The Recurrent Temporal Discriminative Restricted Boltzmann Machines.
arXiv: Learning(2017)
摘要
The recurrent temporal restricted Boltzmann machine (RTRBM) has been successful in modelling very high dimensional sequences. However, there exists an unsolved question of how such model can be useful for discrimination tasks. The key issue of this is that learning is difficult due to the intractable log-likelihood. In this paper we propose the discriminative variant of RTRBM. We show that discriminative learning is easier than its generative counterparts where exact gradient can be computed analytically. In the experiments we evaluate RTDRBM on sequence modelling and sequence labelling which outperforms other baselines, notably the recurrent neural networks, hidden Markov models, and conditional random fields. More interestingly, the model is comparable to recurrent neural networks with complex memory gates while requiring less parameters.
更多查看译文
AI 理解论文
溯源树
样例
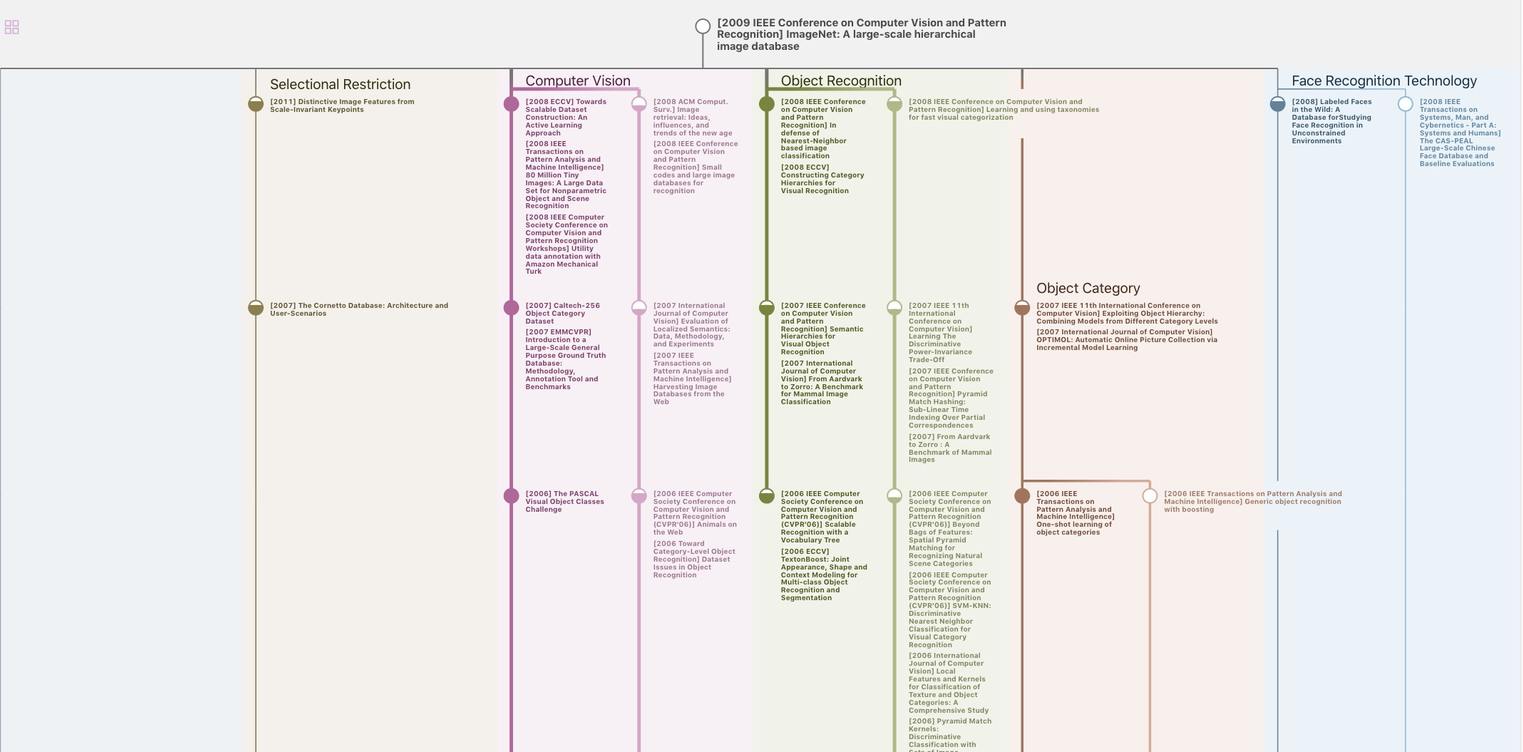
生成溯源树,研究论文发展脉络
Chat Paper
正在生成论文摘要