Short-Term Load Forecasting Using Deep Neural Networks (Dnn)
2017 North American Power Symposium (NAPS)(2017)
摘要
Load forecasting is an important electric utility task for planning resources in Smart grid. This function also aids in predicting the behavior of energy systems in reducing dynamic uncertainties. The efficiency of the entire grid operation depends on accurate load forecasting. This paper proposes and investigates the application of a multi-layered deep neural network to the Iberian electric market (MIBEL) forecasting task. Ninety days of energy demand data are used to train the proposed model. The ninety-day period is treated as a historical dataset to train and predict the demand for day-ahead markets. The network structure is implemented using Google's machine learning Tensor-flow platform. Various combinations of activation functions were tested to achieve a better Mean Absolute percentage error (MAPE) considering the weekday and weekend variations. The tested functions include Sigmoid, Rectifier linear unit (ReLU), and Exponential linear unit (ELU). The preliminary results are promising. and show significant savings in the MAPE values using the ELU function over the other activation functions.
更多查看译文
关键词
Deep learning,Load Forecasting,Neural Network,Tensor flow,Exponential linear activation
AI 理解论文
溯源树
样例
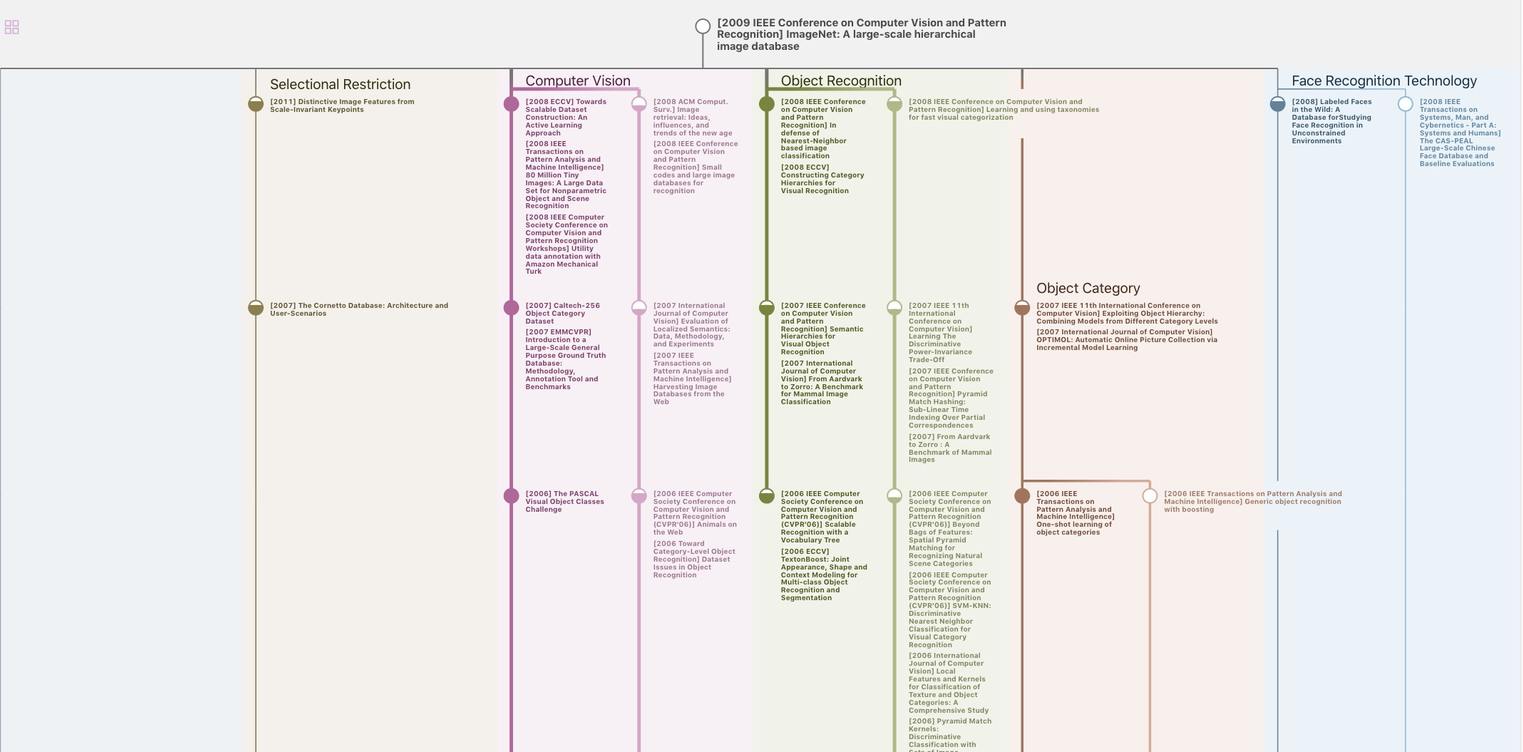
生成溯源树,研究论文发展脉络
Chat Paper
正在生成论文摘要