Optimal Peak Shaving Using Batteries at Datacenters: Characterizing the Risks and Benefits
2017 IEEE 25th International Symposium on Modeling, Analysis, and Simulation of Computer and Telecommunication Systems (MASCOTS)(2017)
摘要
A datacenter's power consumption is a major contributor to its operational expenditures (op-ex) and one-time capital expenditures (cap-ex). The recurring electricity cost is often in large determined by datacenter peak-demand under peak-based pricing which is employed by major electric utility providers. There is a growing interest in reducing a datacenter's electricity costs by using throttling techniques and/or energy storage devices (batteries) which are readily available at most datacenters as a backup energy source. A datacenter's power-demand uncertainty makes this a challenging problem, which is largely neglected in existing work, by assuming perfect predictability of power demand. We model this inherent uncertainty as a Markov chain and also evaluate the risk of over/under charging batteries as a result of the randomness in power demand. We design an online optimization framework for peak shaving which considers Conditional Value at Risk and allows for navigating cost-risk trade-offs of datacenters based on their energy infrastructure and workload characteristics. We show that this framework offers significantly higher (up to 2X) cost-savings with small risks of over/under charging batteries, compared to existing stochastic optimization techniques. This framework leverages Markov Decision Processes to perform online dynamic peak shaving, considering battery degradation costs under peak-based pricing.
更多查看译文
关键词
Peak Shaving,Markov Decision Process,Risk,Battery Cost,Peak-based Pricing
AI 理解论文
溯源树
样例
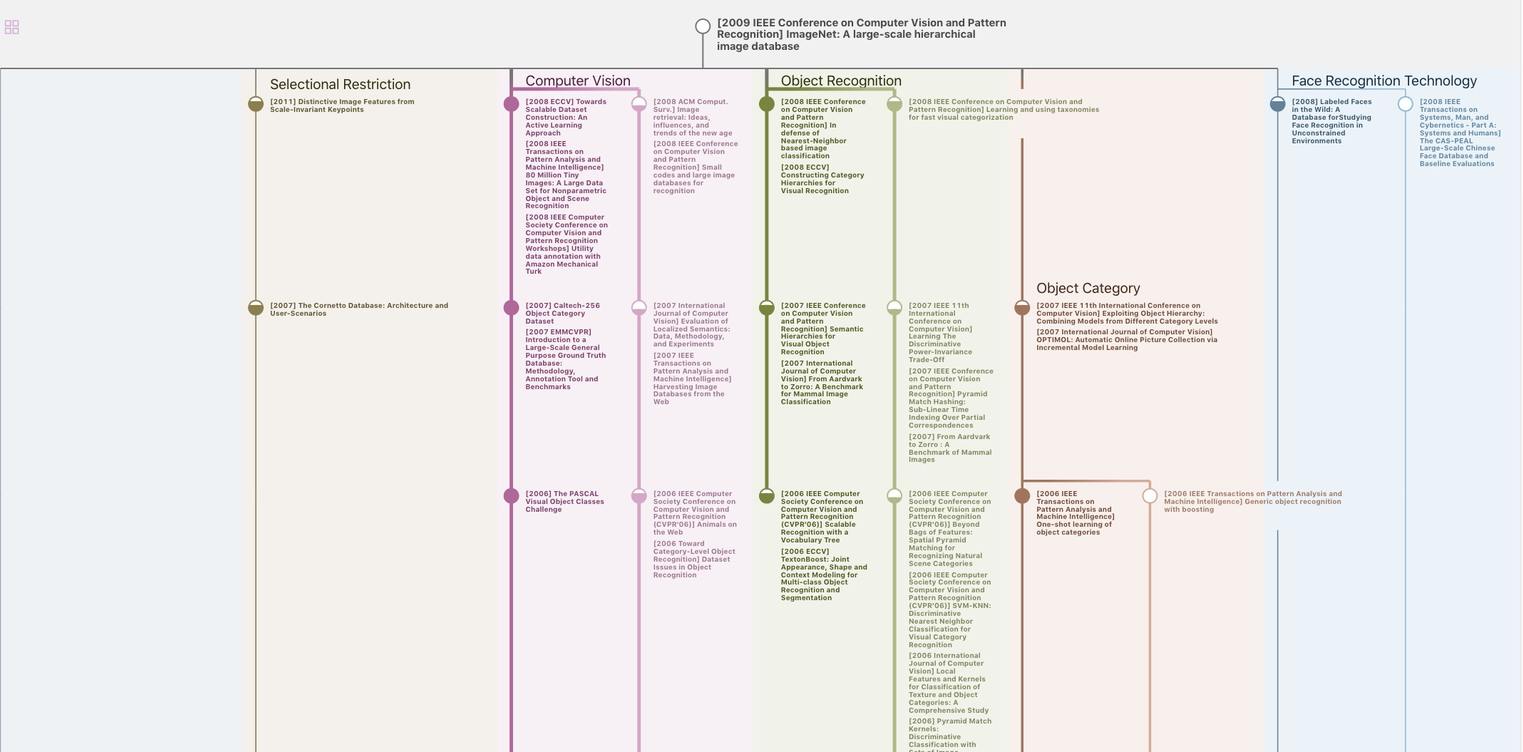
生成溯源树,研究论文发展脉络
Chat Paper
正在生成论文摘要