Two Demonstrators are Better than One - A Social Robot that Learns to Imitate People with Different Interaction Styles
IEEE Transactions on Cognitive and Developmental Systems(2019)
摘要
With recent advances in social robotics, many studies have investigated techniques for learning top-level multimodal interaction logic by imitation from a corpus of human–human interaction examples. Most such studies have taken the approach of learning equally from a variety of demonstrators, with the effect of reproducing a mixture of their average behavior. However, in many scenarios it would be desirable to reproduce specific interaction styles captured from individuals. In this paper, we train one deep neural network jointly on two separate corpuses collected from demonstrators with differing interaction styles. We show that training on both corpuses together improves performance in terms of generating socially appropriate behavior even when reproducing only one of the two styles. Furthermore, the trained neural network also enables us to synthesize new interaction styles on a continuum between the two demonstrated interaction styles. We discuss plots of the hidden layer activations from the neural network, indicating the types of semantic information that appear to be learned by the system. Further, we observe that the better performance with the synthesized corpus is not merely due to the increase of the sample size, as even with the same number of training examples, training on half the data from each corpus provided better performance than training on all the data from a single corpus.
更多查看译文
关键词
Robots,Cameras,Training,Speech,Sensors,Human-robot interaction
AI 理解论文
溯源树
样例
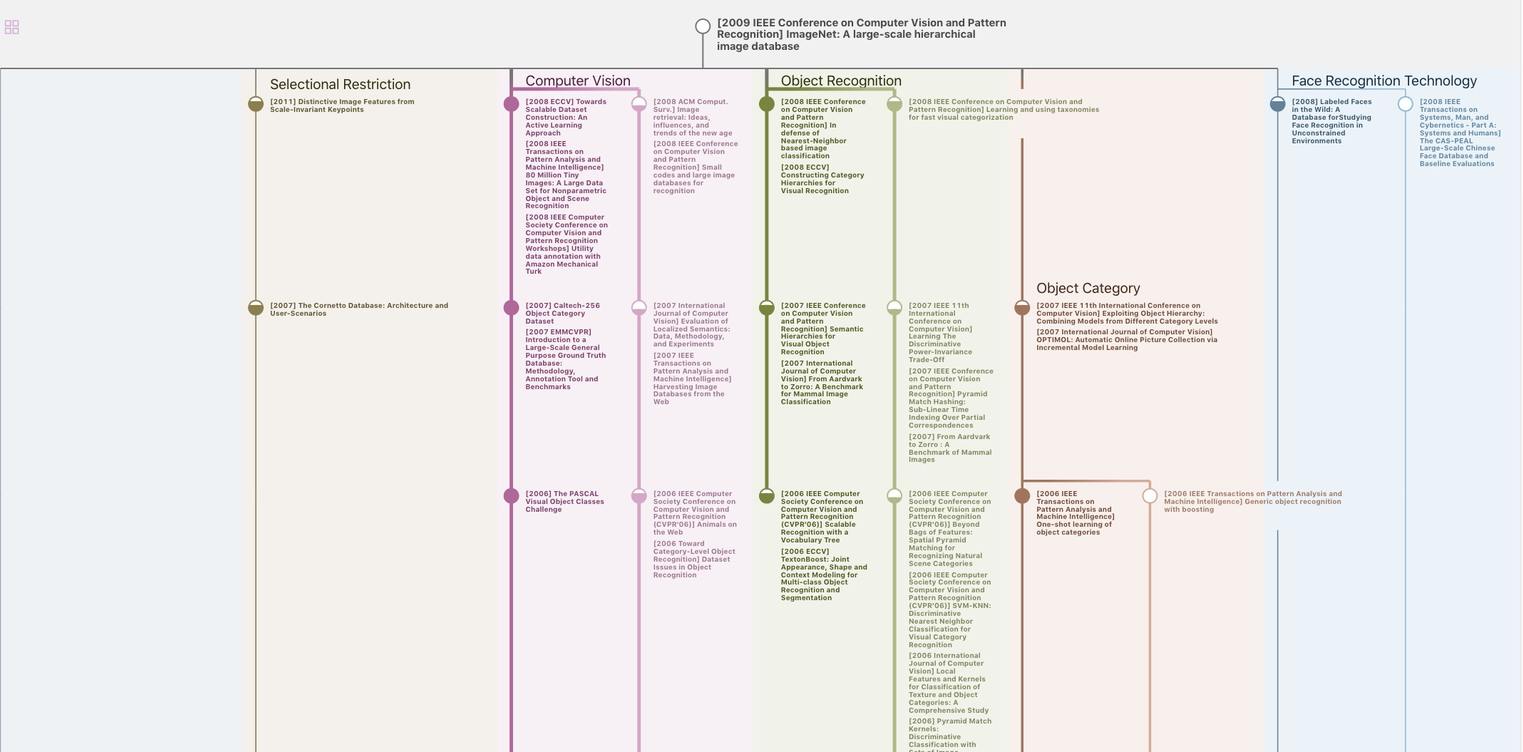
生成溯源树,研究论文发展脉络
Chat Paper
正在生成论文摘要