Limited angle CT reconstruction by simultaneous spatial and Radon domain regularization based on TV and data-driven tight frame
Nuclear Instruments and Methods in Physics Research Section A: Accelerators, Spectrometers, Detectors and Associated Equipment(2018)
摘要
Limited angle computed tomography (CT) reconstruction is widely performed in medical diagnosis and industrial testing because of the size of objects, engine/armor inspection requirements, and limited scan flexibility. Limited angle reconstruction necessitates usage of optimization-based methods that utilize additional sparse priors. However, most of conventional methods solely exploit sparsity priors of spatial domains. When CT projection suffers from serious data deficiency or various noises, obtaining reconstruction images that meet the requirement of quality becomes difficult and challenging. To solve this problem, this paper developed an adaptive reconstruction method for limited angle CT problem. The proposed method simultaneously uses spatial and Radon domain regularization model based on total variation (TV) and data-driven tight frame. Data-driven tight frame being derived from wavelet transformation aims at exploiting sparsity priors of sinogram in Radon domain. Unlike existing works that utilize pre-constructed sparse transformation, the framelets of the data-driven regularization model can be adaptively learned from the latest projection data in the process of iterative reconstruction to provide optimal sparse approximations for given sinogram. At the same time, an effective alternating direction method is designed to solve the simultaneous spatial and Radon domain regularization model. The experiments for both simulation and real data demonstrate that the proposed algorithm shows better performance in artifacts depression and details preservation than the algorithms solely using regularization model of spatial domain. Quantitative evaluations for the results also indicate that the proposed algorithm applying learning strategy performs better than the dual domains algorithms without learning regularization model
更多查看译文
关键词
Computed tomography,Limited angle problem,Simultaneous spatial and Radon domain,Data-driven tight frame
AI 理解论文
溯源树
样例
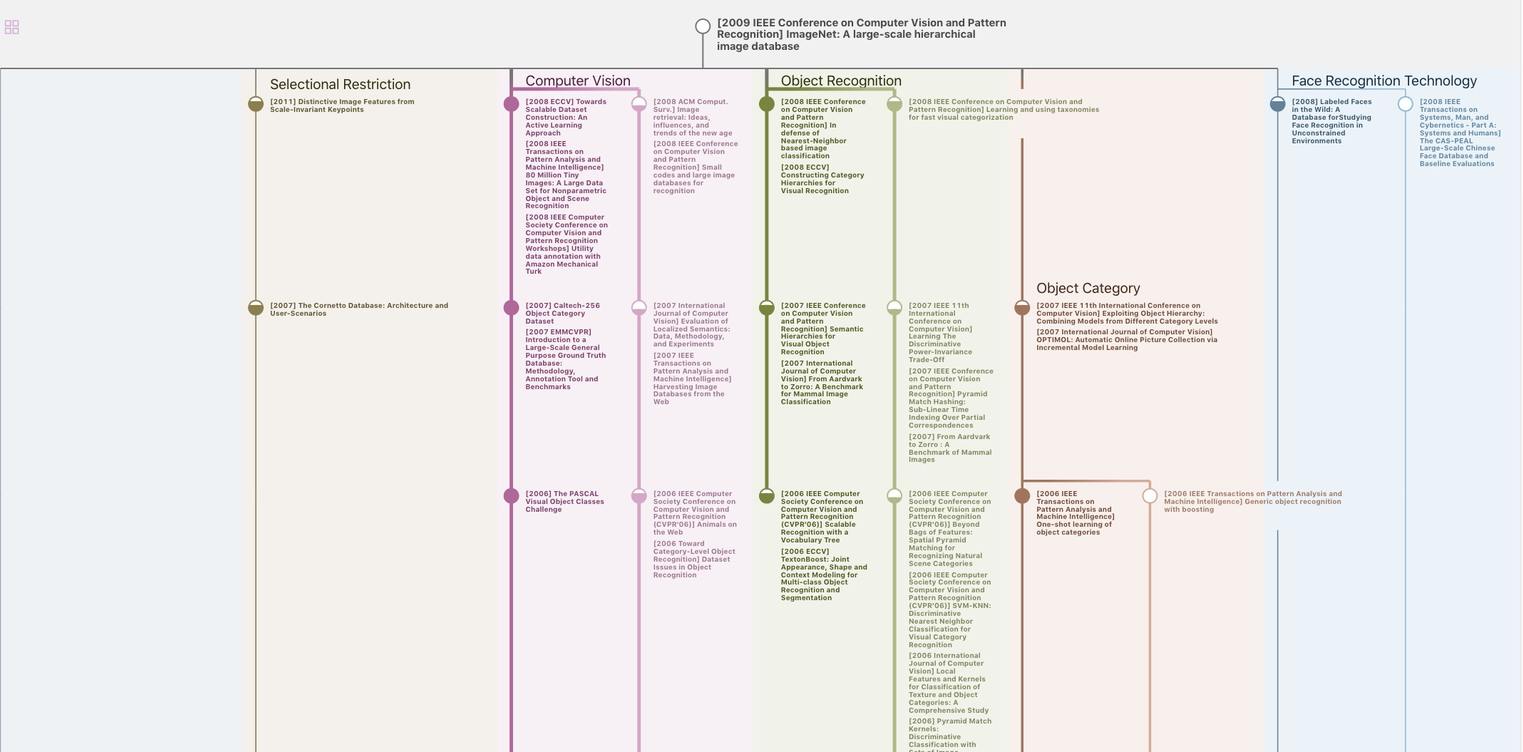
生成溯源树,研究论文发展脉络
Chat Paper
正在生成论文摘要