Image super-resolution using a dilated convolutional neural network.
NEUROCOMPUTING(2018)
摘要
Image super-resolution (SR) has attracted great attention due to its wide practical applications. The objective of SR is to reconstruct high-resolution images from low-resolution ones. By virtue of the great success in computer vision fields achieved by deep learning approach, especially the convolutional neural networks (CNNs), it is a good way to tackle the SR problem using CNNs. In this paper, a 7-layer dilated convolutional neural network (DCNN) with skip-connections is proposed to recover the high-resolution image from the interpolated low-resolution image. Dilated convolutions allow us to arbitrarily control the field-of-view (FOV) of the network. To the best of our knowledge, this is the first study to apply dilated convolution and skip-connections for image super-resolution. We explore different FOV of the network by adjusting the dilation rate and different combination of contextual information using skip-connections to achieve a trade-offbetween performance and speed. We also design a cascade model (CDCNN) to address the different magnification factors problems. Comparisons with state-of-the-art methods, experimental results demonstrate that the proposed model achieves a notable improvement in terms of both quantitative and qualitative measurements. (C) 2017 Elsevier B.V. All rights reserved.
更多查看译文
关键词
Super-resolution,Convolutional neural network,Dilated convolution,Skip-connections
AI 理解论文
溯源树
样例
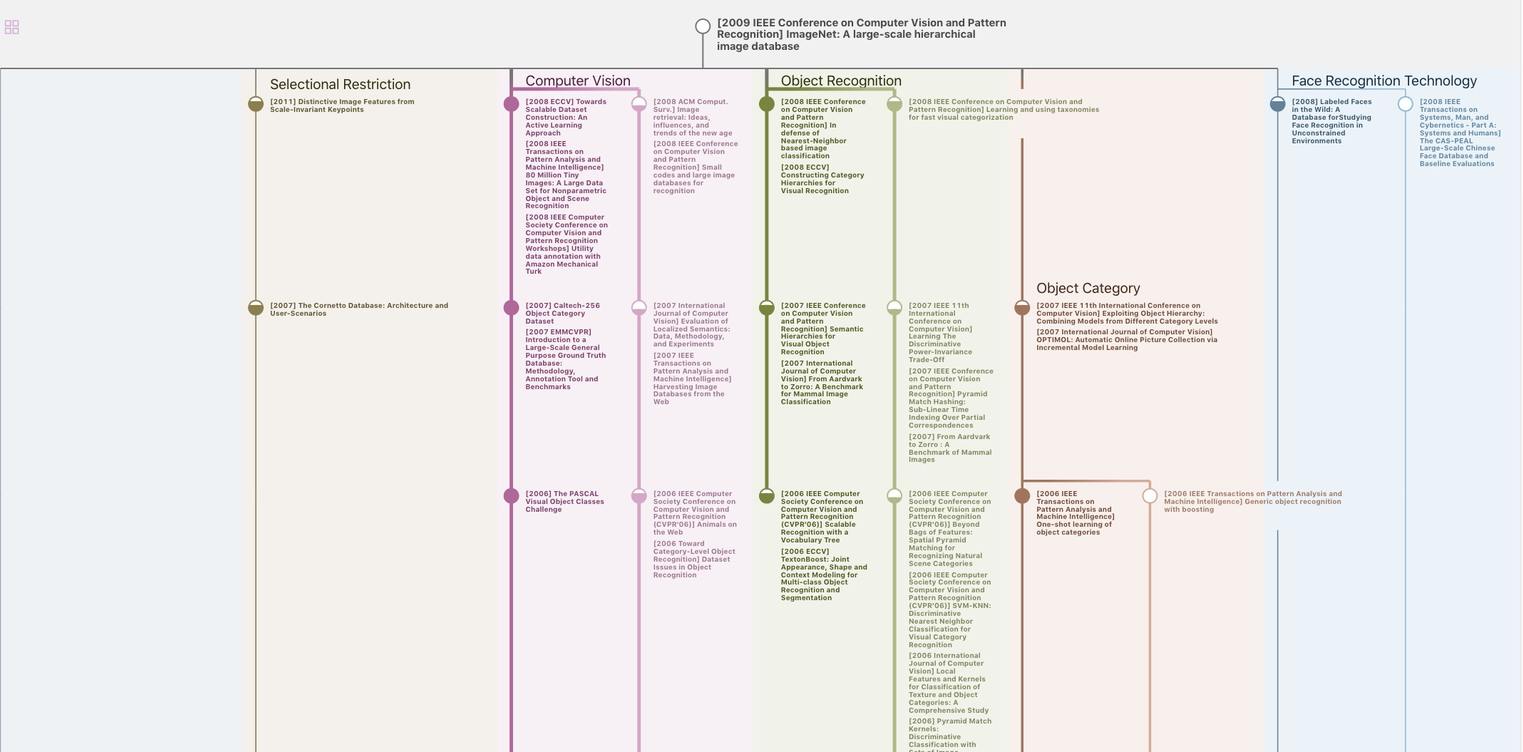
生成溯源树,研究论文发展脉络
Chat Paper
正在生成论文摘要