Environment perception in racing simulators using deep neural networks
2017 International Conference on Optimization of Electrical and Electronic Equipment (OPTIM) & 2017 Intl Aegean Conference on Electrical Machines and Power Electronics (ACEMP)(2017)
摘要
In this paper, we introduce a real-time approach for object recognition, applying the Faster Region-based Convolutional Network (Faster-RCNN) concept in an affordable and open source simulator that can be used to train and test Deep Neural Networks (DNN) models. The objective is to provide the scientific community with a framework where the advantages and disadvantages of already published or new designed architectures and concepts for object detection can be validated. The framework proposes two available configurations for implementing DNN algorithms. Our framework provides an interface to the TORCS racing game simulator, where the deployed methods can be evaluated. Various ground truth information can be extracted from TORCS, like the traffic scene image, car position on the track, speed or distances between the traffic participants.
更多查看译文
关键词
environment perception,real-time approach,object recognition,faster region-based convolutional network,Faster-RCNN,open source simulator,deep neural network model training,deep neural network model testing,DNN models,scientific community,object detection,TORCS racing game simulator,traffic scene image,car position,traffic participant distance
AI 理解论文
溯源树
样例
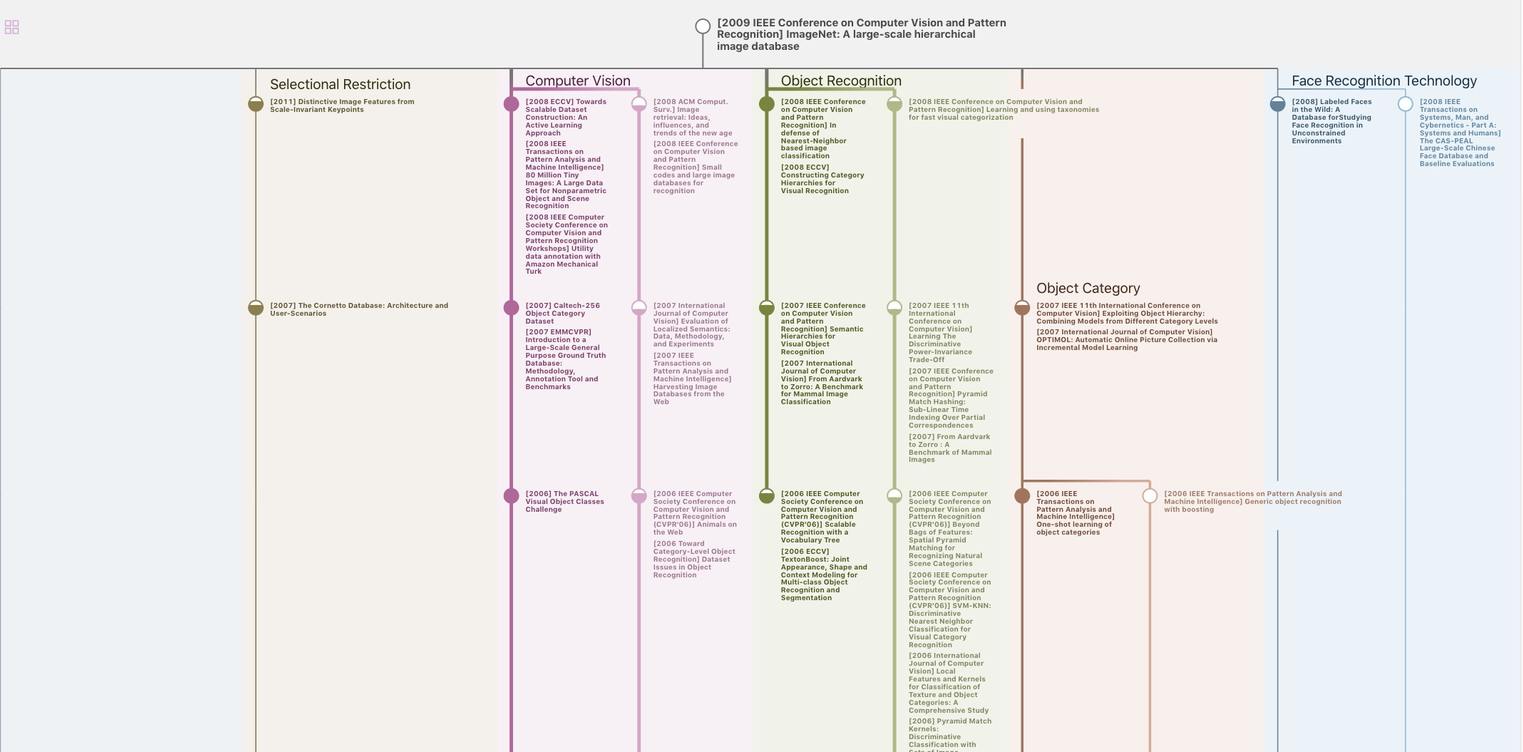
生成溯源树,研究论文发展脉络
Chat Paper
正在生成论文摘要