Convergence Results for Neural Networks via Electrodynamics.
conference on innovations in theoretical computer science(2018)
摘要
We study whether a two neural network can learn another depth two network using gradient descent. Assuming a linear output node, we show that the question of whether gradient descent converges to the target function is equivalent to the following question in electrodynamics: Given k fixed protons in R^d, and k electrons, each moving due to the attractive force from the protons and repulsive force from the remaining electrons, whether at equilibrium all the electrons will be matched up with the protons, up to a permutation. Under the standard electrical force, this follows from the classic Earnshawu0027s theorem. In our setting,the force is determined by the activation function and theinput distribution. Building on this equivalence, we prove theexistence of an activation function such that gradient descent learns at least one of the hidden nodes in the target network. Iterating, we show that gradient descent can be used to learn the entire network one node at a time.
更多查看译文
AI 理解论文
溯源树
样例
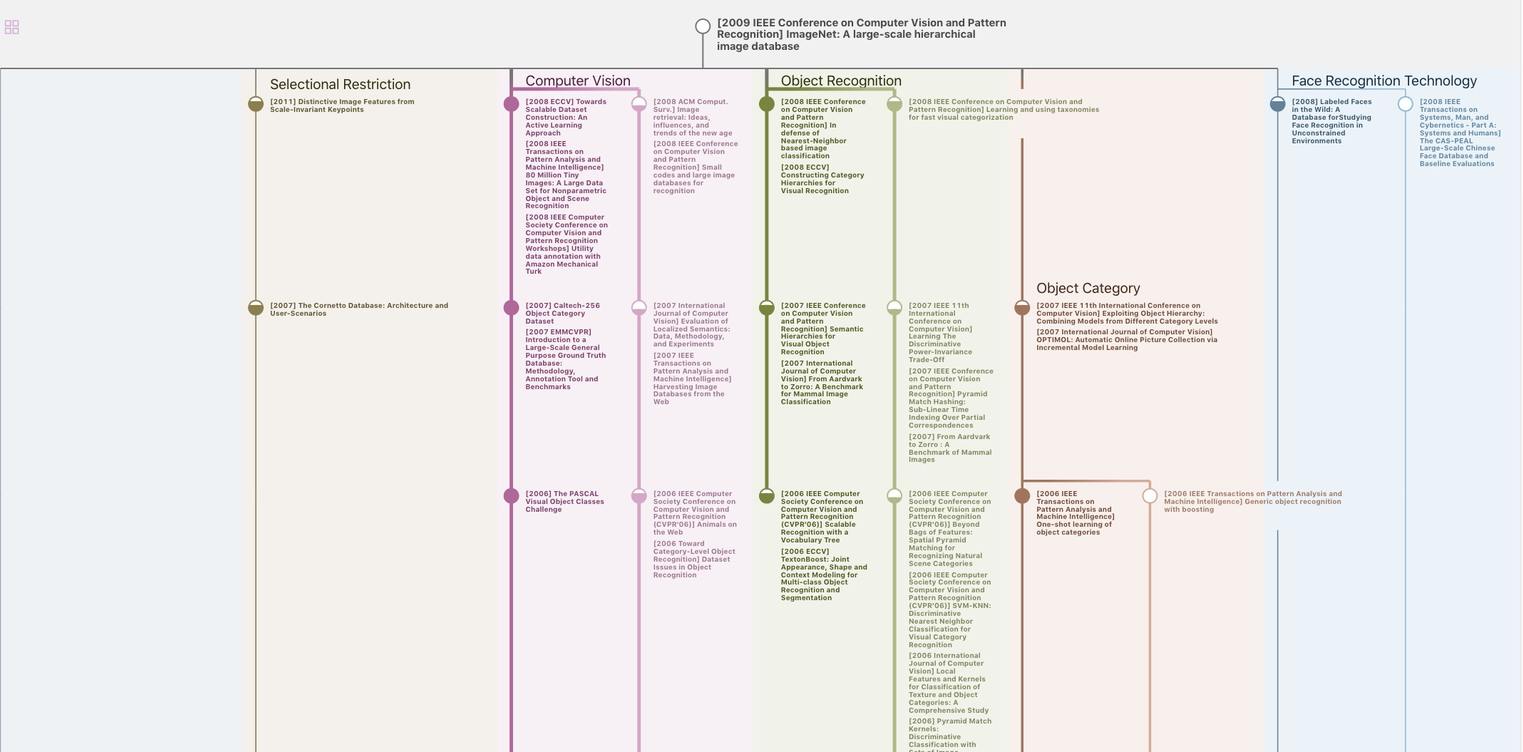
生成溯源树,研究论文发展脉络
Chat Paper
正在生成论文摘要