FPGA implementation of a real-time super-resolution system with a CNN based on a residue number system
2017 International Conference on Field Programmable Technology (ICFPT)(2017)
摘要
A super-resolution technology is used for filling the gap between high-resolution displays and lower-resolution images. One of various algorithms to interpolate the lost information is to use a convolutional neural network (CNN). This paper shows an FPGA implementation and a performance evaluation of our CNN-based super-resolution system, which can process moving images in real time. We apply horizontal and/or vertical flips to input images instead of pre-enlargement. This method prevents information loss and enables the network to make the best use of its input size. In addition, we adopted the residue number system (RNS) to reduce resource utilization. The proposed system can perform super-resolution from 960×540 to 1920×1080 at 60fps with a latency of less than 1ms. In spite of resource restriction of the FPGA, the system generates clear super-resolution images with smooth edges. The evaluation results also revealed the superior quality in terms of the peak signal-to-noise ratio (PSNR), compared to other systems using pre-enlargement.
更多查看译文
关键词
resource utilization reduction,RNS,peak signal-to-noise ratio,clear super-resolution images,input size,information loss,input images,vertical flips,horizontal flips,performance evaluation,convolutional neural network,lost information,lower-resolution images,high-resolution displays,super-resolution technology,residue number system,CNN,real-time super-resolution system,FPGA implementation
AI 理解论文
溯源树
样例
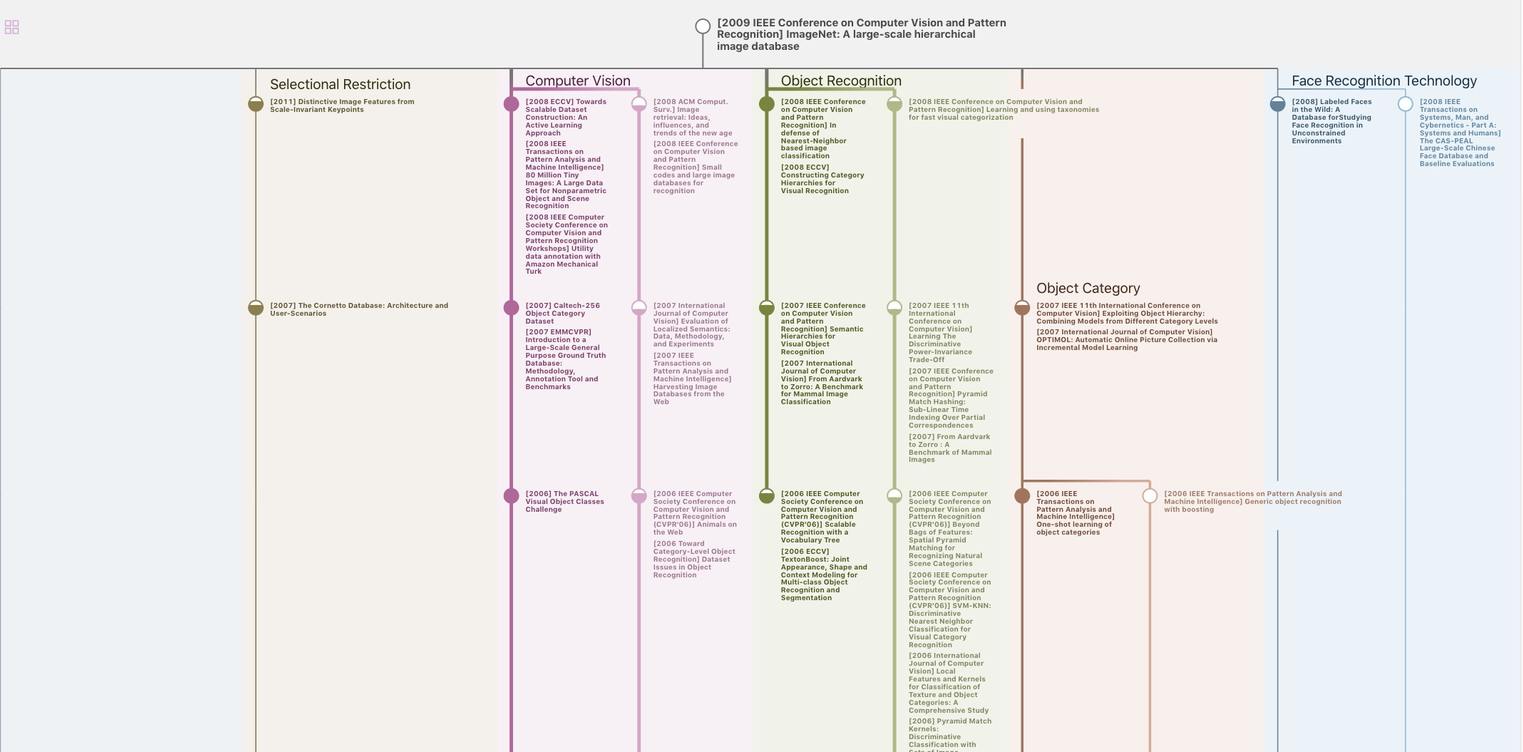
生成溯源树,研究论文发展脉络
Chat Paper
正在生成论文摘要