The Information-Autoencoding Family: A Lagrangian Perspective on Latent Variable Generative Modeling
arXiv: Machine Learning(2018)
摘要
A variety of learning objectives have been recently proposed for training generative models. We show that many of them, including InfoGAN, ALI/BiGAN, ALICE, CycleGAN, VAE, $beta$-VAE, adversarial autoencoders, AVB, and InfoVAE, are Lagrangian duals of the same primal optimization problem. This generalization reveals the implicit modeling trade-offs between flexibility and computational requirements being made by these models. Furthermore, we characterize the class of all objectives that can be optimized under certain computational constraints. Finally, we show how this new Lagrangian perspective can explain undesirable behavior of existing methods and provide new principled solutions.
更多查看译文
AI 理解论文
溯源树
样例
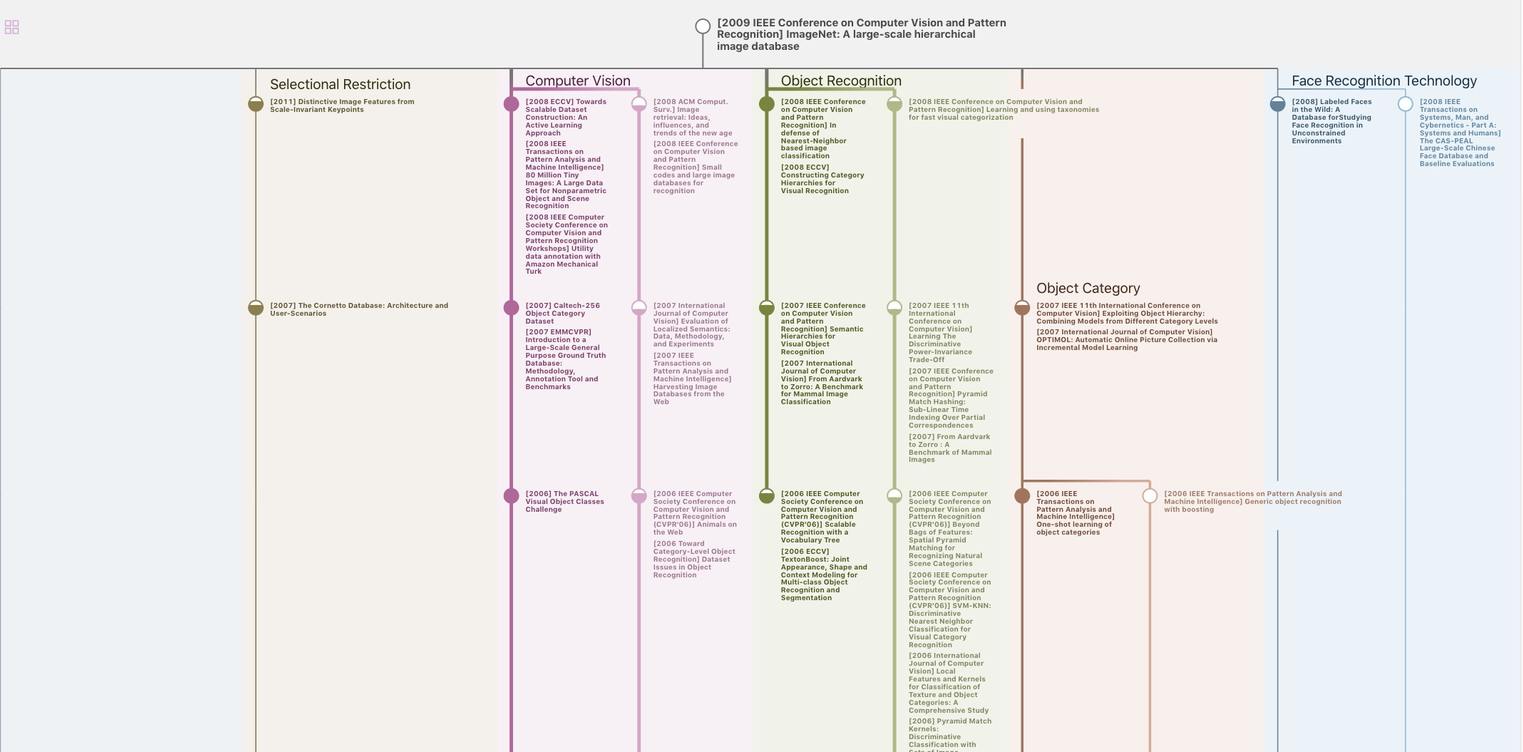
生成溯源树,研究论文发展脉络
Chat Paper
正在生成论文摘要