解论文
溯源树
样例
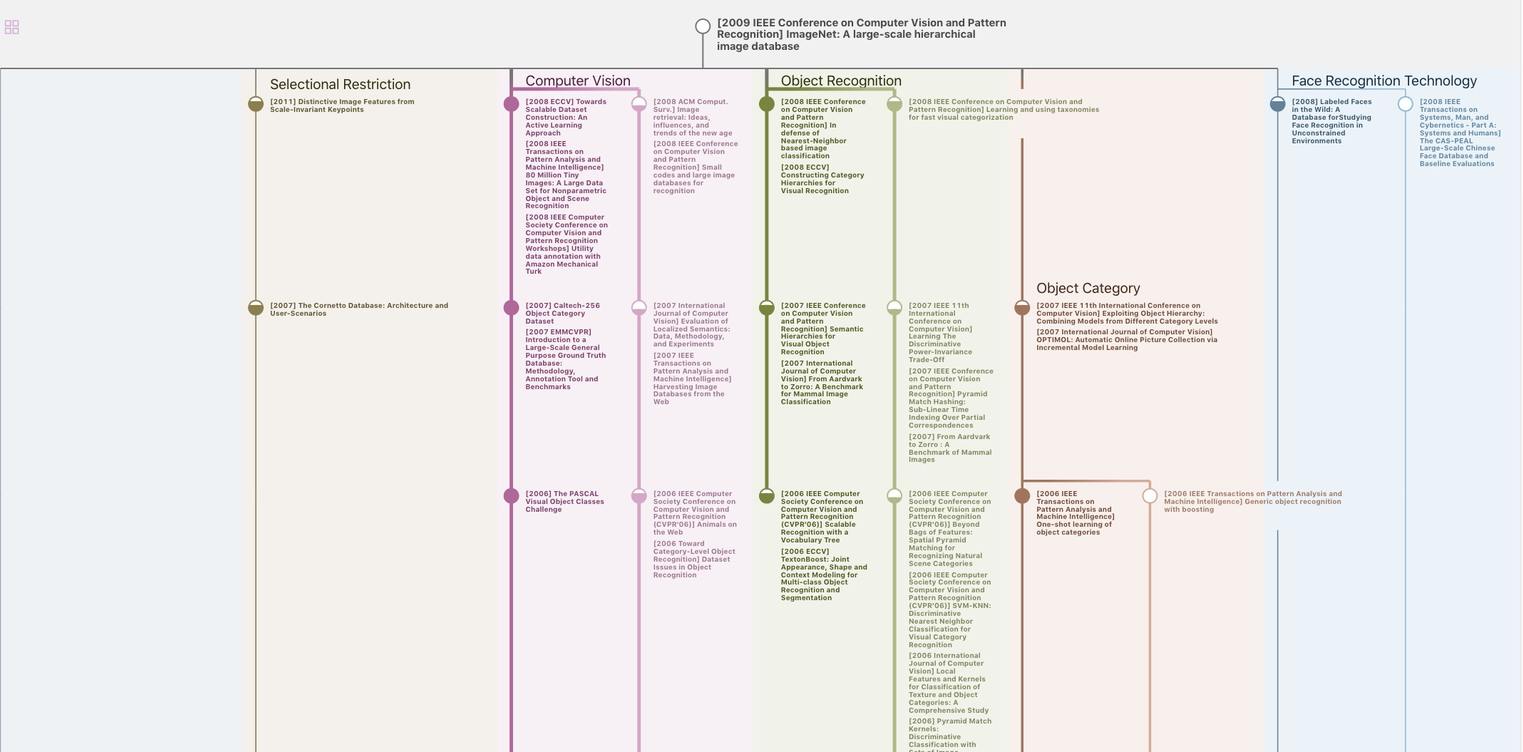
生成溯源树,研究论文发展脉络
Chat Paper
正在生成论文摘要