Towards the ImageNet-CNN of NLP: Pretraining Sentence Encoders with Machine Translation
neural information processing systems(2017)
摘要
Computer vision has benefited from initializing multiple deep layers with weights pre-trained on large supervised training sets like ImageNet. In contrast, deep models for language tasks currently only benefit from transfer of unsupervised word vectors and randomly initialize all higher layers. In this paper, we use the encoder of an attentional sequence-to-sequence model trained for machine translation to initialize models for other, different language tasks. We show that this transfer improves performance over just using word vectors on a wide variety of common NLP tasks: sentiment analysis (SST, IMDb), question classification (QC), entailment (SNLI), and question answering (SQuAD).
更多查看译文
AI 理解论文
溯源树
样例
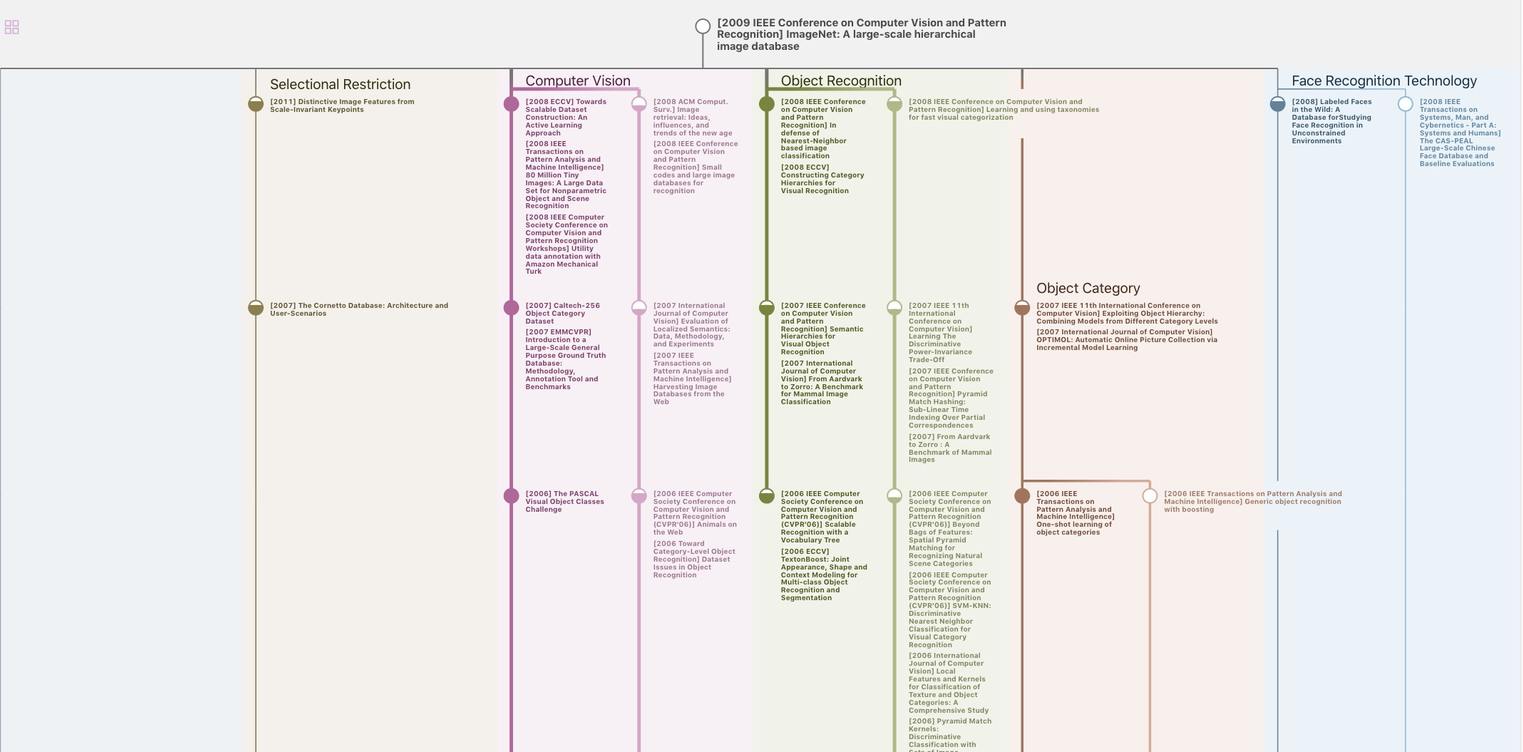
生成溯源树,研究论文发展脉络
Chat Paper
正在生成论文摘要