An empirical assessment of best-answer prediction models in technical Q&A sites
Empirical Software Engineering(2018)
摘要
Technical Q&A sites have become essential for software engineers as they constantly seek help from other experts to solve their work problems. Despite their success, many questions remain unresolved, sometimes because the asker does not acknowledge any helpful answer. In these cases, an information seeker can only browse all the answers within a question thread to assess their quality as potential solutions. We approach this time-consuming problem as a binary-classification task where a best-answer prediction model is built to identify the accepted answer among those within a resolved question thread, and the candidate solutions to those questions that have received answers but are still unresolved. In this paper, we report on a study aimed at assessing 26 best-answer prediction models in two steps. First, we study how models perform when predicting best answers in Stack Overflow, the most popular Q&A site for software engineers. Then, we assess performance in a cross-platform setting where the prediction models are trained on Stack Overflow and tested on other technical Q&A sites. Our findings show that the choice of the classifier and automatied parameter tuning have a large impact on the prediction of the best answer. We also demonstrate that our approach to the best-answer prediction problem is generalizable across technical Q&A sites. Finally, we provide practical recommendations to Q&A platform designers to curate and preserve the crowdsourced knowledge shared through these sites.
更多查看译文
关键词
Cross-platform prediction,Q&a,Stack overflow,Crowdsourcing,Knowledge sharing,Imbalanced datasets
AI 理解论文
溯源树
样例
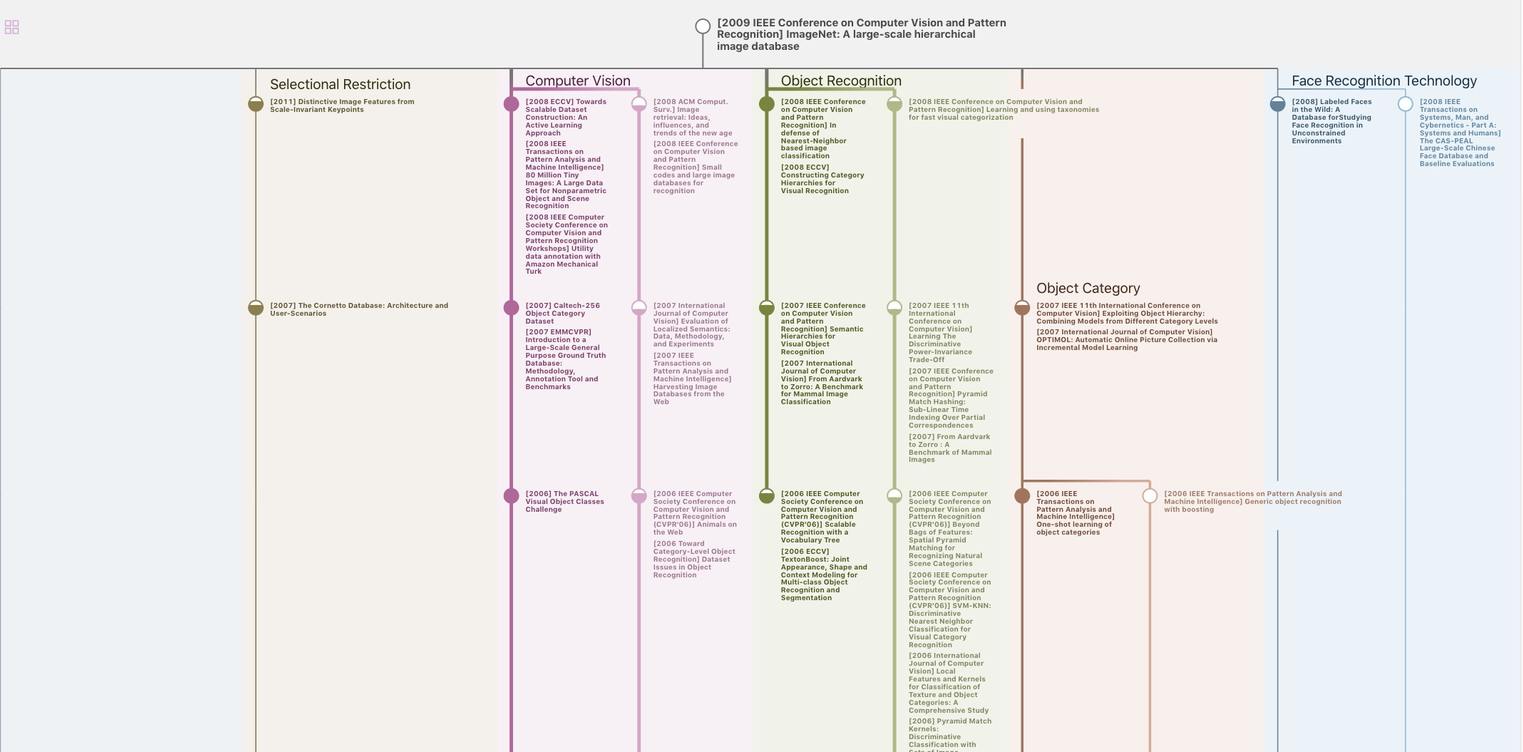
生成溯源树,研究论文发展脉络
Chat Paper
正在生成论文摘要