A Novel Mathematical Framework for Similarity-based Opportunistic Social Networks.
Pervasive and Mobile Computing(2017)
摘要
In this paper we study social networks as an enabling technology for new applications and services leveraging, largely unutilized, opportunistic mobile encounters. More specifically, we quantify mobile user similarity and introduce a novel mathematical framework, grounded in information theory, to characterize fundamental limits and quantify the performance of sample knowledge sharing strategies. First, we introduce generalized, non-temporal and temporal profile structures, beyond geographic location, as a probability mass function. Second, we examine classic and information-theoretic similarity metrics using data in the public domain. A noticeable finding is that temporal metrics give lower similarity indices on the average (i.e., conservative) compared to non-temporal metrics, due to leveraging the wealth of information in the temporal dimension. Third, we introduce a novel mathematical framework that establishes fundamental limits for knowledge sharing among similar opportunistic users. Finally, we show numerical results quantifying the cumulative knowledge gain over time and its upper bound, the knowledge gain limit, using public smartphone data for the user behavior and mobility traces, in the case of fixed as well as mobile scenarios. The presented results provide valuable insights highlighting the key role of the introduced information-theoretic framework in motivating future research along this ripe research direction, studying diverse scenarios as well as novel knowledge sharing strategies.
更多查看译文
关键词
Social networks,Opportunistic,Profiles,Similarity,Modeling,User traces,Numerical results
AI 理解论文
溯源树
样例
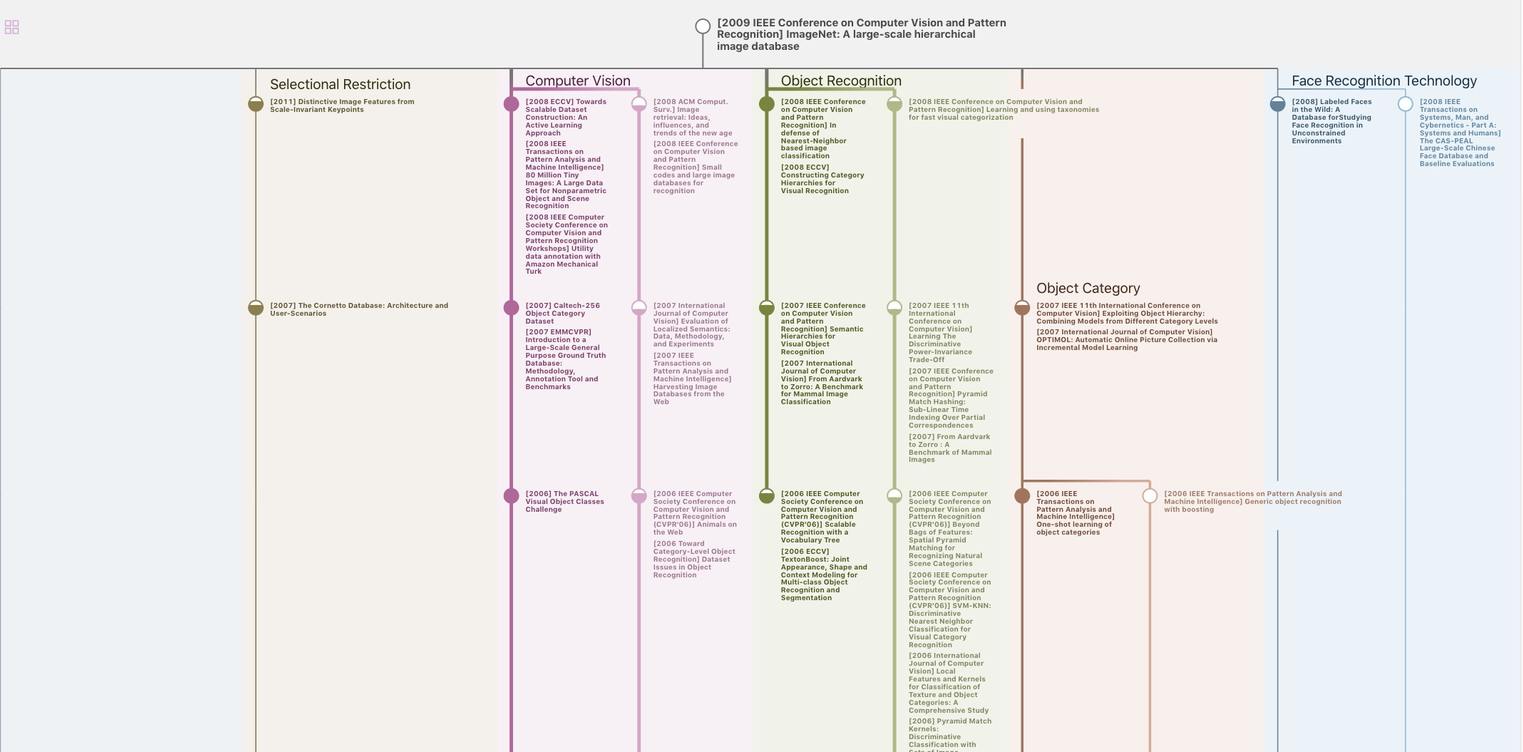
生成溯源树,研究论文发展脉络
Chat Paper
正在生成论文摘要