Feature selection for an SVM based webpage classifier
2017 IEEE 4th International Conference on Soft Computing & Machine Intelligence (ISCMI)(2017)
摘要
Machine-learning techniques are a handy tool for deriving insights from data extracted from the web. Because of the structure of web data extracted by web crawlers there is need for preprocessing the data to extract features that can be used to train a machine learning classifier. The number of available features that can be linked to a website is huge. Narrowing down to a minimum number of features required to drive a classifier has huge benefits. This paper presents a workflow that uses a set of metrics that can be used to reduce the numbers of features for training a support vector machine (SVM) for classifying webpages as fraudulent or not. The paper reports that a three quarter reduction in feature set size only incurs a 5% reduction in classification accuracy which has huge computational benefits.
更多查看译文
关键词
feature extraction,machine learning,web crawling,information,support vector machine
AI 理解论文
溯源树
样例
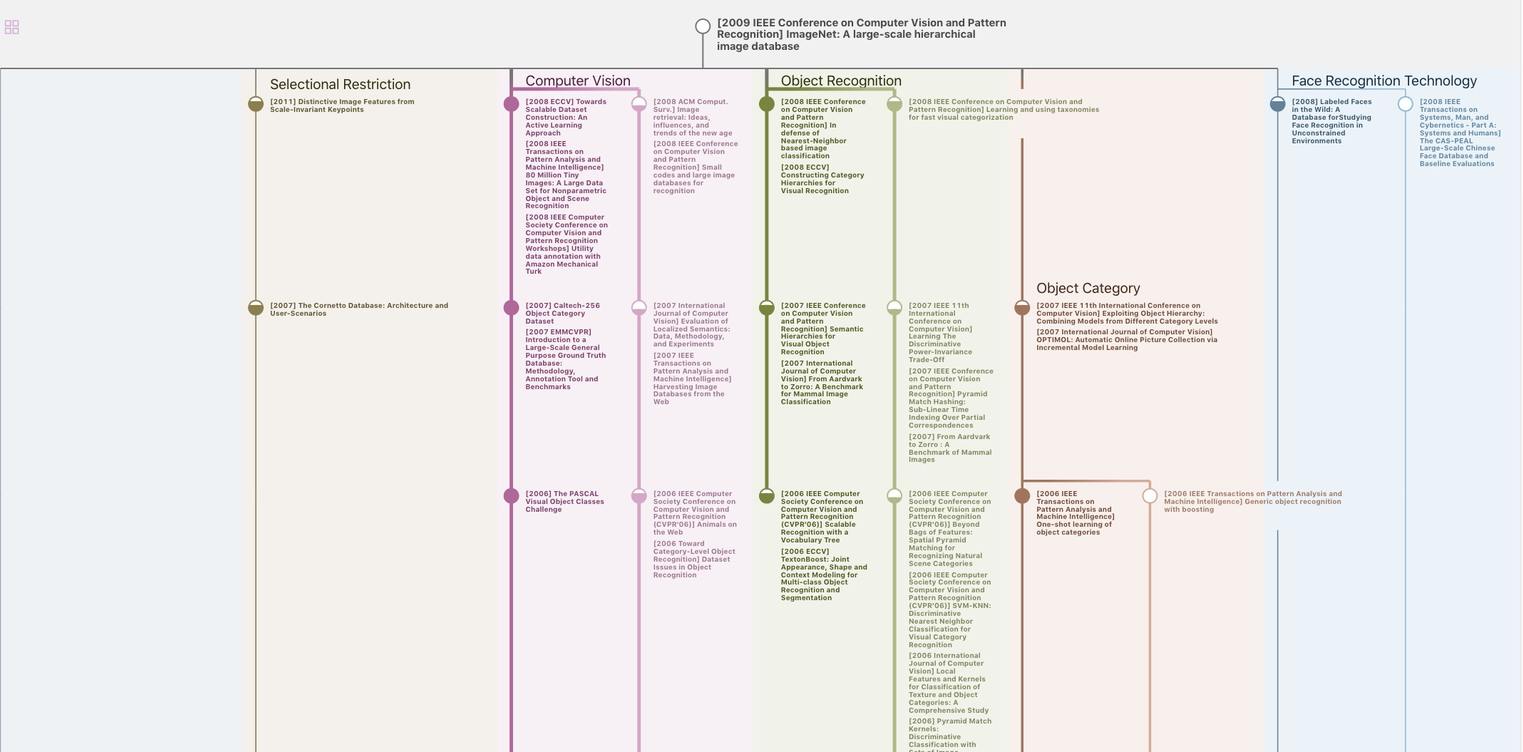
生成溯源树,研究论文发展脉络
Chat Paper
正在生成论文摘要